The program is in CEST Time, check the time converter to know your local time.
1A1-I1
The strong increase in digital computing power in combination with the availability of large amounts of data has led to a revolution in machine learning. Computers now exhibit superhuman performance in activities such as pattern recognition and board games. However, the implementation of machine learning in digital computers is intrinsically wasteful, with energy consumption becoming prohibitively high for many applications. For that reason, people have started looking at natural information processing systems, in particular the brain, that operate much more efficiently. Whereas the brain utilizes wet, soft tissue for information processing, one could in principle exploit any material and its physical properties to solve a problem. Here we give examples of how nanomaterial networks, in particular dopant network processing units (DNPUs), can be trained using the principle of material learning to take full advantage of the computational power of matter [1].
Previously, we have shown that a disordered (or ‘designless’) network of gold nanoparticles can be electronically configured into arbitrary Boolean logic gates using artificial evolution [2]. We demonstrated that this principle is generic and can be transferred to other material systems. By exploiting the nonlinearity of variable-range hopping transport in a nanoscale network of boron dopants in silicon, we can significantly facilitate classification. Using a convolutional neural network approach, it becomes possible to use our device for handwritten digit recognition [3]. An alternative material-learning is approach is followed by first mapping our Si:B network on a deep neural network model, which allows for applying standard machine-learning techniques in finding functionality [4]. Finally, we show that the widely applied machine learning technique of gradient descent can be directly applied in materio, using the principle of homodyne gradient extraction, opening up the pathway for autonomously learning hardware systems.
1A1-I2
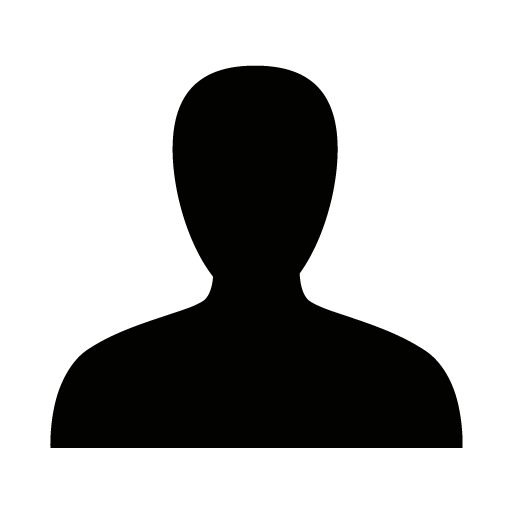
Resistive random access memory (RRAM) technologies, often referred to as memristors, hold fantastic promise for implementing novel in-memory computing systems for massively parallel, low-power and low-latency computation. Compared to conventional systems, these solutions offer promising advantages in terms of energy efficiency and computing power when processing AI workloads. This talk will first present the role of RRAM to enable the hardware implementation of resource-constrained neuromorphic hardware. I will present a event-driven object localization system that couples state-of-the-art piezoelectric micromachined ultrasound transducer (pMUT) sensors to a neuromorphic resistive memories-based computational map. Second, I will present an approach where the random nature of resistive memories, instead of being mitigated, is fully exploited to implement a type of probabilistic learning in Bayesian neural networks. The inherent variability of resistive memories can naturally implement the sampling step in the Metropolis-Hastings Markov Chain Monte Carlo algorithm. This approch constitutes a new path to bring learning to the edge.
1A1-T1
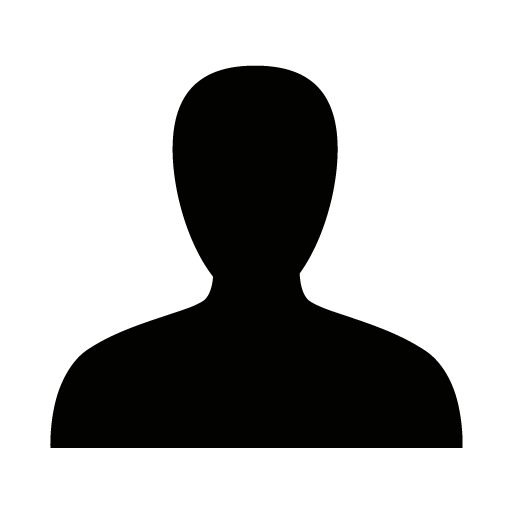
With Moore's law coming to an end and Artificial Neural Networks gaining widespread adoption, specialized neuromorphic accelerators have become highly sought after. Electrochemical conductive-polymer devices with many linearly programmable resistance states enable uncomplicated, energy-efficient implementations. They suffer, however, from self-discharge and non-ideal writes caused by depolarizing impurities that are difficult to exclude and limit device performance. This is especially valid for scaled-down devices. Predicting these effects through numerical simulations could enable partial mitigation and highlight hard device limits.
