Session-K1
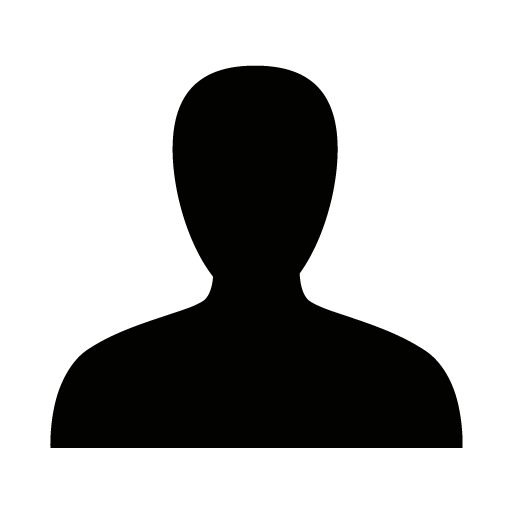
Session-K2
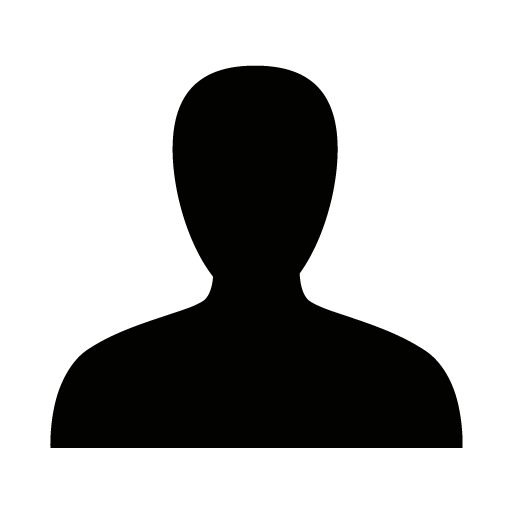
Session-K3
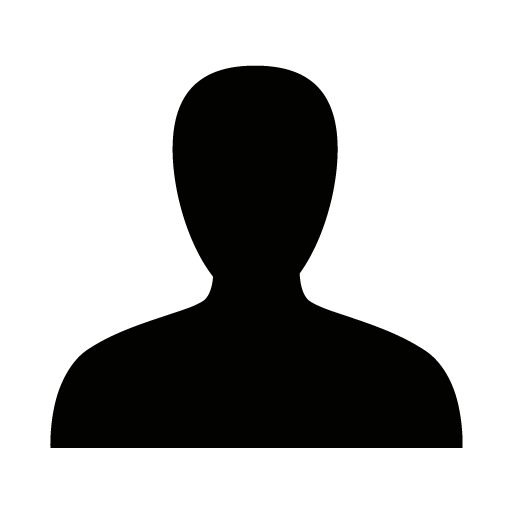
Session-K4
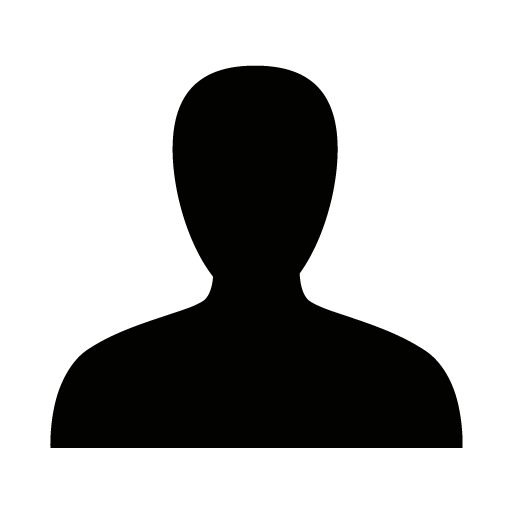
1.1-I1
J. Joshua Yang is a professor of the Department of Electrical and Computer Engineering at the University of Southern California. He was a professor of the ECE department at the University of Massachusetts Amherst between 2015 and 2020. His current research interest is Post-CMOS hardware for neuromorphic computing, machine learning and artificial intelligence, where he published several pioneering papers and holds 120 granted and about 60 pending US Patents. He is the Founding Chair of the IEEE Neuromorphic Computing Technical Committee, a recipient of the Powell Faculty Research Award and a recipient of UMass distinguished faculty lecturer and UMass Chancellor's Medal. He serves on the Advisory Boards of a number of prime international journals and conferences, including serving as an associate editor of Science Advances. Dr. Yang is a Clarivate™ Highly Cited Researcher in the field of Cross-Field and an IEEE fellow for his contributions to resistive switching materials and devices for nonvolatile memory and neuromorphic computing.
Memristors, emerging as key enablers of next-generation computing, can be broadly categorized into diffusive and drift types based on their reset mechanisms. Diffusive memristors reset via the spontaneous movement of mobile species under zero bias, exhibiting stochastic dynamics that closely resemble biological ion transport, making them highly suitable for neuromorphic computing. In contrast, drift memristors rely on electric-field-driven ion migration, allowing precise control over non-volatile resistance states, which is ideal for analog neural network implementations.
This talk will present recent progress in memristor device design, array-level integration, and system-level demonstrations, highlighting their transformative potential in neuromorphic and analog computing architectures. Special emphasis will be placed on the role of material engineering, particularly in selecting materials with appropriate ion migration activation energies to optimize memristor performance. Additionally, emerging in-memory computing applications in scientific computing and edge AI hardware will be discussed to showcase the broad relevance of this technology.
1.1-I2
Artificial intelligence is pushing the limits of digital computing to such an extent that, if current trends continue, global energy consumption from computation alone would eclipse all other forms of energy in the next two decades [1]. Consequently, it is crucial to explore new strategies that can reduce energy consumption and increase computational speed to meet growing demands. One promising approach is in-memory analog computing, which has the potential to deliver significant improvements in both efficiency and speed. However, designing analog computing systems presents considerable challenges. First, analog computing necessitates a fundamental rethinking of computation at the material level, where information is now stored as continuously variable physical observables. This shift introduces difficulties related to the accuracy, dynamic range, and reliability of analog systems—issues that prompted the transition to digital computing nearly a century ago. Second, digital computation has traditionally relied on a reductionist hierarchy, allowing for the separate design of devices, architectures, and algorithms without sensitivity to one another. In contrast, analog computing systems are inherently interdependent from the material to architectural level, and this sensitivity can be advantageous. I will discuss two approaches to in-memory compute: first, on developing accelerators for neural networks using analog memory, and second, on developing dynamic devices which more closely mimic the brain. In the case of memory devices, I will focus on newly developed electro-thermo-chemical random-access memory (ETCRAM) [2]. ETCRAM exhibits a dynamic range of more than six decades of analog tunable resistance with thousands of available states, deterministic write operations with high accuracy, low programming voltages, current-voltage linearity across all decades, and programming speeds as fast as 15 ns. These features, when combined in a single device, heralds a massively improved set of benchmarks for analog compute. Second, I will discuss our work on designing artificial axons, neurons, and networks using spin-crossover oxides[3,4].
1.1-I3
The world today, dominated by data-centric applications, require local data processing or computing at the edge, known as edge intelligence, to decide on a course of action autonomously, without communicating data to cloud platforms. So far, Moore’s Law scaling of semiconductor devices provided the needed hardware support for the heavy workload of AI computing. However, with the end of Moore’s law scaling and limitations of von Neumann computing architectures, the performance and energy efficiency of conventional hardware for AI computations are facing a severe roadblock. In the first part of my talk, I will discuss the promising new discoveries of advanced CMOS-compatible HfO2-based ferroelectric devices and the electronics based on ferroelectric building blocks integrated on advanced CMOS technology nodes that can provide the needed support to the AI computing tasks, for instance in in-memory computing in data-flow architectures that can enable more than 1000X energy-efficient AI accelerators needed for edge intelligence. Smart edge intelligent IoT devices can enable new applications, for example, autonomous vehicles, robotics, industrial automation, 24x7 health monitoring, space and quantum technologies, that demand higher performance to support local embedded intelligence, real-time learning, and decision making. The field has the potential to drive the next phase of growth in the semiconductor industry.
In the second part of my talk, I will discuss an example application of brain-inspired neuromorphic hardware in autonomous cars. Autonomous vehicles rely heavily on accurate multi-sensor fusion to perceive their environment and make driving decisions. However, conventional AI-based perception systems face challenges in irregular conditions such as poor visibility, obstructions, or adverse weather conditions which can lead to incomplete information to the central computing and navigation system severely impacting the perception accuracy, threatening vehicle and pedestrian safety. In a recent work we have developed a memristor-based associative learning circuit that enhances fault tolerance by dynamically adapting to multi-sensor inputs, including camera, LiDAR, radar, and ultrasonic sensors. The proposed circuit dynamically reinforces patterns, allowing the system to retain decision-making capabilities even when certain sensors fail or provide incomplete data. The system demonstrates an average error of 6.98% across ten critical driving scenarios, with a power consumption of ~152 mW per scenario, confirming its robustness, energy-efficiency and adaptability in case of sensor failures and under-performance. I will discuss how this kind of learning circuit can act as a scalable, neuromorphic alternative for real-time adaptive decision-making in autonomous vehicles, bridging the gap between traditional digital computation and biologically inspired learning mechanisms.
1.2-I1
The electronic information devices around us have achieved remarkable development thanks to the technological progress of electronic devices, especially semiconductor devices. However, there are concerns about the performance limits of semiconductor devices, which have been supported by the incredible progress of ultra-fine processing technology. Therefore, in order to overcome the performance limits of conventional semiconductor devices, emphasis is also being placed on the creation of new concept devices with diverse functions and performance, such as brain-inspired devices. In creating such new devices, attention is being focused not only on conventional electronics technology, but also on the fusion of electronics and ionics. We have developed ionic nanoarchitectonics methods that fuse ionics, electronics and nanotechnology[1-4], and have been working on the creation of new devices with various unique functions and performances. We have already developed several devices with diverse functions and excellent performance that improve conventional electronic devices using ionic nanoarchitectonics. These include atomic switches, reservoir devices, decision making devices, artificial synaptic devices, solid-state double-layer electric transistors, on-demand multifunctional devices, and magnetisation and magnetoresistance modulation devices. In particular, the ionic nanoarchitectonics method has found to be a promising method for creating artificial intelligence (AI) devices based on neuro-morphological features, which have attracted attention in recent years. This presentation will focus on the fabrication of neuromorphic devices using the ionic nanoarchitectonics method.
