The program is in CET time
1.1.-K1
Giacomo Indiveri is Professor of Neuromorphic Cognitive Systems at the University of Zurich and ETH Zurich. He holds an MSc degree in electrical engineering and a PhD degree in computer science. His interests lie in the design of mixed signal neuromorphic electronic systems and learning circuits.
For many practical tasks, that involve real-time processing of sensory data and closed-loop interactions with the environment, conventional artificial intelligence neural network accelerators cannot match the performance of biological ones.
One of the reasons for this gap is that neural computation in biological systems is organized in a way that is very different from the way it is implemented in today's deep networks. In biological neural systems computation is tightly linked to the properties of their computational embodiment, to the physics of their computing elements and to their temporal dynamics.
Recently developed brain-inspired hardware architectures that emulate the biophysics of real neurons and synapses represent a promising technology for implementing alternative computing paradigms that bridge this gap. Memristive devices are a key element of this technology.
In this talk I will present hybrid analog/digital microelectronic and memristive circuits that use their physics directly emulate the biophysics of the neural processes and memory elements they model, and brain-inspired architectures that integrate massively parallel arrays of such circuits to implement on-chip on-line learning and computation.
I will discuss the advantages and disadvantages of these types of computing architectures and argue that they represent a promising approach for applications that need to process input data as it arrives in real-time without having to use eternal memory storage.
1.1.-I1
Arindam Basu received the B.Tech. and M.Tech. degrees in ECE from the I.I.T, Kharagpur, India, and the M.S. degree in
Mathematics and the Ph.D. degree in ECE from the Georgia Institute of Technology,
Atlanta, GA, USA. He is currently a Professor with the Department of EE, City University of Hong Kong and was a tenured faculty at NTU, Singapore previusly.
Dr. Basu was included in Georgia Tech Alumni Association’s 40 under 40 list in 2021 and was awarded the MIT Technology Review’s TR35 Asia Pacific Award in 2012. He also received the Prime Minister of India Gold Medal from I.I.T Kharagpur in 2005. He and his students have received several best paper awards and nominations in IEEE conferences.
He has served as IEEE CAS Distinguished Lecturer from 2016 to 2017 and currently serves IEEE in various roles such as TC Chair, Associate editor of journals etc.
In this talk, I will describe how the concept of neuromorphic In-memory computing (IMC) has evolved over time. I will share some of our work on IMC spanning different flavours: volatile and non-volatile memory based, chip scale to large area covering flexible designs etc. Concrete applications of IMC employed in a system to process event streams from neuromorphic vision sensors in traffic monitoring will be shown as a means to enable the Internet of Video Things. I will also talk about some of the lessons learnt in working in cross-disciplinary teams spanning circuits, algorithms, devices, material scientists and physicists.
1.1.-K2
This talk addresses redox-based resistive switching devices also called nanoionic memories or memristive elements, operating according to the bipolar valence change mechanism (VCM), which has become a major trend in electronic materials and devices over the last decade due to its high potential for non-volatile memories and future neuromorphic computing. We will provide detailed insights into the status of understanding of these devices as a fundament for their use in the different fields of application. The talks covers the microscopic physics of memristive states and the switching kinetics of VCM devices. It is shown that the switching of all variants of VCM cells relies on the movement of mobile donor ions, which are typically oxygen vacancies or cation interstitials. Emphasis is placed on the extreme nonlinearity of switching kinetics described by physics-based multiscale modeling, ranging from ab initio methods to kinetic Monte Carlo and finite element models to compact models that can be used in circuit simulators. The talk concludes with a treatment of the highly relevant reliability issues and a description of the failure mechanisms, including mutual trade-offs.
1.2.-T1
Nanowire Networks are a novel class of neuromorphic information processing hardware
devices, combining the advantage of synapse-like memristive cross-point junctions and
brain-like complex network topology. In addition to their low operating power, NWNs are
also easy to fabricate and scale up with bottom-up self-assembly. Using a reservoir
computing framework, NWNs have demonstrated the abilities to perform complex learning
tasks such as non-linear transformation, chaotic Mackey-Glass time-series prediction,
MNIST classification and MNIST digit reconstruction using significantly less amount of
training data. Moreover, we also investigated information theoretic metrics of mutual
information (MI), transfer entropy (TE) and active information storage (AIS) to help analyze
the dynamics in the NWNs during learning and optimize the performance. Overall, their
neural-like properties and information processing capabilities make them promising
candidate for neuromorphic computing systems.