Our work combines two-phase charge transport models based on the Nernst-Planck-Poisson equations [1] with electrochemical self-discharge based on a Butler-Volmer approach to simulate PEDOT:PSS based neuromorphic devices [2, 3] in detail. These device models are aggregated into virtual neuromorphic crossbar arrays, on which inference of neural networks under the influence of self-discharge is simulated. Our device models validate against experimental data for cyclic voltammetry and self-discharge experiments. They can predict optimal device parameters and operating conditions depending on depolarizing impurities and write error. For example, for devices based on PEDOT:PSS/PEI, between 81 and 107 discrete states stable for at least five minutes can be expected at 0.5 % write error. Our results show that a single-layer network previously implemented on a metal-oxide memristive crossbar [4] can be transferred to electrochemical devices and keep a perfect classification accuracy of 100 % for over three hours at ambient conditions. Furthermore, compensatory programming pulses based only on the crossbar state and time of inference revert the drift in the network's weights entirely within the read/write accuracy limits. We expect a multi-layer network to see a more substantial impact of self-discharge that the demonstrated compensatory pulses will help help to offset.
This work enables an in-depth understanding of electrochemical neuromorphic devices, their limitations, and how to mitigate them. It, therefore, takes an essential step towards the successful implementation of the first electrochemical neuromorphic accelerators.
1A2-T1
Juan Bisquert (pHD Universitat de València, 1991) is a Professor of applied physics at Universitat Jaume I de Castelló, Spain. He is the director of the Institute of Advanced Materials at UJI. He authored 360 peer reviewed papers, and a series of books including . Physics of Solar Cells: Perovskites, Organics, and Photovoltaics Fundamentals (CRC Press). His h-index 95, and is currently a Senior Editor of the Journal of Physical Chemistry Letters. He conducts experimental and theoretical research on materials and devices for production and storage of clean energies. His main topics of interest are materials and processes in perovskite solar cells and solar fuel production. He has developed the application of measurement techniques and physical modeling of nanostructured energy devices, that relate the device operation with the elementary steps that take place at the nanoscale dimension: charge transfer, carrier transport, chemical reaction, etc., especially in the field of impedance spectroscopy, as well as general device models. He has been distinguished in the 2014-2019 list of ISI Highly Cited Researchers.
A memristor is a two-terminal device that undergoes a voltage-controlled conductance change. Because the resistance depends on the history of the system, it has a strong hysteresis effect and produces a resistance switching. Memristors are the key elements for neuronal networks, as the memory effect represents plasticity of synapses. Neurons have the same ingredients as memristors plus at least one negative resistance that destabilizes the system in a Hopf bifurcation that passes the dynamics from rest to a spiking state. The operation of spiking networks occurs by time domain impulses, but characterization of the material elements is much better done in the frequency domain, by the techniques of impedance spectroscopy. Here we provide the methods to assess memory, plasticity and spiking in the frequency domain, and we show the transformation to the time domain. We present the fundamental model of a halide perovskite memristor, that describes the behaviour both in time and frequency domain. Next, we show the impedance spectroscopy criteria for dynamical regimes of a FitzHugh-Nagumo model, that is a representative minimal model of a spiking neuron. We expand the analysis to cover the possible impedance spectroscopy behaviours of all two-dimensional oscillating systems. In conclusion we show that impedance spectroscopy is a strong characterization method for producing memristors, synapses and neurons with tailored temporal dynamics, hysteresis, and rhythmic oscillations for neuromorphic computing.
1A2-T2
Antonio Guerrero is Associate Professor in Applied Physics at the Institute of Advanced Materials (Spain). His background includes synthesis of organic and inorganic materials (PhD in Chemistry). He worked 4 years at Cambridge Dispaly Technology fabricating materiales for organic light emitting diodes and joined University Jaume I in 2010 to lead the fabrication laboratory of electronic devices. His expertise includes chemical and electrical characterization of several types of electronic devices. In the last years he has focused in solar cells, memristors, electrochemical cells and batteries.
Families of hybrid perovskite materials have been developed in the last 10 years for photovoltaics and unique properties have been revealed that render them suitable for memory applications. In particular, the discovery of their mixed electronic and ionic conductivity that links with the hysteresis in their electrical response allows the presence of different conductance states. The hybrid perovskites can be described as ABX3 where A = monovalent cations, B = divalent cations and X = halide anions. The versatility of the system is very high and it is possible to use combinations of cations (Methyl ammonium, Formamidinium, Cs+, guanidinium+ and Rb+) and anions (I, Br) to modify their physical and chemical properties. Particularly useful for memory applications, the interplay between electronic current and ionic displacements can be tuned with the formulation leading to a range of ion diffusion coefficients and hysteresis behaviours.1, 2 Here, we analyse the main properties of these families and we discuss the dynamic interaction of migrating ions with external interfaces in their role as memristor devices.3,4 We discuss the internal mechanism on the basis of the device configuration and the electrical response leading to volatile and non-volatile memories with different conductance states.