1.2-O1
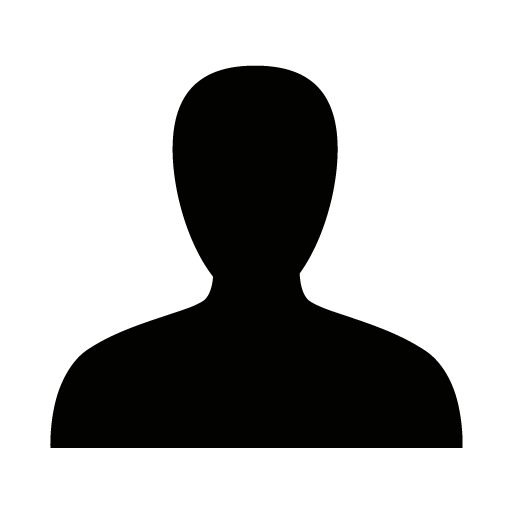
Neuromorphic devices, inspired by the brain's ability to process and store information efficiently, have garnered significant attention due to their potential for low-power computing and artificial intelligence applications. Conventional von Neumann architectures face limitations in energy efficiency and parallel processing, making neuromorphic systems a promising alternative for next-generation computing. By emulating synaptic behavior, these devices can enable advanced pattern recognition, learning, and memory functions while significantly reducing energy consumption.
In this study, we present an electrolyte-gated organic field-effect transistor (EGOFET) that utilizes a solid-state polymer electrolyte (SPE) as the gating medium. The SPE is composed of a thiol–ene-assisted photo-cross-linked nitrile butadiene rubber (NBR) network embedded with lithium bis(trifluoromethanesulfonyl)imide (LiTFSI). This photocurable polymer electrolyte can be precisely patterned through photolithography, allowing for the fabrication of devices with tailored dimensions. The resulting NBR/LiTFSI-based EGOFET demonstrates outstanding electronic performance, including a high transconductance of 11.9 mS and an impressive on/off ratio of 10⁵ at a scan rate of 40 mV/s.
Moreover, the strong polarity of the photo-cross-linked NBR network, combined with Li-ion diffusion, induces substantial current hysteresis, enabling synaptic-like plasticity and memory functions. This behavior allows the device to replicate key neuromorphic characteristics, making it suitable for artificial synapse applications. Notably, our device achieves a 91.9% recognition accuracy in deep neural network (DNN)-based handwritten digit classification. These findings highlight the potential of solid-state NBR/LiTFSI EGOFETs as energy-efficient and high-performance neuromorphic computing platforms.
1.2-O2
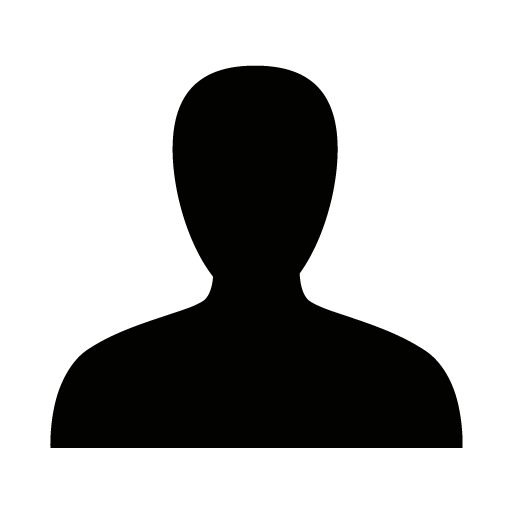
Research efforts towards novel and more energy efficient computing architectures have recently increased in order to help alleviate the high computational demands imposed by Artificial Intelligence (AI). One of the proposed solutions is to mimic the brain using neuromorphic devices, such as artificial neurons, that have potential to execute AI tasks more efficiently. Recently, artificial neurons based on electrothermally-induced insulator metal transitions (IMT) have been demonstrated to mimic neuron-like behaviors, but typically rely upon first order phase transitions[1]. On the other hand, LaCoO3 (LCO) has recently been demonstrated with neuron-like firing and axon-like signal transmission[2,3], and is based upon a second order phase transition associated with a spin crossover at technologically relevant temperatures[4–6]. We constructed LCO artificial neurons and used a combination of Raman spectroscopy, electronic and thermal measurements to study the nature of neuronal spiking. Surprisingly, many signatures observed in first order materials such as VO2 exist, despite the fundamental difference in the nature of the transition. For example, we present images of current collapse and evidence of filament formation by electrical triggering which has also been observed in first order phase transition materials. These observables are coupled to a rich electronic behavior as a function of applied bias, including self-oscillating and static regimes with volatile and non-volatile effects observed. Non-volatile effects are hypothesized to be related to strain and defects. Using a material-based approach, and a new way to directly visualize local properties concurrently to device behavior, we address the controversial spin and electronic transitions and explore how the electrothermally driven transition differs from the conventional thermally driven case. Our results suggest that abrupt gradients in temperature and strain may determine the macroscopic characteristics of devices as well as define the spatial probability of filament formation, which could be exploited for memory applications[7]. Finite element modeling of the current collapse yields similarly sized filaments and validates the universality of the phenomenon between first order and second order transitions. Our results can be applied to a broader range of materials exhibiting IMT and spin-crossover and provides new tools to explore promising neuromorphic candidates. Broadly, we demonstrate that developing device physics models is crucial to realizing neuromorphic computing architectures where device properties must be engineered from the materials level.
1.2-O3
Yanbo Xie, who now work at Institute of Extreme Mechanics, Northwestern Polytechnical University (NPU), Xi’an, China, as tenured full professor. He gained Bachelor degree of Science from NPU in 2006, and Master degree of Engineering from Peking University in 2009, and PhD degree of Engineering from University of Twente in 2013. He was selected as the Outstanding Self-financed Students Abroad in Netherlands in 2013, and awarded the Young talents award in 2015. He was appointed as the Young editor position of <Acta Mechanica Sinica> since 2021. His current research interests now focus on the fundamental studies of flows and mass transport in nanofluidic system, including the experiments, simulations and theories in flow and transport phenomena in Angstrom-scale confinement and fluidic memristors for neuromorphic computing.
The emergence of nanofluidic memristors offers a promising platform for mimicking biological synapses, where signal transmission relies on ionic transport in aqueous environments [1,2,3]. In our previous studies, we demonstrated the realization of nanofluidic memristors through various mechanisms, including ion-wall interactions in conical nanochannels using ionic liquids [1], voltage-gated mechanical deformation of polymer films [4], dynamic ion enrichment and depletion in asymmetrically charged channels [5] in 2~23 fJ per spike per channel [6], and electrohydrodynamic modulation via voltage-induced liquid film thickening and thinning [7], which successfully replicated the hibernation-like behavior observed in animals [8].
In this work, I will present an advantage of fluidic memristors that can restore the resistance, thus enhancing their endurance. Resistance drift constrains the accuracy of memristor-based neuromorphic computing due to resistance switching from residual ions in the junction. We demonstrate nanofluidic memristors using voltage-activated ion filling in asymmetrically concentrated electrolyte solutions within Ångström channels, enabling resistance switching. Inspired by the brain’s waste clearance mechanism, we replicated this process to remove residual ions, restoring conductance after 2 × 104 cycles, paving the way for endurance enhancement. Furthermore, our devices exhibit hour-long memory retention and low energy consumption of 60 nJ·cm-2 for the membrane and ~ 0.2 fJ per voltage spike per channel. By optimizing frequency, voltage, concentration gradients, and pH, we emulate short-term synaptic plasticity for adaptive learning. Finally, we demonstrated the first nanofluidic neuromorphic chip with a 4 × 4 memristor array, capable of recognizing mathematical operators using a pre-trained nanofluidic memristor array. Our work demonstrated the potential of fluidic memristor-based neuromorphic computing chips with long retention, low energy consumption, and restorable resistance.
1.2-I2
Asal Kiazadeh, PhD in Electronics and Optoelectronics, leads the Memristor Group at CENIMAT/i3N and serves as a lecturer at the University of Nova de Lisboa, Faculty of Engineering. She has extensive expertise in flexible oxide electronics and has secured five project grants as a Principal Investigator and key team member, funded by national government agencies and the European Commission. Her research focuses on memristor technology for advanced communication systems, such as THz non-volatile RF switches, and computational domains, including neuromorphic vision and brain-inspired low-power neural network hardware. She has authored over 40 research articles, with 30 of them dedicated to memristor technology.
Amorphous oxide semiconductor (AOS) technology has emerged as a transformative platform for the development of advanced memory and logic devices, particularly in the field of flexible electronics. This talk will explore the evolution of AOS-based devices, from Thin-Film Transistors (TFTs) to Resistive switching RAMs (RRAMs), and their integration into a range of computing paradigms. AOS materials, such as Indium Gallium Zinc Oxide (IGZO), offer unique properties like high mobility, low temperature processing, and excellent flexibility, making them ideal for next-generation electronics. We will first examine the capabilities of IGZO TFTs, which have demonstrated remarkable performance in various display, sensor, and neuromorphic applications, highlighting their role as efficient access transistors in RRAMs crossbar arrays. Next, we will discuss the transition to RRAMs and how these devices exploit the resistive switching mechanism for non-volatile memory, enabling high-speed, low-power data storage. The advantages of RRAM technology, including its scalability, endurance, and energy efficiency, will be emphasized, particularly in the context of emerging neuromorphic computing systems such as Reservoir computing (RC). We also discuss the integration of AOS-based RRAMs into flexible and wearable electronics, highlighting their potential to revolutionize both conventional and unconventional computing paradigms. The goal of this talk is to provide insights into the progress of AOS-based RRAM technology and to inspire further exploration of its potential to reshape the future of computing, from memory-intensive applications to neuromorphic and AI-driven systems.