1.2.-T2
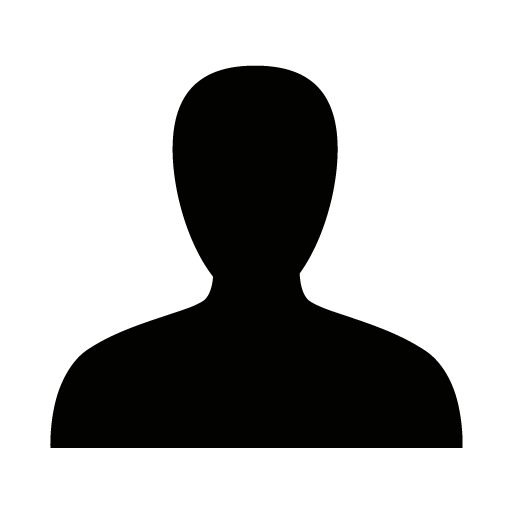
Complex environments generate a large amount of information. This is typically achieved by employing a progressively larger number of sensor nodes and power-draining central processing units to gather and process the temporal input. However, this is inefficient and unscalable in edge computing devices. Unifying the sensing, memory, and computing units is the holy grail of the Internet of Things (IoT). Optimization of edge computing devices for real-time input calls for circumventing the latency arising from data transfer between the components. Unconventional computers, akin to a biological brain, utilise the intrinsic dynamics of physical features in a material substrate to implement systems without a clear distinction between the computational, memory, and sensing units. Therefore, there is a need to innovate in materials to aid or potentially replace the information processing archetype.
Halide perovskites are a class of materials potentially viable for this application. Anomolous effects such as light soaking, JV hysteresis, and slow open circuit voltage decay (OCVD) are the phenomena which establish the theme for their integration in neuromorphic computing.
In this work, the transient OCVD has been probed in a formamidinium lead bromide (FAPbBr3)-based solar cell architecture. Upon stimulation through light pulses, the device shows characteristics of short-term, fading memory with a decay time constant of ~0.3 seconds. Additionally, a non-linear dependence of the paired-pulse facilitation on the number and timing of pulses is observed. The dynamics check all the prerequisites for application as a single-node physical reservoir computing system. Subsequently, slices of spatiotemporal information from a handwritten digit image can be fed into the physical reservoir as sensory inputs. A neural network can then be trained and tested with virtual nodes derived from the compression of these inputs to recognize handwritten digits. Interestingly, due to our device's high distinguishability of a 4-bit slice (with a gain of 200%), the neural network performed on par (at 95% testing accuracy) compared to the baseline. Encouraged by the results, we also simulated and observed a performance trade-off between the testing and validation performances at higher compression.
To process temporal input, physical reservoir computers have been realized in several electrical, magnetic, mechanical, photonic, and optoelectronic systems. However, we introduce a photovoltaic reservoir computing network with photovoltage as the read-out variable. This gives the system an edge over other implementations as voltage output can be directly fed to TFTs, comparators, or ADCs to manifest an entirely physical neural network.