REFERENCES:
1. Li, C.; Guerrero, A.; Huettner, S.; Bisquert, J., Unravelling the role of vacancies in lead halide perovskite through electrical switching of photoluminescence. Nature Communications 2018, 9 (1), 5113.
2. Wang, H.; Guerrero, A.; Bou, A.; Al-Mayouf, A. M.; Bisquert, J., Kinetic and material properties of interfaces governing slow response and long timescale phenomena in perovskite solar cells. Energy Environ. Sci. 2019, 12 (7), 2054-2079.
3. Solanki, A.; Guerrero, A.; Zhang, Q.; Bisquert, J.; Sum, T. C., Interfacial Mechanism for Efficient Resistive Switching in Ruddlesden–Popper Perovskites for Non-volatile Memories. J. Phys. Chem. Lett. 2020, 11 (2), 463-470.
4. Gonzales, C.; Guerrero, A.; Bisquert, J., Spectral properties of the dynamic state transition in metal halide perovskite-based memristor exhibiting negative capacitance. Appl. Phys. Lett. 2021, 118 (7), 073501.
1A2-T3
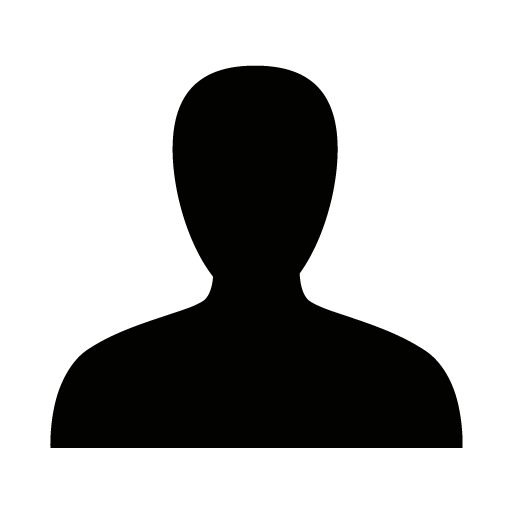
Brain-like architectures for future computing are touted as the next standard for processing information [1]. These neuromorphic architectures can provide a more efficient platform than traditional technologies for specific tasks. Nanoscale networks of such standard technologies have been investigated with respect to nanowire networks or nanoparticle systems [2], [3]. Such networks develop due to the formation of percolating networks inside tunnel junctions which emulate a biological neuron [2]. But comparable efforts have not been performed on technologies that can be scaled up to large area systems. In this work, we demonstrate a scalable 2D material system comprised of CVD-grown hBN on Cu contacted with Ag. Such a structure gives rise to atomic-scale networks due to the diffusion of Ag inside the hBN matrix which also acts as a resistive switching memory device. The formation of nanoscale networks establishes brain-like network dynamics which show critical avalanches as those observed in cortical tissue structures [4]. We observe the avalanche behavior in both low resistance (LRS), as well as high resistance (HRS) states corroborating the role of Ag diffusion in the formation of nanoscale networks leading to change in channel resistance. Reports have suggested that systems with nanoscale networks exhibit critical behavior in a self-organized manner where the distribution function of quantized conductance (∆G) and inter-event intervals (IEI) follow power laws. We observe power-law fits for ∆G and IEI with exponents of the order of 2.45 and 1.54 respectively. Such a range of exponents has been previously observed in neuronal avalanches [4]. The avalanche behavior observed in the Ag-hBN system also exhibits scale-free dynamics as they are autocorrelated and demonstrate spatial and temporal long-range correlation. Thus, we report the first of its kind brain-like avalanche behavior in a 2D material system comprising of Ag-hBN, this kind of system can be scaled up to form large-area devices and the case of hBN being a 2D material allows for engineered structures for future applications in neuromorphic computing.
1A2-T4
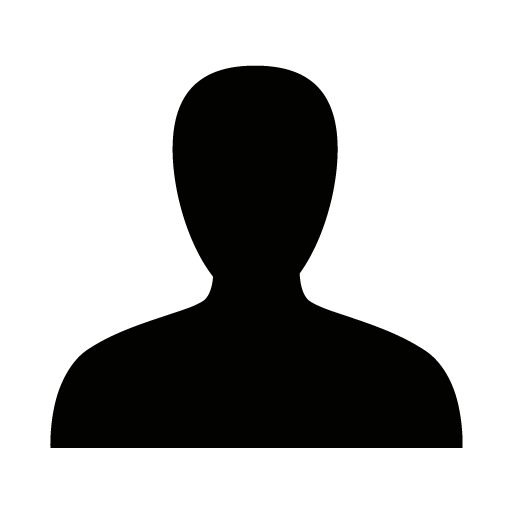
Designing materials and devices for energy-efficient hardware is a crucial aspect for developing novel applications and for computing schemes beyond von Neumann. Devices on complex oxide platforms, such as SrTiO3 (STO), bring in functionalities that are relevant for this purpose. These functionalities are largely derived from the temperature and electric field dependence of its dielectric permittivity. As a result, such devices possess transport characteristics that can be controllably changed by external stimuli such as temperature, voltage, current, electric and magnetic fields, and spin-orbit torque. In this context, we have exploited the rich phase space, intrinsic to complex oxides, to build devices capable of brain-like physical computing. We will discuss two memristive device types, where tailoring of the energy landscape enables multistate resistance states.