1.3-I1
In recent decades, studies on molecular electronics have made significant advances and single molecular transistors have been demonstrated. Such investigations, however, have not directly led to actual molecular-scale electronic devices, due to the lack of effective technologies for wiring between molecules. Beyond single molecular transistors, the exploration of device architecture is a central issue in molecular-scale electronics. One of the attractive directions is the realization of neural networks that utilize self-assembled molecular systems.
We focus self-doped water-soluble polyaniline sulfonate (SPAN) exhibiting high conductivity and nanoscale network structure. In our previous work, we found that SPAN ultrathin films show ohmic conduction from 10 K to room temperature. However, SPAN indicates nonlinear I-V characteristics in microscale and humid condition at room temperature. These nonlinearities in SPAN network are useful for a physical reservoir computing (RC) by spoken-digit classification realized with 70 % accuracy [1].
Polyoxometalates (POMs) are generic designation for condensed transition metal oxyanions used for catalysis, electrode and other applications related with electron transfer. Recently, POMs attracted much attention for the molecular devices due to their multiple redox states including pentavalent and hexavalent Mo atoms. The I-V characteristics show strong memristor-type hysteresis with long time constant suggesting charging effect of mixed-valence system. Such phenomena is useful for classification tasks like a MNIST with 90 %.
1.3-I2
Prof. Tanaka completed his doctorate in materials science by studying the structural and magnetic properties of ferromagnetic nanoalloys at Osaka University in 1999. Then, moved to a national laboratory, RIKEN, to study the conductivity of metallic nanowires with double-probe scanning tunneling microscopy as a special postdoctoral researcher. After that, he advanced the molecular-ruler method in which precise multilayers of self-assembled molecular monolayers are used as lithographic resists to yield nanostructures with precise nanometer-scale spacings as a postdoctoral researcher at the Pennsylvania State University under Prof. Paul Weiss (presently UCLA, former chief editor of ACS Nano). Prof. Tanaka then joined the Research Center for Molecular-Scale Nanoscience at the Institute for Molecular Science in 2003 as an assistant professor, where he directed research in molecular electronics using carbon nanotube electrodes. He found that gold nanoparticles can switch from metallic conduction of SWNTs to semiconducting simply by nanoparticle adsorption. He has also focused on the development of atomic switches, exploring the ultimate miniaturization of electrical switches, and controlled by photo irradiation 2004-2008 in a key technology project of the Ministry of Education, Culture, Sports, Science, and Technology (MEXT) and receive an excellent journal award from Japan Society of Applied Physics in 2012. He moved to the Department of Human Intelligence Systems, Graduate School of Life Science and Systems Engineering, Kyushu Institute of Technology (Kyutech) as a full professor in 2014 and is focusing on bio-mimic and/or neuromorphic AI electric nanodevices such as material reservoir devices. He concurrently became a director of the Research Center for Neuromorphic AI Hardware, Kyutech, in 2020. He was awarded an honorary degree from Suranaree University of Technology, Thailand, in 2021 (see photo), and received the degree certificate from Thai HRH Princess Sirindhorn. He obtained a national project as a PI, ALCA-NEXT, during 2023-2026 supported by Japan Science and Technology Agency, focused on carbon neutral and green computing. He became a visiting professor of Universiti Putra Malaysia in 2024. His broad knowledge of materials, from metals and inorganic materials to organic materials, and techniques for measurement and fabrication helped lead efforts in molecular electronics and in combining nanocarbon and nanoparticles to realize a new world of materials intelligence in nanosystems.
In recent years, the exceptional computational power of software-based deep learning has gained widespread recognition, fueling the rapid advancement of artificial intelligence (AI) applications across diverse fields. However, the continual scaling of silicon CMOS technology—central to current computing systems—is approaching physical limits, posing a significant barrier to further performance improvements. As a result, there is growing interest in alternative hardware paradigms capable of sustaining future progress in AI. Among them, artificial neural networks (ANNs) are driving the development of neuromorphic hardware, particularly in the form of reservoir computing devices, to address energy efficiency challenges.
These next-generation AI systems depart fundamentally from conventional computing architectures by leveraging dynamic physical properties rather than static logic circuits. A key innovation lies in exploiting the intrinsic nonlinearity and interconnectedness of nanomaterials to engineer devices that naturally exhibit pulses, noise, and other complex physical phenomena. Such behaviors are essential for enabling real-time learning, adaptation, and decision-making, while also dramatically lowering power consumption and enhancing integration density.
A central requirement for learning in ANNs is the dynamic modulation and storage of synaptic weights, typically realized through weighted summation operations. To meet this need, our research center has been actively developing novel materials and device architectures that complement—or potentially outperform—traditional CMOS-based systems. In particular, we focus on molecular systems and carbon nanotubes (CNTs) as promising candidates for building energy-efficient, scalable AI hardware.
This work highlights our recent progress in realizing CNT-based reservoir computing devices. We explore critical aspects of their operation, including the ability to generate time-dependent responses and strategies to ensure stability and reproducibility in complex nanomaterial networks. Notably, we demonstrate a system in which pressure sensor signals from a robotic hand are input into a 2D random CNT network, enabling accurate classification of grasped objects [1]. Furthermore, we show that transforming a CNT network into a 3D sponge-like structure not only haptic sensing capabilities but also imparts physical reservoir computational functionality [2].
Beyond device-level innovations, we are applying these technologies to build autonomous AI robots. By embedding nanomaterial-based systems into robotic platforms, we aim to demonstrate intelligent behavior that emerges from the underlying physical computation, rather than from software algorithms alone. These robots serve as proof-of-concept for the potential of neuromorphic hardware in real-world applications such as sensor fusion, adaptive control, and embodied intelligence.
In the presentation, I will also review our latest research findings, emphasizing the interplay between material properties, device-level dynamics, and system performance [3]–[10]. We provide a comprehensive overview of our approach, including the material engineering, device design, and integration strategies that underpin our progress. Taken together, our results underscore the transformative potential of nanoscale physical phenomena to power the next generation of intelligent systems—paving the way for truly energy-efficient, adaptive, and integrated AI hardware systems.
1.3-O1
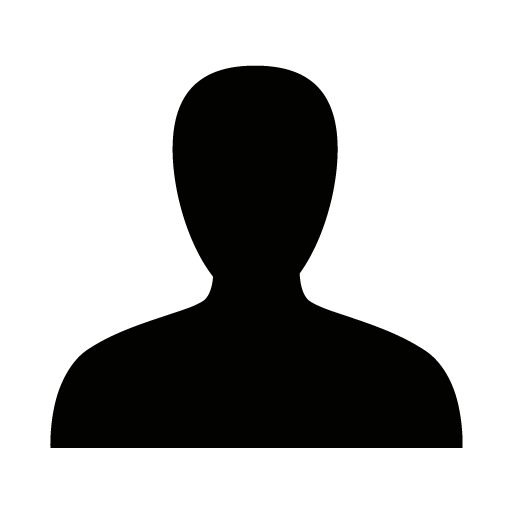
Physical reservoir computing (PRC) attracts considerable attention as a promising candidate to achieve high-performance and low-power machine learning (ML) at the edge region. We have focused on the electrochemical reactions of metal cations in ionic liquids (ILs), which derive fundamental functionalities as a physical reservoir device (PRD) [1], [2] between the input and read out layers in the ML model of the PRC. To improve the implementability of our electrochemical PRD, we developed the ionogel (IG) which are principally composed of the SiO2 nanoparticles and the IL containing Cu cations. IL used in the present study was 1-butyl-3-methylimidazolium bis(trifluoromethylsulfonyl)amide ([C4mim][Tf2N]). By using the reverse-offset printing technology [3], we prepared a flat IG layer on microfabricated electrode patterns and obtained the crossbar-type ionogel-based PRD (IGPRD) as shown in Fig. 1(a). The thickness of the IG layer was approximately 200 nm, while the line width of the top and bottom electrodes (TE and BE) in Fig. 1(a) was approximately 100 mm. By changing the line width of TE and BE, it was confirmed that the output currents from IGPRD scaled with the electrode area, indicating that the electrochemical reactions at the interface between IG and the planar section of the electrodes rather than the peripheral part of the electrodes generate the output currents. We evaluated the short-term memory (STM) property of the present IGPRD by applying the pseudo-random binary sequence as the triangular voltage pulse (TVP) train. The signals 0 and 1 were represented as the negative and positive TVP, respectively. Fig. 1(b) is the current-voltage (I-V) curves color-coded into eight classes according to the three-digit number corresponding to the history of the input signals 0 and 1 at three continuous timesteps. The pulse width (PW) of the TVP was set to 500 ms. The results in Fig. 1(b) clearly show that different current waveforms were generated by the IGPRD depending on the history of the input signals, indicating that the IGPRD has the STM property. The amount of the STM in the IGPRD is quantified as a memory capacity (MC) in the STM task [2]. The value of MC over the time delays from 1 to 4 timesteps was approximately 2.24 in the test phase, which is almost comparable to that for IL (MC = 2.39) evaluated using the planar-type PRD used in our previous study [1], [2]. The similar STM performance in the IGPRD was confirmed within the wide range of the PW conditions ranging from 50 ms to 500 ms. Therefore, the implementability of the electrochemical PRD was successfully improved by using IG patterns instead of IL, with keeping the STM task capability.