1.2.-T3
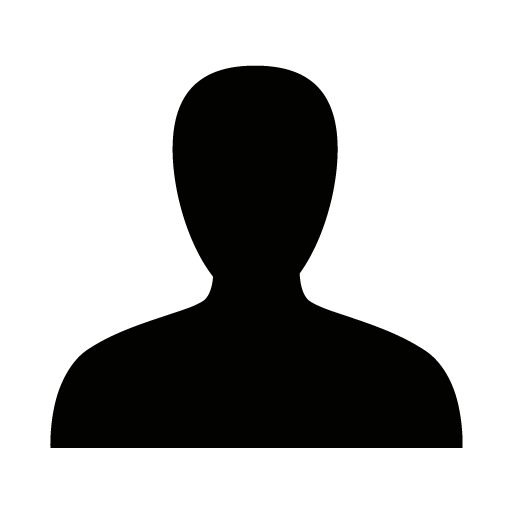
In the recent past, halide perovskites (HPs) have earned fame in the genre of optoelectronics and photovoltaics owing to their excellent optoelectronic properties. Besides optoelectronic traits, HPs also possess a plethora of charge migration pathways, inherent hysteresis, high charge-carrier and ionic mobilities which make them perfect candidates for resistive random access switching memories (RRAMs). However, due to material and electrical instability, the figures-of-merits (FOMs) namely retention, endurance and switching speed were not up to the state of-the-art standard until recently. In order to revolutionize HP Re-RAMs, we devised a novel structure where we replaced the thin-film architecture with vertically aligned high-density HP nanowires rooted in a porous alumina membrane (PAM) sandwiched between silver and aluminum contacts. The excellent passivation provided by the PAM imparted the electrical and material stability to the environmentally fragile HPs by drastically reducing the moisture aided attacks. Data retention time as long as 28.3 years and device endurance of 5 million cycles were obtained along with a switching speed of 100 ps and a down-scaled cell size of 14 nm was achieved. Besides data storage, the HP nanowires were utilized in developing neuromorphic devices possessing low power and high-precision computing functionalities. The nanowire based devices were made to operate in the electro-chemical metallization (ECM) mode and the non-electro-chemical metallization (non-ECM) mode by using silver and indium doped tin oxide as the top electrodes respectively, in order to obtain robust multi-level resistance states. The artificial neural networks tuned by the multi-level states thus obtained, was used to perform image processing tasks of outlining, sharpening and embossing in the ECM mode while the one in the non-ECM mode was utilized to emulate the cognitive learning model of Gestalt Closure Principle. All in all, our nanowire in PAM based architecture, uplifts HP RRAMs to the state-of-the-art standard in various applications concerning data storage and brain-inspired computing.
1.2.-T4
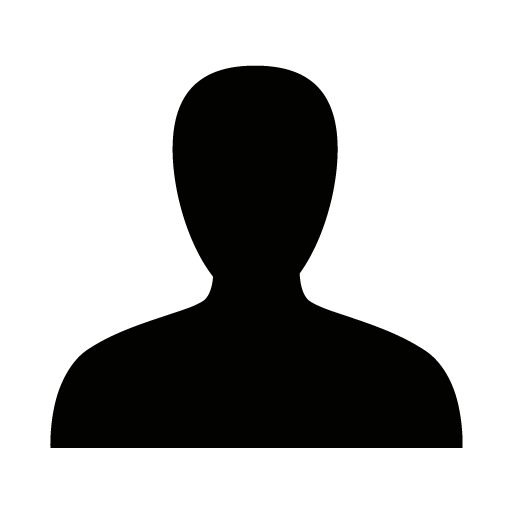
Ferroelectric field-effect transistor (FeFET) is a three-terminal device with a non-volatile property. This device has the functionalities of logic and memory in a single device, which can be used for in-memory computing in non-Von Neumann architectures. HfO2-based ferroelectric materials, especially HfZrO2 (HZO), attract the most attention due to CMOS compatibility and scalability, while it has relatively weak polarization [1]. In order to improve HZO-based FeFET characteristics, it is necessary to enhance HZO ferroelectric properties. However, many works do not take into account the fundamental compromise on dielectric breakdown strength (BDS), transistor ON/OFF current (ION/IOFF) ratio, and memory window (MW) due to the enhanced polarization [2], [3]. In this work, we propose a method of stress memorization based on the thermal expansion mismatch of the TiN/W stacked capping layer in order to control the ferroelectric orthorhombic phase in the HZO layer and the corresponding polarization in optimal value [4]. Our optimized FeFET shows good transistor and memory characteristics with large BDS of ≥4.8 MV/cm, large ION/IOFF ratio of ≥106, large MW of >2 V, and linear potentiation and depression of -0.84 and -2.04.