The first work is based on interface based memristive Schottky devices on semiconducting Nb-doped STO (Nb:STO). These memristors require no forming process and show continuous conductance modulation akin to synapses [1]; we have previously shown that this behaviour can be described by a power-law which can successfully be implemented as a learning algorithm [2]. Given that these memristors meet both hardware and software requirements, they serve as a strong material candidate for material-based computing. The scalability of memristors is an important consideration for integration into novel architectures. For example, limitations in device stability, endurance and associated enhanced power of operation when devices are miniaturised are major roadblocks in the successful implementation of filamentary memristors in large scale architectures. In this work, we developed a reliable process to fabricate Co contacts on Nb:STO of variable device dimensions and performed electrical measurements on devices of areas spanning three orders of magnitude. We show the ability to achieve analogue switching with remarkably high resistive windows by downscaling, high endurance and low device and cycle variation integrated directly on a semiconducting platform. We were able to verify the results by measuring across a range of devices showing minimal device-to-device variation. By combining an ionic semiconductor possessing unconventional properties with an appropriate device design, local enhancement of the electric field around the devices’ edges tunes the dielectric constant and increases the concentration of defects leading to improved resistive switching.
In the second type of devices, we utilise the crystal orientation and show that magnetic anisotropy in tailored SrRuO3 (SRO) ferromagnetic layers can be tuned to either exhibit a perfect or slightly tilted perpendicular magnetic anisotropy (PMA). We show that the strong magnetocrystalline anisotropy in SRO not only allows for the design of a perpendicular magnetic anisotropy in such devices but enables the tailoring of easy axes at controlled tilt angles from the surface normal, for probabilistic as well as deterministic switching, with relative ease [3].
1A3-I1
Prof. Jennifer Rupp is the Thomas Lord Associate Professor of Electrochemical Materials at the Department of Materials Science and Engineering, and Associate Professor at the Department of Electrical Engineering and Computer Science at MIT. Prior she was a non-tenure track assistant professor at ETH Zurich Switzerland where she held two prestigious externally funded career grants, namely an ERC Starting Grant (SNSF) and Swiss National Science Foundation (SNF) professorship.
She previously was affiliated as a visiting and senior scientist at MIT (2012-2011), the National Institute of Materials Science (NIMS) in Tsukuba, Japan (2011), and was working as a postdoc at ETH Zurich (2010-2006). Rupp team`s current research interests are on processing of ceramic and glass materials, solid state material design and tuning of structure-property relations for novel energy and information devices and operation schemes. This ranges from alternative energy storage via solid state batteries, solar-to-synthetic fuel conversion or novel types of neuromorphic memories and computing logic entities for data storage and transfer beyond transistors and new sensing functions to track chemicals in the environment. Here, her team goes the whole way from material design, novel processing techniques to make ceramics, cermets or glassy-type ceramic structures up to novel device prototypes, their operation and characteristics.
She has published 90 papers, holds 12 patents, and, being a frequent speaker and panel member of the World Economic Forum, enjoys discussing material tech trends on the theme of energy with the public, economists and policy makers. Rupp also enjoys engaging with companies all around the world through both consultancy and collaborations focused either on material processing business or electrochemical device & product engineering (e.g. battery, oil & fuel processing, sensing, electronic companies). Currently, she holds a position as associate editor at Journal of Materials Chemistry A and is also on the advisory board member for Advanced Functional Materials and Advanced Materials Interfaces.
Rupp and team received several honors and awards such as the Displaying Future Award by the company Merck KGaA 2018 for a glucose converting fuel cell chip, BASF and Volkswagen Science Award 2017 for battery research, "Top 40 international scientist under the age of 40" by World Economic Forum 2015, Spark Award for the most innovative and economically important invention of the year 2014 at ETH Zurich, Kepler award “new materials in energy technology” by the European Academy of Science 2012 or Young Scientist Award by the Solid State Ionic Society. She gave keynote lectures at Royal Society UK 2018, Nature Energy conference 2016, Gordon Research lecture 2014 and many others, also she presented on battery and energy technologies at the World Economic Forum 2017.
Next generation of energy storage may largely benefit from fast Li+ ceramic electrolyte conductors to allow for safe and efficient batteries. With recent discoveries in thin film processing solid-state lithium ion conductors, such as Li-garnets and LIPON or LiSICON-based solids, have been recently considered as candidate materials not only for next-generation solid-state batteries but also for neuromorphic computing via memristors owing to the fast ionic transport in the solid-state electrolyte.