1.3-O2
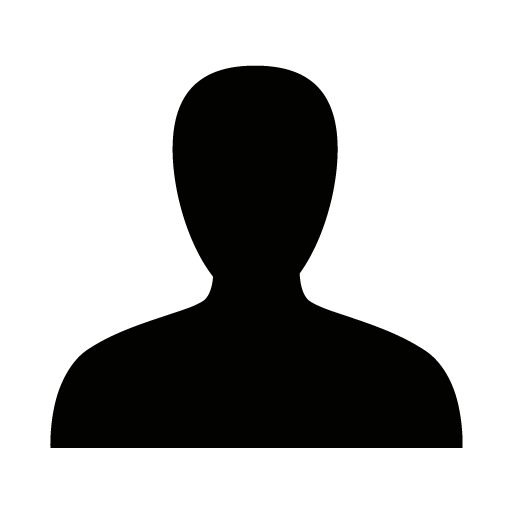
With the advent of novel and more pervasive electronic applications, like point-of-care ones, developing an electronic paradigm that can avoid conventional signal reconstruction appears crucial. Neuromorphic computing gives the opportunity to directly process and store information without the need for complex acquisition, storage units, and other peripherals. In view of a more sustainable approach to disposable electronic applications, combining the concept of neuromorphic computing with transient, biocompatible, and even metabolizable technologies could be a viable path for next-generation green devices. In particular, edible devices could be a game-changing technology in food and health-related sectors, where information on the quality of food and a patient's health can be easily obtained by a digestible, safe, low-cost electronic platform.
This work presents a neuromorphic system for spatiotemporal pattern recognition with short-term synapses and neurons based on fully edible EGOFET made of copper phthalocyanine (CuPc). The devices are fabricated starting from ethyl cellulose substrate and interdigitated source and drain contacts of gold nanoparticles, where CuPc is evaporated as to serve as the edible semiconductor. Chitosan is drop-cast on top of the semiconductor and silver is printed to finalize the electrolyte-gate structure. The slow ion movement inside the chitosan, which is responsible for the double layer that enables the channel modulation of the CuPc, opens a hysteretic window visible in the transfer curve of the devices. Short-term plasticity with controllable and repeatable potentiation is enabled by carefully tuning the amplitude and pulse duration of the gate stimulation. The devices exhibit spontaneous depression with a relaxation time in the order of a second, compatible with the time constants of the mechanisms that take place inside the brain. We exploit this synaptic behavior to implement a reservoir computing architecture for number recognition, where the pixels are encoded in spatiotemporal pulses, with accuracy higher than 90%. We then combine the edible synapse with an electronic neuron that oscillates when a suitable potentiation level is reached at the synapse, thus acting as an activation function. The resulting neuromorphic circuit displays high operational and ambient stability, on par with more mature technologies. These results support CuPc-based edible EGOFETs as suitable elements to implement neuromorphic building blocks and pave the way for more sustainable and compact point-of-care devices.
1.3-I3
Francesca Borghi is a tenure track assistant professor at the Physics Department of the University of Milano. She graduated in Physics from the University of Milano in 2011 and she received her PhD in Physics, Astrophysics and Applied Physics in 2015. Her research focuses on structural and functional properties of cluster-assembled nanostructured materials, and the development of neuromorphic computing systems and soft electronic devices. She’s currently coordinating multidisciplinary laboratories for the advanced characterization of neuromorphic systems at the Interdisciplinary Centre for Nanostructured Materials and Interfaces (CIMaINa) at the Physics Department (UniMi). She’s co-founder of GRUCIO, a start-up initiative aiming at the development of unconventional data processing devices.
The brain’s ability to perform efficient and fault-tolerant data processing is strongly related to its peculiar interconnected adaptive architecture, based on redundant neural circuits interacting at different scales. In such a system, individual neurons count for little; it is population activity that matters. Part of the difficulty in understanding population coding is that neurons are noisy, which means that the same pattern of activity never occurs twice, even when the same stimulus is presented.1 In this context, correlation and simultaneity within spiking activity of different neurons may play a crucial role in decreasing/increasing the amount of information that is decoded by population activity, in a non-trivial way.1 Furthermore, since cells are recurrently connected, forming a certain number of recursive loops, their own connectivity plays also a crucial role in the coordinated and synchronous firing of the population.2
On the artificial side, the exploitation of self-assembled systems with nonlinear dynamics has already been demonstrated as a possible unconventional strategy for energy efficient data processing tasks, relying on the emerging behavior of stochastic network activity.3,4 However, the encoding of the stimulus and the decoding of information in the response of such systems is still a challenge.
Here I will present and discuss results obtained on the spatial and temporal dynamic of the resistive switching activity of a nanostructured gold network, combining micro-thermography and electrical measurements. Cluster-assembled films produced by Supersonic Cluster Beam Deposition (SCBD)5 have been widely studied from both experimental and theoretical perspectives, as resistive switching systems that can be used for data processing tasks.6–10 The main mechanism at the nanoscale which causes the reversible changes in electric conductivity (10% to 105% from its initial value) is the stochastic reorganization of the grain boundaries in the nanogranular matrix of gold cluster-assembled thin films.11,12
The description and the understanding of the dynamic of local active sites which lead, at a larger scale, to the emerging electrical behavior of the network will be shown for the first time. The control obtained over the adaptive reorganization of the network will be proposed as an effective strategy to control the reversible connectivity and the tunable correlation between local active sites which promote the activity of the network at the microscale. This control allows the programmability of these systems used as reversible electronic switches and reconfigurable threshold logic gates (TLGs), implemented on both hard6 and soft substrates13 as complete functional systems for Boolean function classification tasks.14 We also demonstrated the potentiality of the use of these nanostructured devices to classify with high accuracy and in real-time neuronal traces, requiring limited datasets for training and memory storage.
The hardware implementation of such self-assembled neuromorphic materials will be proposed for the development of novel hybrid reprogrammable electronic architectures for data processing tasks employed on edge systems to efficiently interact with the environment.
2.1-I1
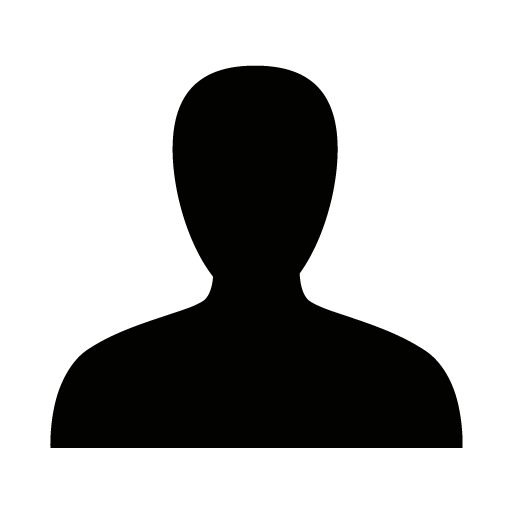
2.1-O1
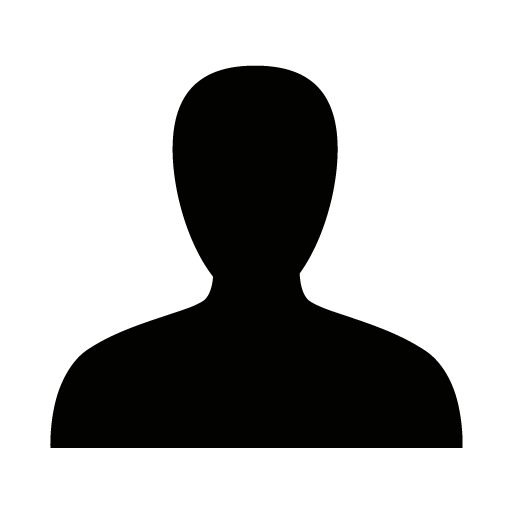
In recent years, the increasing power consumption of artificial intelligence (AI) has become a critical challenge, driving growing interest in novel computing paradigms inspired by the highly efficient information processing mechanisms observed in biological and neural systems. One promising approach is physical reservoir computing (PRC), which leverages the inherent spatiotemporal dynamics of materials and devices as a virtual neural network to process information. By exploiting the complex physical properties of various systems, PRC offers a fundamentally different approach to computation compared to conventional von Neumann architectures. Although PRC has been demonstrated in various physical systems[1,2], several key challenges remain unaddressed, preventing its practical implementation. These challenges include low computational performance, a narrow operational speed range, and the relatively large size of PRC devices, which hinder their scalability and integration into practical applications. Overcoming these limitations requires a novel strategy to fully harness the computational potential inherent in materials based on nanotechnology. To address this issue, we have developed ion-gating reservoirs (IGRs), an innovative PRC framework that utilizes the modulation of material properties via ion-gating as a computational resource. IGRs are transistor-structured devices composed of an ionic conductor and a semiconductor, enabling highly efficient and adaptive information processing. By applying this concept to a variety of material systems, we successfully demonstrated high-performance reservoir computing based on electric double-layer (EDL) effects, redox reactions, and molecular vibration modulations [2-6]. These mechanisms introduce dynamic and nonlinear responses, significantly enhancing the computational capacity of the reservoir.
In this presentation, we report on the development of IGRs across various material platforms and highlight the latest findings on ion-gel/graphene-based IGRs [7]. In this system, the interplay of multiple physical effects—including EDL-induced carrier injection, graphene ambipolar behavior, and charge trapping dynamics—gives rise to intricate spatiotemporal state evolution. This behavior is expected to contribute to network characteristics analogous to those found in biological neural systems, thereby enhancing the system’s computational versatility. To quantitatively assess the computational performance of our IGR, we conducted a benchmark task based on the Mackey-Glass equation, a well-known chaotic time-series prediction problem frequently used in machine learning evaluations. Our IGR exhibited prediction accuracy on par with deep learning (DL) models while achieving a two-order-of-magnitude reduction in computational load, highlighting its efficiency. Furthermore, we found that our system reached or even exceeded the theoretical computational efficiency limit observed in well-optimized simulation-based reservoir computing models, demonstrating its remarkable performance. These findings underscore the exceptional efficiency and computational potential of IGRs, positioning them as a promising candidate for next-generation computing technologies. By harnessing the complex dynamics of materials induced by ion-gating, IGRs provide a powerful platform for neuromorphic computing, which seeks to emulate the highly efficient and adaptive information processing observed in biological and neural systems. This work represents a significant step toward realizing energy-efficient, high-performance computing architectures inspired by nature, with potential applications in real-time signal processing, machine learning, and brain-inspired artificial intelligence.