1.2.-T5
Traditional computation based on von Neumann architecture is limited by the time and energy consumption due to data transfer between the storage and the processing units. The von Neumann architecture is also inefficient in solving unstructured, probabilistic, and real-time problems. To address these challenges, a new brain-inspired neuromorphic computational architecture is required. Due to the absence of resistance-capacitance (RC) delay, high bandwidth and low power consumption, optoelectronic artificial synaptic devices are desirable. However, stable, scalable, and complementary-metal-oxide-semiconductor (CMOS)-compatible synapses that can emulate both inhibitory and excitatory activities have not been demonstrated. This talk presents a work that will overcome this challenge by exploiting the persistence in the photoconductivity of undoped and magnesium-doped scandium nitride (ScN). The negative and positive photoconductivity in undoped and magnesium-doped ScN can be equated to the inhibitory and excitatory synaptic plasticity of the biological synapses, respectively. These artificial optoelectronic synapses can mimic primary functionalities of a biological synapse like short-term memory (STM), long-term memory (LTM), the transition from STM-to-LTM, learning and forgetting cycles, frequency-selective optical filtering, frequency-dependent potentiation and depression, Hebbian learning, and logic gate operation. This work opens up the possibility of utilising a group-III epitaxial semiconducting nitride material with inhibitory and excitatory optoelectronic synaptic functionalities for practical neuromorphic applications.
1.2.-I1
The interface between biological cells and non-biological materials has profound influences on cellular activities, chronic tissue responses, and ultimately the success of medical implants and bioelectronic devices. The optimal coupling between cells and materials is mainly based on surface interaction, electrical communication and sensing.
In the last years, many efforts have been devoted to engineer materials to recapitulate both the environment (i.e., dimensionality, curvature, dynamicity) and the functionalities (i.e., long and short term synaptic plasticity) of the neuronal tissue to ensure a better integration of the bioelectronic platform and cells. In this scenario, resembling also the composition of the neuronal membrane might be beneficial to reconstitute fluidity and proteins’ arrangement (i.e. synaptic receptors) to further optimize the communication between neuronal cells and in vitro bioelectronic platforms2.
Here, we explore how organic electrochemical transistors (OECT) can be used to emulate the operation of neuronal cells as neuromorphic devices and potentially be interfaced with biological cells.
Through the neurotransmitters’ oxidation (i.e., catecholamines) first, we were capable of modulating the synaptic potentiation and ultimately the coupling with biological cells to form a functional synapse. Transitioning from a single to a multiple neurotransmitters modulation, the biohybrid synapses have been also proven to create specific learning patterning and ultimately might be embedded in a feedback-loop control system for adaptive actuation.
In turn, this could represent a first step toward in vitro adaptive neurohybrid interfaces to engineering neuronal networks with biomimetic structural and functional connections at synaptic level.
1.3.-T1
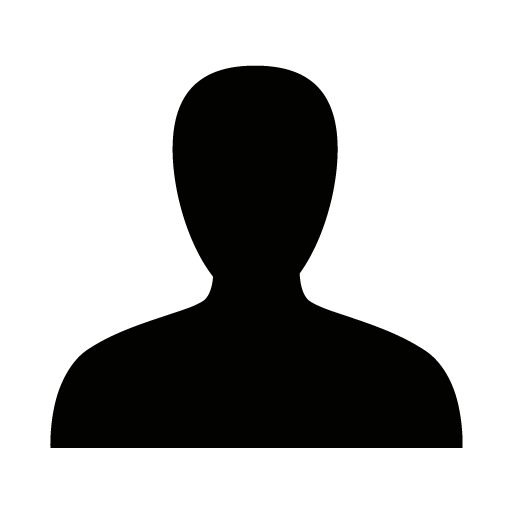
Artificial neural networks (ANNs) with rectified linear units (ReLUs) are standard in solving many artificial intelligence (AI) tasks and pretrained weights are often available. Spiking neural networks (SNNs) offer a potential for energy efficient neuromorphic implementation, however the training of such networks is challenging. In this work we show that fully-connected ReLU and single-spike SNNs are equivalent. Let’s assume that we have pretrained weights and biases of a fully-connected ReLU network with L layers. The real value coming to the input of the ANN is converted to the spiking time which is sent to the spiking neural network implementing a specific non-leaky integrate-and-fire dynamics with linear postsynaptic potential and positive integration bias. Each neuron spikes at time when its membrane potential reaches the predefined threshold after which a very long refractory period is assumed making sure that the neuron stays silent. All neurons that don’t spike before some observation time are forced to spike. The parameters of SNN are set according to our mapping function such that the activity of the neuron in ReLU network can be recovered from the timing of the spike from the corresponding neuron in the SNN. Due to the theoretical equivalence between the two networks it is possible to perform the energy efficient SNN classification of the MNIST dataset with the exact same perfomance as pretrained ReLU network and without any additional training or fine-tuning.