In the first part of this talk, we review current needs and challenges in the field of solid state batteries. We underline the advantages of various Li solid-state conductor materials and reflect on opportunities of thin film processing, being a requirement to define precisely the Lithium stoichiometries and related electronic state changes for transition metal ions, miniaturize the device, and reach high energy/information densities for energy storage, computation, and sensing.
In the second part, we focus on Li-film processing and controlling Lithium stoichiometries to reach fast conductive phases for Li garnets and Li titanates as solid state battery components, and memristive neuromorphic computing units. Insights on structure-phase-transport interaction and implications on performances will be exemplified for energy storage aiming high energy densities, and modulations of synaptic artificial weights through lithium induced metal-to-insulator transitions in lithium titanate memristors.
1A3-T1
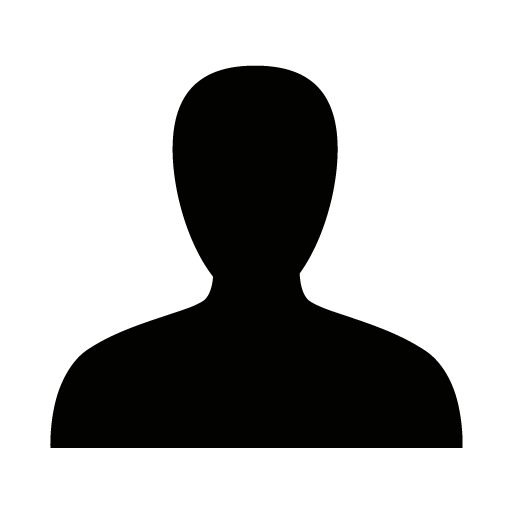
In Mott insulators electrical currents can change resistance by orders-of-magnitude due to an insulator-metal phase transition. The volatility of switching in Mott insulators can be tuned, enabling both memory devices and neuron-like functionalities. In this talk I will present our recent advances in defect- and strain- engineering of Mott insulators, with the aim of developing highly energy-efficient resistive switching devices and novel functionalities.
I will first discuss how defects induced by ion irradiation in V2O3 and VO2 nanowire devices can dramatically reduce the electric field and energy required for switching by inducing non-thermal insulator-metal transitions. I will then discuss strain engineering in V2O3 which allows to tune the trajectory of the phase transition, allowing access to hitherto inaccessible regions of the phase diagram. We first report on a thermally-induced transition into the paramagnetic insulating phase in pure V2O3 which to date has only been observed in Cr-doped V2O3 samples. This may allow devices operating above room temperature to harness this prototypical Mott transition. Next, we show how to modify the trajectory of the phase transition so that it follows the metal-insulator equilibrium line down to absolute zero using self-induced stress. These unusual states of matter hold great promise for resistive switching applications, in terms of both energy efficiency and functionality.
1A3-T2
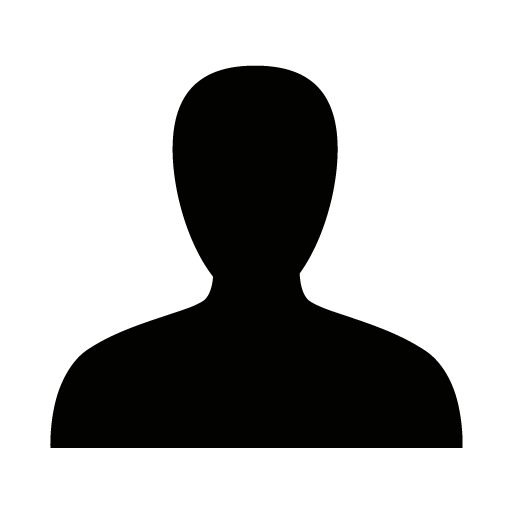
Within the last few years, memristors have aroused much interest in the scientific community.[1] Their resistance tunability, as well as their hysteretic and non-volatile behaviour has made them attractive for memory applications. Recently, memristors are actively investigated as hardware implementations for future neuromorphic computers. This emerging field comprises novel electronic circuits inspired by the human brain, motivated by its strikingly low energy consumption, high efficiency of cognitive tasks, such as image or pattern recognition, and the ability of the brain to reconfigure nervous connections, also called neuronal plasticity.[2] Prominent candidates for enabling neuromorphic computing on a hardware-level are ferroic materials. An intrinsic feature of all ferroic materials is the occurrence of domain walls (DWs) separating domains, the latter being regions with homogeneous orientation of the order parameter (electric polarization in the case of ferroelectrics). Ferroelectrics have already been successfully employed for memristive devices such as ferroelectric tunnel junctions.[3] At the same time, ferroic materials paved the way for the large class of DW-based nanodevices, which can exhibit high reconfigurability as DWs can be easily written and erased.[4] Extensive works on the multiferroic oxide bismuth ferrite (BiFeO3) have shown that DWs in thin films can be more conducting than the domains.[5] During thin film growth, BiFeO3 self-assembles into a nanoscale network of conducting domain walls,[6] which can be tailored by varying the deposition parameters. While the memristive properties of the individual DWs have been demonstrated,[7] their functionality as building blocks for hardware-based neural networks is currently being investigated.[8] However, control of the nanoscale connectivity of the DW network, as well as characterizing the electrical conductivity through the network, has proven to be very challenging. Here we present our progress on the study of the transport properties of self-assembled DW networks in BiFeO3 thin films. Scanning probe techniques allow local DW probing to investigate the memristive properties and mapping of the conductive DW network.