2.1-O2
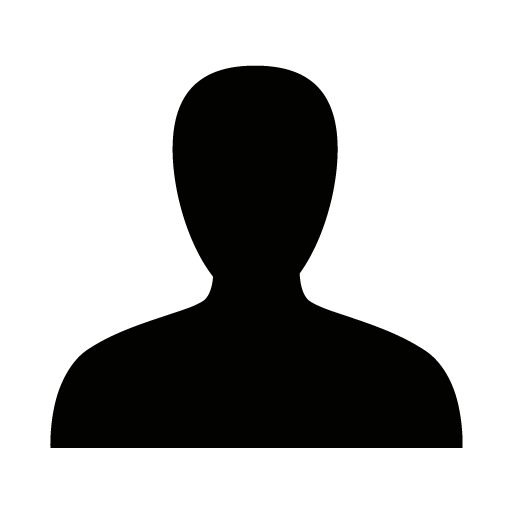
Zacharie Jehl is a French scientist specializing in semiconductors, solid-state physics, photovoltaics, and optoelectronics. He holds a PhD in Physics from Paris-Saclay University and has extensive international experience, having worked in France, Japan, and Spain. Currently, he is a tenure-track professor at the Polytechnic University of Catalonia (UPC), where he leads research on photovoltaic materials, low-dimensional semiconductors, and artificial synapses for neuromorphic computing. He has authored over 85 peer-reviewed publications, holds several patents, and has received prestigious grants, including the Ramon y Cajal and Marie Curie fellowships. Dr. Jehl is also the coordinator of the SOLIS European Project and actively collaborates with international research institutions.
As artificial intelligence continues to evolve and become ubiquitous, the limitations of traditional Von Neumann computing architectures become increasingly obvious, both in terms of energy consumption and for tackling complex, probabilistic tasks typical in real-world data. Inspired by the human brain, neuromorphic computing integrates memory and computation directly through a network of artificial synapses. As more than 80% of the brain’s input is visual, the development of artificial visual synapses is a very promising solution, particularly for edge computing, where data is processed locally, reducing latency and bandwidth usage. In this work, we explore the integration of ZnMgO/Se heterojunction photodiodes as artificial visual synapses, combining both photodetection and memory functions to enable ultra-low power neuromorphic systems.
We demonstrated last year the capability of these ZnMgO/Se photodiodes to mimic advanced synaptic behaviors through light-induced plasticity, leveraging the mechanism of trapping photocarriers in metastable interface defects at the ZnMgO/Se junction to simulate plasticity through persistent photoconductivity. However, our previous work required an external energy input to read the memory state of the system (read voltage). In this work, we demonstrate how these defects can modulate the system’s photocurrent in response to light stimulation in real time. Upon illumination, a non-volatile increase or decrease in the device’s photocurrent is observed, depending on the light intensity, duration, and photon energy. This phenomenon, referred to as the light soaking effect in thin-film solar cells, allows the photodiode to emulate fundamental synaptic functionalities with zero external power input beyond the visual stimulation, including short-term potentiation (STP), long-term potentiation (LTP), and their corresponding depression mechanisms. As the memory state of the synapse can be both written and read by monitoring changes in the short-circuit current (Jsc), it eliminsates the need for an external power source during operation.To characterize the synaptic plasticity, we perform a detailed analysis of the post-synaptic plasticity in response to various pulsed light stimuli and demonstrate short-term potentiation through paired-pulse facilitation (PPF). Our results show a significant increase in the PPF ratio as a function of the inter-stimulus interval reduction. Moreover, we investigated spike rate-dependent plasticity (SRDP), where the device exhibited enhanced photocurrent responses at higher stimulation frequencies, as well as spike timing-dependent plasticity (STDP), mimicking biological synapses. This precise temporal control over plasticity opens the door to complex learning algorithms within visual neuromorphic systems, without the need for a read voltage, as all measurements are performed in short-circuit conditions. Remarkably, we demonstrate the possiblity of analogically switching between potentiation and depression by stimulation with different wavelengths. This significantly increases the possibilities in terms of programming states, and provides important insights is terms of physical interpretation of the mechanism responsible for the charge trapping-detrapping at the p-n interface.
Our study demonstrates the potential of inorganic thin-film ZnMgO/Se heterojunctions in visual neuromorphic computing, positioning these devices as a promising candidate for future hardware-based AI-driven applications.
2.1-I2
Juan Bisquert (pHD Universitat de València, 1991) is a Professor of applied physics at Universitat Jaume I de Castelló, Spain. He is the director of the Institute of Advanced Materials at UJI. He authored 360 peer reviewed papers, and a series of books including . Physics of Solar Cells: Perovskites, Organics, and Photovoltaics Fundamentals (CRC Press). His h-index 95, and is currently a Senior Editor of the Journal of Physical Chemistry Letters. He conducts experimental and theoretical research on materials and devices for production and storage of clean energies. His main topics of interest are materials and processes in perovskite solar cells and solar fuel production. He has developed the application of measurement techniques and physical modeling of nanostructured energy devices, that relate the device operation with the elementary steps that take place at the nanoscale dimension: charge transfer, carrier transport, chemical reaction, etc., especially in the field of impedance spectroscopy, as well as general device models. He has been distinguished in the 2014-2019 list of ISI Highly Cited Researchers.
The potentiation and depression of synaptic conductivity regulate the plasticity and adaptability of synapses. In this discussion, we examine the general dynamic characteristics of ionic or electronic current conduction in memristors, which underpin the fundamental principles of synaptic activity. Key model requirements for memristors or chemical inductors to achieve conductance adaptation in response to incoming stimuli are outlined. We also propose various criteria, such as hysteresis and rectification, to achieve these properties. Additionally, we describe a range of diagnostic methods that link nonlinear time responses, the nonlinear cycling of current-voltage curves, and the linear frequency responses from impedance spectroscopy to evaluate adaptation properties.1,2 The frequency domain analysis of memristors and more generally, of conducting systems with memory features, provides essential information about the dynamic behaviour of the system. The impedance response of a memristor can be represented as a linear circuit made of resistances, capacitors, and inductors, with voltage-dependent elements. The equivalent circuit properties also establish the criteria for a Hopf bifurcation that produces spiking of artificial neurons.3,4
2.2-I2
In this talk, I will explore the intersection of artificial intelligence (AI), advanced materials, and novel computing architectures, focusing on the development of bio-inspired circuits for neuromorphic and edge sensing applications. By leveraging the unique properties of two-dimensional (2D) materials, such as MoS2, WSe2, and graphene, I will demonstrate their potential in enabling energy-efficient, scalable, and highly adaptable circuits that emulate biological neural systems. These circuits are particularly well-suited for edge computing and sensing tasks, where compactness and power efficiency are critical.
One example of this approach is leveraging AI and machine learning (ML) to enhance the performance of graphene-based electronic tongue for food safety, environmental monitoring, and healthcare applications (Nature, 634, 572–578, 2024). Furthermore, we have demonstrated bio-inspired computing paradigms that replicate complex biological processes, including auditory processing in barn owls, collision avoidance in locusts, probabilistic computing in dragonflies, and multisensory integration in octopuses. By combining 2D materials with bio-inspired principles, we are paving the way for compact, functionally diverse integrated circuits that harness the power of neuromorphic processing.
Finally, beyond bio-inspired circuit design, I will delve into the transformative potential of monolithic 3D integration using emerging 2D field-effect transistors (FETs). Our recent breakthroughs in wafer-scale 2-tier and 3-tier 3D integration with MoS2 and WSe2 FETs have enabled the realization of multifunctional circuits, paving the way for the next generation of logic and memory devices (Nature, 625, 276-281, 2024). These advancements are critical for overcoming the limitations of conventional scaling and unlocking new possibilities in high-density, low-power electronics, particularly for mimicking the three-dimensional structure of the brain.
2.2-O1
2D materials are a relatively novel class of resistive switching materials. Neuromorphic devices can be prepared in a variety of ways with these layers, mainly differing between lateral and vertical configurations.[1] Vertical devices are typically advantageous for integration in crossbar arrays. The technique for depositing the 2D materials is the main bottleneck for achieving high quality layers while maintaining fabrication cost-effective, environmentally friendly and compatible with conventional microelectronic processing.
In this contribution, the first vertical solid-state MoS2 memristors using liquid-liquid interface assembly is presented. This deposition technique produces conformal coatings of > 1 μm wide sheets on large areas and does not require the hazardous gases required for chemical vapor deposition.[2] Devices are presented with contacts by physical vapor deposition and patterned by photolithography as well as with printed metal electrodes. The memristors work both in bipolar and unipolar fashion. Electrical characteristics comprise static IV and pulsed switching parameters, switching energy as well as insertion losses up to 110 GHz.
2.2-O2
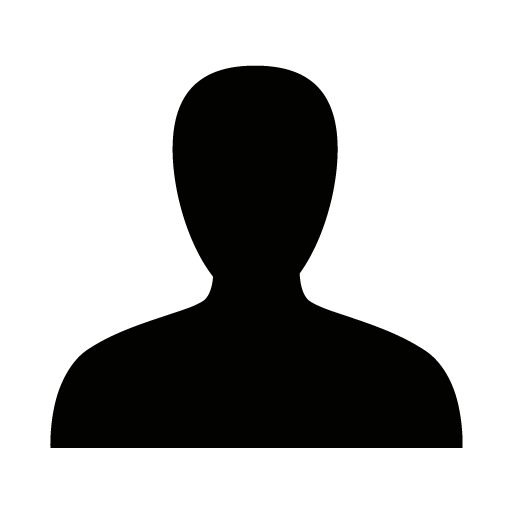
Energy inefficiency in traditional silicon-based CMOS neuromorphic computing limits the development of advanced artificial intelligence systems. Although CMOS-based in-memory computing reduces the energy required to move data, it suffers from material limitations, including high operating voltages and inability to emulate analogue computation. MXenes are a recently discovered family of 2D transition metal carbides and nitrides
In this study, we fabricated planar Ti₂CTx MXene-based memristors through optimised thin-film deposition. Systematic I-V measurements revealed that the devices exhibit strong retention, high endurance, and low power consumption, outperforming 2D material counterparts. The device properties of MXene-based memristors were then simulated in a neuromorphic crossbar array using the AIHWkit simulator. The memristors were integrated into a spiking neural network with a three-layer architecture of leaky integrate-and-fire neurons for handwritten digit classification on the MNIST dataset. Various characterisation tools, including SEM, XRD, XPS, AFM, and Raman spectroscopy, were employed to analyse the structural, morphological, and chemical properties of the material, providing insights into its impact on device performance.