2A-I1
Fredrik Sandin is a professor in Machine Learning at the Lulea University of Technology (LTU) in Sweden. He is interested in brain-inspired machine learning, neuromorphic computing, and applications requiring new efficient approaches to sensing, computing, and artificial intelligence. He was Gunnar Öquist Fellow (2014) and received two post-doctoral scholarships, one in brain-inspired computing from LTU (2010–11) and one in theoretical physics from FNRS in Belgium (2008–09). He has a Ph.D. in Physics (2007) from the Swedish Graduate School of Space Technology and received the 2004 “New-Talents” award for original work in theoretical physics at the International School of Subnuclear Physics in Erice.
Mixed-signal neuromorphic processors with dynamic neurosynaptic elements and sparse, event-based information coding are used for ultra-low-power inference and learning. In such processors, the dynamic properties of the neurosynaptic elements are inhomogeneous due to device mismatch, i.e., the dissimilarity between small-scale integrated circuit (IC) components. We describe how the resulting inhomogeneous neurosynaptic dynamics have been exploited for resource-efficient spatiotemporal inference with single neurons and spiking neural networks. For example, we demonstrate: (i) how inhibitory–excitatory pairs of dynamic synapses can be used to mimic non-spiking post inhibitory rebound in an auditory feature detection circuit of crickets, and (ii) how spatiotemporal receptive fields of output-neurons in the Spatiotemporal Correlator (STC) network can be implemented using balanced disynaptic inputs instead of dedicated axonal or neuronal delays. In experiments with the DYNAP-SE neuromorphic processor, we demonstrate synapse level temporal feature tuning with a configurable timescale of up to 0.1 seconds. This approach leads to about one order of magnitude reduction of the energy dissipation per lateral connection in an STC-type network with disynaptic dynamics instead of dedicated delay neurons. We discuss some desired properties of neuromorphic devices and materials in a regime where device mismatch constitutes the basis for efficient inference and reproducible learning.
2A-I2
Yao-Hong Liu is currently Scientific Director in imec. He is a recipient of a European Research Council (ERC) Consolidator grant and is the principal investigator of “Intranet of Neurons.” His research focus is high-capacity and minimally-invasive wireless technology for implantable brain-computer interfaces.
Dr. Liu received his Ph.D. degree from National Taiwan University, Taiwan, in 2009. He was with Terax, Via Telecom (now Intel), and Mobile Devices, Taiwan, from 2002 to 2010, developing Bluetooth, WiFi and cellular wireless SoC products. Since 2010, he joined imec, the Netherlands, and is leading the research of the ultra-low power wireless ASIC design for IoT and implantable applications. He served as a technical program committee of IEEE ISSCC, and currently serves in IEE RFIC symposium. He was a recipient of ISSCC 2020 Best System Demonstration Award.
“Sitting on your shoulders is the most complicated object in the known universe” - Prof. M. Kaku. The human brain has up to 80 billion of neurons, which is similar to the number of planets in our Galaxy. It gives us a feeling that we are an independent and unique individual, but we have very little idea how the brain functions. Many neural sensing technologies, mostly imaging, have been developed for scientific or therapeutic purposes. With the advancement in semiconductor technology, silicon-based intra-cortical micro-electrode arrays (MEAs) enable electrical sensing with sub-millisecond temporal resolution and 10’s of µm spatial resolution. They have become the most widely adopted method in neurotherapeutics or neuroscience experiments at the moment. However, there are still many challenges yet to be overcome. For instance, high channel count of neural sensing with high temporal resolution produces a huge amount of data, and they need to be wirelessly transferred across tissues without introducing heating. However, such brain implants should be highly miniature to minimize the surgical invasiveness, so they have very limited resource to support energy-consuming wireless transmission. In this plenary talk, we will discuss how neuromorphic technology inspired from our brains can be a potential solution for future brain-computer interfaces.
2A-I3
After graduating in physics from University of Cologne, Germany he obtained a Ph.D. in semiconductor physics at the IHP institute (Innovations for High Performance Microelectronics) in Frankfurt-Oder, Germany in 1997. Afterwards taking a post-doc position at University of California, Berkeley, US, working on the characterization of GaN thin films for device applications.