2.2-O3
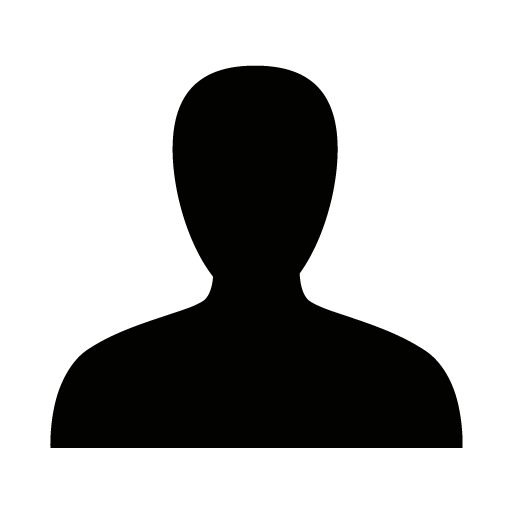
Ionic effect has shown to be an effective approach to tune electrical, ferroelectric and magnetic properties of materials, providing new opportunities in developing robust and high-performance devices for sensors, memories, logics, and neuromorphic applications. Memristive devices working with ionic processes have drawn particular attention for implementing artificial synapses and neurons for brain-inspired computing. Ionic control is a novel approach to modulate memristive functionalities to realize synaptic plasticity and neuronal dynamics, due to the similar dynamic electrochemical processes with biological systems. This improves computation efficiency and area efficiency compared with present CMOS based electronic devices. This work will introduce the fundamentals of ionic-based memristive device, including the electrochemical redox reactions and ionic motion processes in solid-state memristive devices. Specifically, ionic process in transition metal oxides and two dimensional materials will be talked. Depending on the ion species and valences, various memristive switching modes with high stability and optimal device performance can be achieved.
2.2-I1
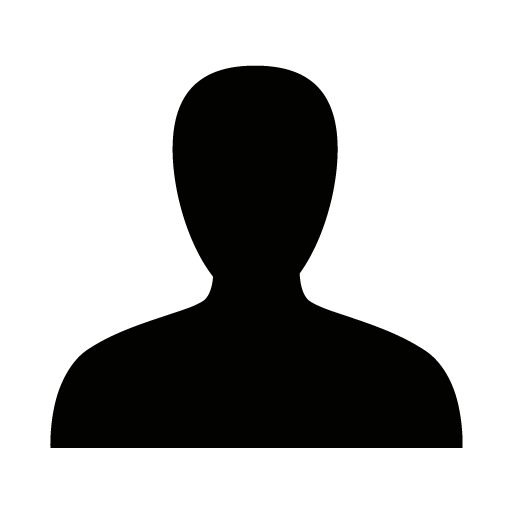
Memristors could mitigate the limitations of von Neumann architecture, empower brain-mimicking capabilities, and lower energy demand. However, advanced neuronal functions and long-term memory among other technical issues remain challenging to establish. Our group have adopted a combined experimental and simulation approach from fundamental nanoelectrochemistry perspective to study ion transport dynamics confined through ordered asymmetric nanogeometry and its defined nanointerfaces. This talk will introduce our recent findings based on two types of nanofluidic-type devices, both featuring broken symmetry along the transport trajectory. In the first system, we make individual conical quartz nanopipettes that can hold the same or different solutions inside and outside. As a scaled-up ensemble system, anodized aluminum oxide membranes containing arrays of nanochannels are synthesized as separators of two solutions. Characteristic memristor responses of pinched current–potential loops are resolved in voltammetric experiments and successfully reproduced through finite element simulation. Excitatory and inhibitory conductance states are shown to arise from the enrichment and depletion of mobile charge carriers, conveniently tuned by the pulse waveform. Negative differential resistance, a decrease in conductance with increasing potential, is shown to arise from the availability and redistribution of the ionic charges during the hysteretic and rectified transport at asymmetric nanointerfaces. Mechanistic understanding and controllable state-switching dynamics shine light on avenues to achieve higher-order complexity functions.
2.3-I1
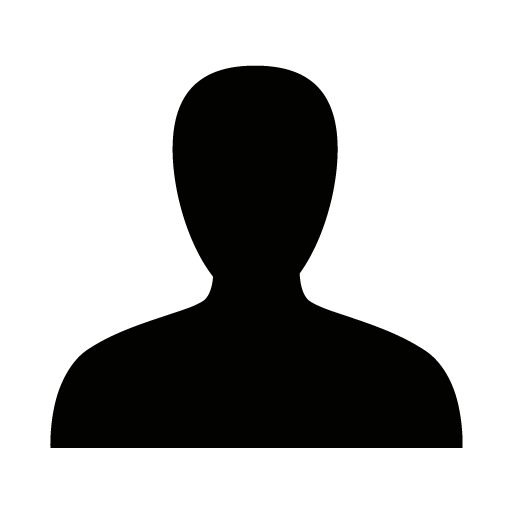
The increasing complexity of modern neural network architectures has led to a substantial rise in energy consumption during both training and inference, especially when relying on conventional CMOS hardware based on the von Neumann architecture. The continuous exchange of data between memory and processing units represents a major bottleneck, limiting both efficiency and speed in artificial neural network (ANN) computations. To address these challenges, specialized neuromorphic architectures leveraging analog memristor crossbar arrays have emerged as a promising alternative, offering improved energy efficiency and computational speed for AI workloads, by enabling some arithmetic and logic operations to be performed directly at the location where the data is stored [1].
Recent implementations of the analog in-memory computing (AIMC) paradigm have primarily focused on accelerating the inference step (i.e., the forward pass) of digitally trained deep neural networks (DNNs), even though the training phase is orders of magnitude more demanding in terms of time and energy costs. This is because analog training acceleration imposes even more stringent requirements on memristive devices. In addition to performing inference, the learning phase requires handling error backpropagation, gradient computation, and weight updates. Promising memristive technologies that could address these challenges include redox-based resistive switching memory (ReRAM) [2] and electrochemical random access memory (ECRAM) [3]. However, a unified analog in-memory technology platform—capable of on-chip training, weight retention, and long-term inference acceleration—has yet to be demonstrated.
This work fills this gap by demonstrating an all-in-one AI accelerator based on Conductive Metal-Oxide (CMO)/HfOx ReRAM technology, enabling the execution of forward and backward passes, along with weight updates and gradient computations, directly on a unified analog in-memory platform with O(1) time complexity [4]. The CMO/HfOx ReRAM devices are integrated in the BEOL of a NMOS transistor platform in a scalable 1T1R array architecture. The highly reproducible forming step demonstrates compatibility with NMOS rated for 3.3 V operation, while the uniform quasi-static cycling characteristics, achieved with voltage amplitudes of less than ± 1.5 V, exhibit a significant conductance window and a low off-state. The multi-bit capability of more than 32 states (5 bits) as well as the record-low programming noise ranging from 10 nS to 100 nS will be presented. Inference performance is validated through matrix-vector multiplication simulations on a 64×64 array, achieving a root-mean-square error improvement by a factor of 20 at 1 second and 3 at 10 years after programming, compared to state-of-the-art. Training accuracy closely matching the software equivalent is achieved across different datasets using the same technology.
The CMO/HfOx ReRAM technology lays the foundation for efficient analog systems accelerating both inference and training in deep neural networks.
2.3-I2
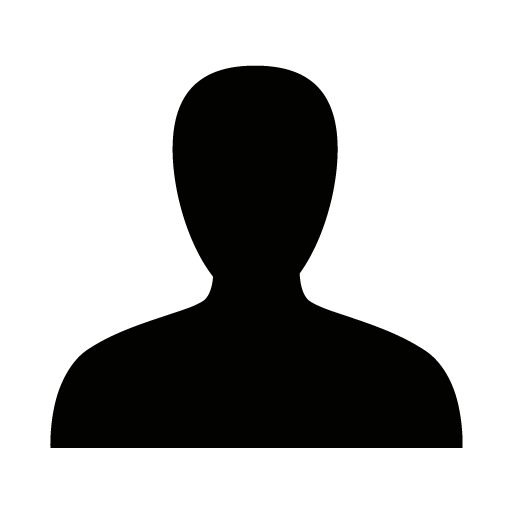
The recent rapid progress of AI technology has made our lives more convenient and comfortable, but the energy required for information processing has also increased dramatically. Therefore, we are focusing on the brain, which can perform highly intelligent information processing with extremely low energy and aiming to create a neuromorphic computer that realizes ultra-low power AI processing by imitating the brain’s information processing principle in hardware. We are particularly interested in analog spiking neural network (SNN) hardware, in which information is not represented by digital data but by trains of voltage spikes that propagate in the network of analog neuronal circuits to process information. For learning with SNNs, neurons and synapses should have “plasticity”; parameters such as firing thresholds and synaptic efficacies change dynamically in accordance with their own spiking activities and stay unchanged if no spiking occurs. In addition, it has been argued that uncertainty i.e. variability and/or stochasticity in their dynamics should improve the performance of learning. One of the essential aspects in developing analog SNN hardware is how to implement such plasticity and uncertainty with electronic devices. The resistive change device, often being referred to as the memristor, is a promising candidate. We are currently conducting research into how the plasticity and uncertainty of neurons and synapses affect the information processing performance of analog SNN hardware. In this presentation, we will introduce these results and discuss the possibility of applying memristors.
2.3-O1
Wataru Namiki received his Ph.D. degree from Tokyo University of Science in 2021. He joined the National Institute for Materials Science (NIMS) as Postdoc researcher in 2021 and is currently working as Researcher in the Ionic Devices Group of the World Premier International (WPI) Research Center for Materials Nanoarchitectonics (MANA), the NIMS. His current research focuses on high-efficient neuromorphic computing devices including physical reservoir computing based on ionic and spintronic phenomena.