In 1998 he joined Siemens Semiconductor, now Infineon, working on CMOS technology development from 0.25µm down to 40nm and beyond with the focus on embedded memories for automotive microcontroller, smartcard and IoT applications. With the Qimonda memory spin off he worked on 4x ... 3x nm multilevel NAND Flash technology for SSD applications. In the recent years he followed the various emerging memory concepts like PCRAM, RRAM or MRAM, again for embedded microcontrollers.
Since 2019, with the start of the new development center in Dresden, he focused on research of hardware concepts for AI acceleration. New concepts like analogue or spiking neuromorphic are key for a power efficient and secure edge-AI. Currently he is leading a group working on spiking neural network algorithms and hardware for radar processing in automotive.
Driven by mobility and internet-of-things, edge-AI has become increasingly important. Whether it is home applications, autonomous driving or fields like sensor networks and drones, all benefit from local AI data processing close to the device or sensor.
Thus, largely reducing energy consumption avoiding high-bandwidth data transport. Autonomous driving or drones doesn’t even allow communication with bigger processing infrastructure due to real-time latency requirements.
In areas where personal data like audio, visual or even medical data is processed, it is a requirement for privacy and security to handle the data as much as possible local on the device.
However, todays AI algorithms require intensive data processing limiting the scope of edge-AI applications. Although impressive progress has been reached by technology scaling to run GPU, NPU hardware accelerators also on mobile devices, still the power consumption remains a problem to be solved. For level 5 autonomous robot taxis the overall data processing would consume appr. 30% of the battery range of today’s electric cars [1].
And even such edge-AI is still focused on “static” classification of data, e.g. images or “signatures over time”. In contrast, AI processing of time-series sensor data provides further challenges. Prediction of movement of objects in radar requires history to identify only the relevant echoes (KI-ASIC) Systems depending on complex chemical and physical properties, like batteries [2], drones or car electric drives [3], need to include them in the control & prediction loop.
Recurrent neural networks (RNN) or long-short term memory (LSTM) neural networks are applied [4] but have strong disadvantages in multiply and accumulate acceleration due to complex neuron structure of input, forget and output gates as well as limited data re-use [5]. Neuromorphic, spiking neural networks (SNN) are much more hardware friendly working in the digital domain [6], but still lacking good supervised training algorithms. Here new concepts are available like surrogate gradients [7] to allow for backpropagation training.
For a radar gesture recognition, it is shown that such training can be done with backpropagation through time. Efficiently small networks with only 10...30 hidden leaky integrate-and-fire neurons are on-par or even able to outperform LSTM networks. The network is implemented on a digital simulation SpiNNaker 2 FPGA prototype in a real time closed loop system. Impact of quantization and buffer memory usage is investigated to optimize power and latency.
However, still it is an ongoing topic of research what applications and network architectures are best suited for SNN. Obviously, a sparse spike generation helps to reduce power and memory consumption [8]. Several approaches on spike encoding and neuron function are investigated for the gesture recognition, trading spike rate vs. accuracy [9]. For some layers, like the input layers as bitmaps, it is even beneficial to stay with e.g. CNN layers. Resulting in a hybrid CNN-SNN model still trained end-to-end.
All this learning contributes to a benchmarking of such neuromorphic approaches [10, 11]. Nevertheless, it still remains a hard problem due to large variation of use cases, platforms and training, pointing to competitions as best comparison [12].
2A-T1
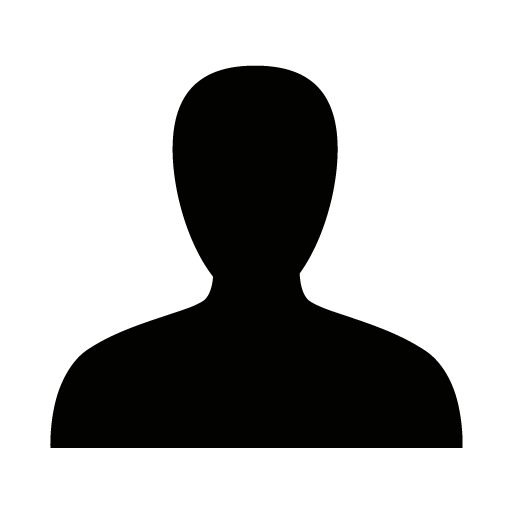
Sequential learning, the training and correct prediction of recurring sequences of different events, is one of the core capabilities of biological brains and has many potential technological applications, e.g., in natural language processing or anomaly detection. Here we focus on a concept for sequence learning inspired by the Spiking Temporal Memory (Spiking TM) algorithm [1]. This algorithm allows for continuous learning in a sparsely active network, which promises energy efficiency and makes it favorable for edge IoT applications. To overcome the shortfalls and limitations of an implementation of this algorithm on conventional von-Neumann machines, in this work we propose mapping of this algorithm on a CMOS-co-integrated resistive switching ReRAM crossbar array. ReRAM devices are two terminal resistive switching devices with voltage-history dependent resistance and are considered low power and low area realizations for weights in neural networks.
In a first step, we illustrate the biologically inspired core principles of the spiking TM algorithm including prediction, inhibition, and firing-rate homeostasis and explain how they are adapted in a spiking array-wide learning rule. This Hebbian-type learning rule is facilitated solely by the neuron circuitry outside of the ReRAM crossbar array. Therefore, weights can be realized by a simple one-transistor-one-ReRAM-device (1T1R) structure. As the number of weights scales quadratically with the number of neurons, this structure is beneficial for the scaling behavior of the architecture. It is important to point out that the spatial and temporal locality of the Spiking TM algorithm can nevertheless be conserved in the proposed learning rule.
Circuit-level simulations of the array and its periphery are conducted employing the physics-based JART VCM v1b model [2]. After illustrating the training procedure, we show how the system successfully learns context sensitivity, e.g., to discriminate between different sequences which share a subset of sequence elements (high-order sequences) and investigate the energy consumption during training.
2B-T1
Lyes Khacef is a PostDoc in the Bio-Inspired Circuits and Systems group at University of Groningen. He received the M.Sc. in electrical engineering and embedded systems from the University of Nice Sophia Antipolis (France). He pursued a PhD at Université Côte d’Azur (France) in brain-inspired computing where he worked on understanding and modeling the local computations behind the self-organization capabilities of the brain. He proposed a new model for multimodal unsupervised learning based on local structural and synaptic plasticity rules, implemented then on FPGA-based cellular neuromorphic hardware. Dr. Lyes Khacef developed a multi-disciplinary expertise in computational neuroscience, machine learning and hardware design. He is currently working on the modeling and hardware prototyping of spike-based synaptic plasticity rules, for learning spatio-temporal patterns using neuromorphic vision, audio and tactile sensors.
With the expansion of edge devices in our daily lives, the need for efficient and adaptive embedded systems is growing fast. However, the current Von Neumann architectures and AI models reach their technical and intellectual limits, and fail to address the current challenges of embedded intelligent systems. This is why we follow a brain-inspired computing approach based on the co-development of neuromorphic hardware and algorithms [1]. In particular, we focus on the local mechanisms of structural and synaptic plasticity that lead to the emergence of the global structure and function of the brain. Locality in synaptic plasticity means that all the information needed for the synapse update is locally available in time and space, i.e. based on the activity of the pre- and post-synaptic neurons that the synapse connects [2]. In particular, when implemented in parallel and distributed neuromorphic hardware, locality in time satisfies the real-time constraint of online learning and diminishes the memory overhead, while locality in space satisfies the energy-efficiency constraint of on-chip learning. In addition, local synaptic plasticity rules are inherently unsupervised, opening the possibility of continual adaptation. Nevertheless, the main drawback of local unsupervised learning is the limited performance on complex pattern recognition problems, because there is no explicit optimization of a global objective function as in gradient back-propagation. Therefore, we propose multimodal association as a biologically plausible and practical solution to improve the self-organizing system performance since, in contrast with labeled data, multiple sensory modalities (e.g. sight, sound, touch) are freely available in the real-world environment [3].
2B-I1
Neuromorphic Perception (and Computation) will induce a paradigm shift in robotics, based on the biologically inspired emerging concept of event-driven sensing and processing that leads to better robots able to acquire, transmit and process information only when needed, optimising the use of resources, leading to real-time, low-cost, operation.
In this talk, I will describe our approach to implement neuromorphic tactile sensing. The sparseness of tactile input over space and time calls for ED encoding, where the sensors are not continuously sampled, rather wake-up at stimulation. I'll describe how we emulate the firing properties of biological tactile afferents. I will then describe different flavours of approaches to extract information from neuromorphic vision sensors that can be useful in robotics. We will span from low-latency and real-time computer vision tasks to biologically inspired architectures. The neuromorphic approach will greatly enhance computer vision for robots that have to interact with objects and people in real time, adapting to sudden changes, failures and uncertainties.
2B-I2
Giacomo Indiveri is Professor of Neuromorphic Cognitive Systems at the University of Zurich and ETH Zurich. He holds an MSc degree in electrical engineering and a PhD degree in computer science. His interests lie in the design of mixed signal neuromorphic electronic systems and learning circuits.
Artificial Intelligence (AI) and deep learning algorithms have demonstrated impressive results in a wide range of applications. However, they still have serious shortcomings for use cases that require real-time processing of sensory data and closed-loop interactions with the real-world, in uncontrolled environments.
Neuromorphic Intelligence (NI) aims to mitigate this shortcoming by developing ultra-low power electronic circuits and radically different brain-inspired in-memory computing architectures.
In this presentation I will present examples of NI circuits that exploit the physics of their devices to directly emulate the biophysics of real neurons, and I will demonstrate applications of NI processing systems to use cases that require low power, local processing of the sensed data, and that cannot afford to connect to the cloud for running AI algorithms.