While the development of artificial intelligence on cloud computer has made people's lives more convenient and affluent, the increase in electric power consumption and a load of network will be caused by the explosive growth in the amount of information flying through society in the future. Although this problem could be greatly improved if the information could be processed at an edge device where the information was acquired before sending the data to the cloud, this scheme has not been realized so far due to high computing cost resulting from the complexity of the network structure. Among various machine learning models, reservoir computing, in which only readout weights are trained, has the advantages of low computing cost and fast processing speed due to its simple and small network structure. In particular, development of physical reservoir that is a physical device satisfying distinctive properties required to reservoir (i.e., nonlinearity, short-term memory, and high dimensionality) is a promising approach to realize compact artificial intelligence integrated on the edge device (i.e., edge computer) and is energetically attempted with various physical devices.[1,2] However, some fatal issues remain on the road to said implementation as follows; low computational power, high electrical power consumption, and large device volume. Although it was theoretically found that spintronic physical reservoir utilizing nonlinear interfered spin wave can overcome these issues, the physical reservoir had not been experimentally demonstrated so far.[3]
Very recently, we experimentally demonstrated a physical reservoir utilizing nonlinear interfered spin wave multi-detection for the first time [4,5] and achieved the best benchmark (the lowest processing error of 1.81 × 10-2) in physical reservoirs at that time.[4] This excellent performance results from strong nonlinear dynamics characterized as chaos, which has not been observed in the theoretical demonstration. Here, we developed an innovative physical reservoir based on the physical reservoir using the chaotic interfered spin waves, namely iono-magnonic reservoir, toward further improvement of the computational performance. The iono-magnonic reservoir consists of spin wave propagating ferrimagnet Y3Fe5O12 (YIG) and proton conducting polymer Nafion and combines the spin wave interference (spintronics) and an ion-gating driven by external electric field (ionics). Protons migrate from Nafion to YIG by gate voltage application, leading to spin wave frequency and amplitude variations through magnetization and magnetic anisotropy manipulations. The manipulated spin wave can be used reservoir computing, and the processing error is successfully reduced to 9.53 × 10-3 from 1.81 × 10-2.[6]
In the presentation, we will report details of the iono-magnonic reservoir, including a mechanism of spin wave manipulation through ion-gating. The physical reservoir improved by physical dynamics manipulation will be keys to realize the edge computer.
2.3-O2
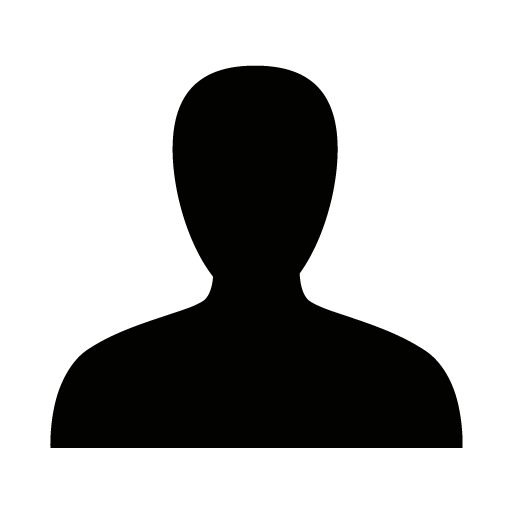
Spin-wave reservoir computing (RC) enables effective neuromorphic computation by utilizing the spatiotemporal dynamics of spin waves in a magnetic thin film. The properties of spin-wave RC systems, governed by the Landau-Lifshitz-Gilbert (LLG) equation, can be complex and may differ from those of software-based RC systems, such as echo-state networks. Consequently, a deeper understanding of spin-wave dynamics and their role in neuromorphic computing is crucial for optimizing system design and advancing practical applications. In this work, we propose an analytic-signal-based input-output model for spin-wave RC systems, enabling direct computation of system responses without requiring physical experiments or numerical simulations, given knowledge of the system's standard responses at low input power. The model demonstrates that individual reservoir outputs capture local spin-wave responses with information encoded in two dimensions with their respective step-response functions. Building on this, we propose passband signal learning, where spin-wave responses are directly fed to the readout for learning without signal demodulation. Passband signal learning not only reduces system peripherals but also leverages the wave-based characteristics of the system's responses for more efficient information learning. We evaluate the effectiveness of this approach in mitigating multipath interference (MPI), in comparison to the conventional learning method. Our results show that spin-wave RC systems with passband signal learning more effectively address the MPI problem, owing to the inherent compatibility between spin-wave dynamics and communication channels. This work is expected to provide valuable insights into spin-wave physics and advance the development of spin-wave-based neuromorphic computing systems.
2.3-I3
The rapid development of artificial intelligence in the big data era has increased the demand for solving complex combinatorial optimization problems (COPs) such as traveling salesperson problem and vehicle routing problem (VRP), which are difficult to efficiently handle with conventional von-Neumann computing systems. Quantum computing, which uses quantum bits (qubits) that utilize the superposition of spin as ‘0’ and ‘1’, shows the potential to solve these COPs efficiently. However, constructing cryogenic operating environments to not only eliminate unwanted noise but also accurately detect the intrinsic spin behavior remains a noticeable drawback. To address these challenges, a new probabilistic computing (P-computing) has recently been proposed as an alternative to emulate the quantum supremacy even at room temperature.
Here, we showed probabilistic bits (P-bits) with a novel Ti/SiOx/Ti stack, which is a fundamental building block for P-computing. The SiOx layer typically exhibits reliable TS behavior, resulting in robust voltage oscillations. When a Ti scavenging layer was introduced at the interface, oxygen vacancies were provided to the SiOx, causing oscillation to occur probabilistically. Through physical analysis and numerical calculations, the underlying mechanism of P-bit operation in Ti/SiOx/Ti stack was investigated by considering the charging and discharging process. This results in a sigmoidal probability curve for ‘1’ over a wide range of input voltage. Therefore, leveraging the developed SiOx-based P-bit can speed up the algorithm for finding the optimal path in the vehicle routing problem, which is verified through MATLAB simulation.
2.3-I4
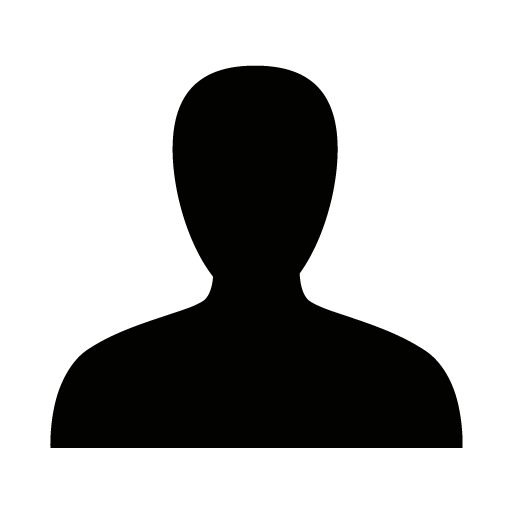
3.1-I1
Hao-Wu Lin graduated from National Taiwan University in 2002 with a bachelor’s degree in electrical engineering. He received his Ph.D. degree from graduate institute of photonics and optoelectronics, National Taiwan University in 2007. After his Ph.D. study and military service, he joined AU Optronics Corp. for research and development on organic light emitting displays. In 2009, he joined the faculty of National Tsing-Hua University in the department of materials science and engineering and now act as a full professor and the group leader of advanced optoelectronic materials laboratory (AOML, http://mx.nthu.edu.tw/~hwlin/). His current research interests include organic and halide perovskite semiconductors for optoelectronic and electronic devices, next-generation photovoltaics, flat panel displays, quantum emitters, hardware-based neuromorphic computing, and nano science and technologies.
Halide perovskites, known for their remarkable optoelectronic properties beyond solar energy conversion, are increasingly explored for memristive devices, alongside the broader class of metallic halides. This presentation focuses on utilizing these materials for fabricating and understanding memristors. Memristors function as non-volatile memory elements capable of storing a continuum of resistance states, rather than merely discrete binary values. This inherent analog characteristic positions them as ideal candidates for developing energy-efficient, hardware-based neuromorphic computing architectures that emulate biological synaptic functions.
The underlying physical mechanisms governing the continuous conductance modulation (resistive switching) in halide perovskite memristors are multifaceted and critical to device performance. Key processes include ion migration leading to the formation and rupture of conductive filaments, as well as charge carrier trapping and detrapping dynamics associated with defect states. A thorough understanding and control of these mechanisms are paramount for engineering devices with desired characteristics like linearity and endurance.
This talk will provide an overview of the halide perovskite memristors, covering the fundamental physics, materials science considerations influencing switching behavior, and device engineering strategies. We will examine characteristics of both two-terminal device structures and three-terminal architectures.
3.1-O1
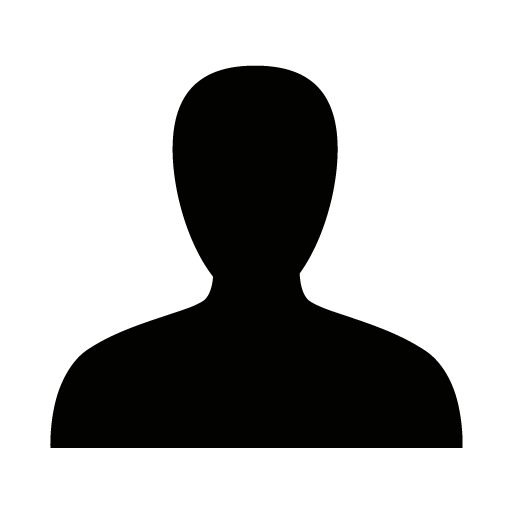
In the era of artificial intelligence (AI), which is deeply integrated into our daily lives, big data processing technologies and AI memory systems are gaining significant attention as key solutions to overcome the bottleneck limitations of the von Neumann architecture. Among various next-generation memory devices, halide perovskite (HP)-based memristors are emerging as promising candidates for neuromorphic computing systems due to their unique ionic, electrical, and material properties. These include high ionic mobility, low power consumption, ease of fabrication, flexible integration compatibility, and non-volatile memory behavior. However, there remains a lack of comprehensive dynamic physical analysis and detailed understanding of the operating mechanisms and characteristics of HP-based memristors and neuromorphic devices, which are crucial for realizing high-performance, accurate future applications.
In this work, I present an in-depth exploration of the various characteristics, operating principles, and dynamic physical models of HP-based memristors and neuromorphic devices. First, I classify the different switching mechanisms based on the arrangement and movement of cations (such as electroactive metals like Ag, Cu, etc.), anions (halide ions), and vacancies within the HP layer. I will then explain how these switching types correspond to specific memory and neuromorphic device applications. Additionally, I introduce physical models, including impedance spectroscopy and time response analysis, to investigate the drift-diffusion dynamics of resistive switching properties driven by ion migration in the HP layer. These dynamic behaviors are essential not only for memory devices but also for mimicking biological synapses, enabling the integration of HP-based memristors into neuromorphic computing architectures.
Ultimately, this presentation offers a comprehensive overview of the mechanisms and electrical actuation in memory and neuromorphic systems, providing valuable insights from multiple perspectives for future high-performance applications.
3.1-O2
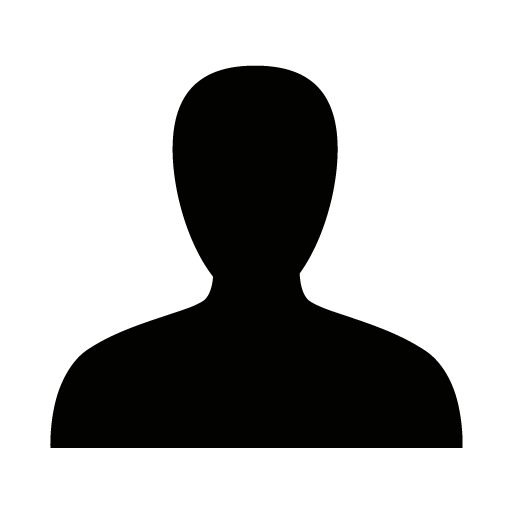
Artificial tactile synapses are neuromorphic devices that mimic the human skin's ability to perceive touch. They can detect subtle touches and convert pressure signals into electrical signals to stimulate the response of artificial synapses. These sensors can be trained to recognize different patterns of pressure, texture, and vibration. However, most current artificial tactile synapses are still mainly focused on rigid or flexible devices, limiting their applications in wearable electronics and intelligent sensing. Therefore, this study integrates the conjugated polymer PDBT-co-TT doped with ionic salts and the elastic polymer SBS as semiconductor layer materials to fabricate an artificial synapse transistor with synaptic plasticity. Simultaneously, it combines a pyramid-microstructured perovskite CsPbBr3 material with a polydimethylsiloxane (PDMS) thin film-based triboelectric nanogenerator (TENG). By converting the mechanical energy generated by pressure into electrical energy, it drives the artificial synapse transistor and utilizes a deep neural network (DNN) for Fashion MNIST image recognition.
The triboelectric nanogenerator prepared in this study demonstrates excellent performance in pressure sensing applications. It exhibits an output voltage as high as 89V under a pure pressure of 2.5 kPa, and achieves a sensitivity of 159.58 VkPa⁻¹ within a smaller pressure range. Furthermore, the device can effectively convert triboelectric charges into electrical energy to drive the artificial synapse transistor, thereby integrating a self-powered artificial synapse system. This system can exhibit the long-term potentiation (LTP) and long-term depression (LTD) effects of biological synapses, and utilizes these plasticity features as synaptic weights for a deep neural network (DNN) to further demonstrate the clothing image recognition capability on the Fashion MNIST dataset. This research not only achieves high-performance, low-power tactile sensing and artificial synapse simulation technology, but also provides a new technical pathway for the development of future intelligent sensing, self-powered neuromorphic computing, and bioelectronic fields.
3.1-I2
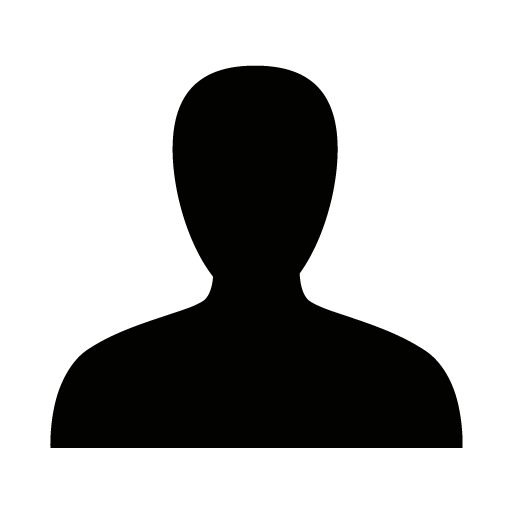
Halide perovskite, an old material, has emerged as a class of material with unique optoelectronic properties showing excellent performances for many applications ranging from solar cells to light-emitting and light detection devices. Recently, halide perovskite compounds have been demonstrated as promising candidates for neuromorphic computing devices owing to their material properties such as ambipolar charge transport, strong light absorption, carrier trapping effects, phase transition, ionic conduction, and unique properties like photo-induced ion migration and defect chemistry. In this talk, we will present recent advances of memristors based on halide perovskite compounds. By analyzing their materials chemistry and structure, fundamental operating mechanisms and device architectures, applications of perovskites in memristor and artificial synaptic devices will be presented. In the end, future prospective and opportunities for developing perovskite-based neuromorphic electronics will be discussed
3.2-O1
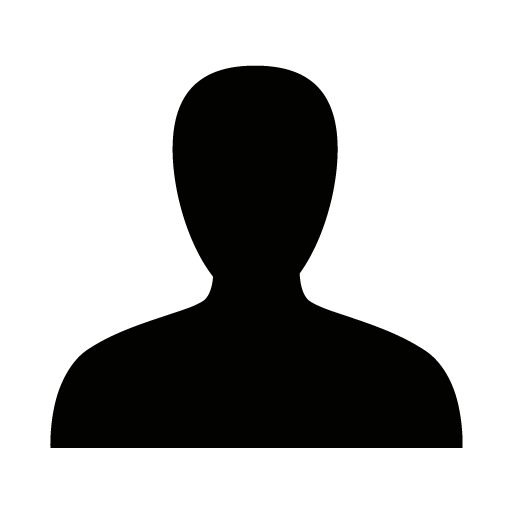
Title: In memory neuromorphic computing in ZnO@ß-SiC based artificial synaptic device
Abstract: The evolution of neuromorphic computing is crucial on the development of advanced resistive random-access memory (RRAM) technologies capable of emulating biological synapses, a key requirement for next-generation artificial intelligence systems. Traditional metal oxide-based RRAM devices, however, face limitations in accurately replicating synaptic behaviors. In this study, a ZnO@β-SiC composite-based RRAM device is introduced, demonstrating self compliant, forming-free resistive switching (RS) at approximately 0.8 V. Notably, the device emulates crucial synaptic functions such as potentiation, depression, and paired-pulse facilitation at low voltage stimuli (~0.6 V, 40 ms), exhibiting both learning and forgetting dynamics. Synaptic plasticity is further explored through spike-rate, spike-number, and spike timing dependent protocols. A transition from short-term to long-term plasticity is observed with increased training pulses and reduced interval durations. The underlying RS mechanism is attributed to electric field-induced formation and rupture of conductive filaments formed by oxygen vacancies. The material's chemical and electronic structures are analyzed using x-ray photoelectron and x-ray absorption spectroscopy, while conductive atomic force microscopy and impedance spectroscopy provide insights into its memristive behavior and electrical characteristics.
3.2-I1
New hardware technologies are necessary to keep up with the major demand in complexity and energy efficiency required by artificial intelligence algorithms. Brain-inspired computing is very promising, since the brain is capable of continuously learning from new data, while consuming only a small fraction of energy that would be consumed in the existing artificial intelligence systems. The reason could be that current artificial intelligence systems have limited biomimicry, as exemplified by deep neural networks with simplistic neuronal and synaptic dynamics. Their training is usually executed in microprocessors, graphics processing units or tensor processing units based on existing digital hardware technologies with poor energy efficiency for these tasks. By comparison, emerging analog hardware technologies, like memristors, show promise for dense energy efficient systems given their ultra-scalable footprint and better energy/bit consumption. I will present our memristor synaptic devices, the integration with CMOS neuronal circuitry as well as our modular mixed-signal prototyping platform which was collaboratively designed for benchmarking memristive neural networks of up to 20,000 memristor devices. However, issues related to device non-idealities prevent practical adoption at scale. By comparison, work in neuroscience seems to indicate that the brain can perform astounding computation with unreliable synapses and in the presence of input noise. The hippocampus is a core region of the brain with a major role in learning and memory and it features of diversity of neuronal types with various connectivity patterns. I will describe our efforts to draw inspiration from the hippocampus to design efficient and robust neuromorphic computing. I will summarize our interdisciplinary efforts across the innovation stack, from new types of materials and synaptic devices to new types of prototyping systems and hippocampal-inspired algorithms.
3.2-I2
Combinatorial optimization problems (COPs) are prevalent in social life and industry, with applications in computer science, engineering, chemistry, logistics, economics, and more. However, they are notoriously difficult to solve exactly. Analog computing in memristor hardware has shown potential for heuristic solutions, but current demonstrations are limited to series updates due to the constraints of simulated annealing and discrete-time Hopfield neural network models. In this talk, I will introduce our recent efforts in developing analog memristor hardware for efficient optimization problems. First, we propose borrowing concepts from quantum adiabatic annealing to the memristor-based Ising solver, achieving better parallelism by synchronously updating node states. We also adopt a binary neural network-inspired updating algorithm, experimentally demonstrating better efficiency and solution quality. Additionally, we developed a continuous-time version by employing the power minimization principle for natural energy gradient descent. By continuing to push the boundaries of analog computing, we hope to unlock new possibilities in optimization and contribute to advancements in various fields reliant on these complex problem-solving capabilities.