1.3.-T2
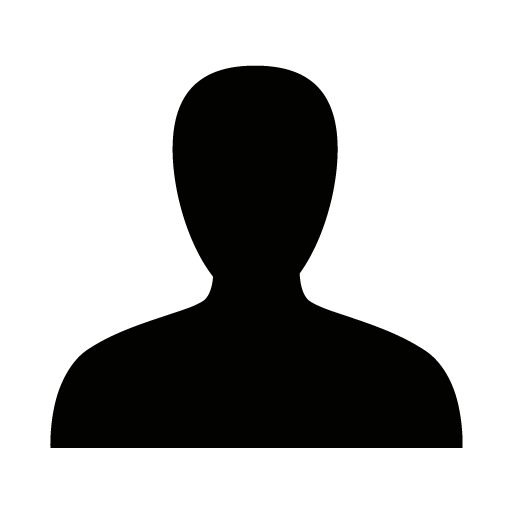
Threshold firing behavior in VO2 neurons is enabled by their Insulator-to-Metal transition (IMT), which can be triggered electrically by applying a voltage across a micro-scaled VO2 resistor. However, akin to biological neurons, the threshold voltage VIMT at which firing occurs varies from one excitation to another, due to inherent stochasticity in the relaxation process [1].
Requirements on the stochastic behavior of VO2 neurons depend on the target application. When used in stochastic neural networks [2], a certain amount of stochasticity is desirable, as it allows to mimic particular behaviors of biological neurons such as probabilistic spiking or stochastic resonance [3][4]. In opposition, when used as sensory neurons, stimulus power is encoded in spike rate [5][6][7][8] (e.g. through modulation of the firing threshold) : then, stochasticity can deteriorate the limit of detection and should thus be minimized. In each type of application, the targeted neuron performance (respectively spiking probability tuning range or sensitivity) is constrained by the inherent stochasticity. However, there has been sparse research regarding which underlying material properties of the VO2 thin film impact the stochastic behavior.
Bridging this gap, our work demonstrates the first experimental evidence that grain size in polycrystalline VO2 films greatly affects the neuron firing-threshold stochasticity. We fabricate VO2 resistors with identical sub-micron dimension, from two sputtered films with 10-fold difference in grain size (obtained through control of annealing temperature). We then compare the variations of their VIMT threshold voltage over 400 cycles of voltage-driven DC sweeps. The small-grain resistor shows remarkably few variations, with a clear Gaussian distribution. The second resistor, whose grain size becomes comparable to its length, has a more pronounced stochastic behavior with a VIMT distribution fitted by a Gaussian mixture, whose main component shows a 4 to 10-fold increase in standard deviation compared to our first device.
We attribute these observations to the filamenting nature of the transition, during which only a subset of grains inside the resistor actually become metallic. The phenomenon percolates from a few “seed” grains which may change from cycle to cycle, yielding different filaments [9][10]. In small-grain devices, these filaments involve larger numbers of grains, averaging out their individual characteristics. In large-grain devices, this number of grains decreases, and their anisotropy yields larger variations of firing threshold.
We thus propose the first design guidelines to significantly tune VO2-neurons stochasticity, by fabricating films with different grain sizes, each best suited for specific neuromorphic applications.
1.3.-T3
Neuromorphic computing is widely regarded as a constraint that increases AI efficiency but trades off proficiency, such as accuracy. However, this separates neuromorphic use cases from mainstream AI, into niches where proficiency is not crucial. To the contrary, our recent work [1-5] shows that neuromorphic mechanisms do not constrain but rather they expand and improve mainstream AI in conventional measures of proficiency, while also improving efficiency. Specifically, we show that neuromorphic algorithms can outperform the state of the art of the broader, conventional machine learning field, beyond neuromorphic niches, in classification accuracy, robustness, inference speed, learning speed, and task reward. We show these in tasks such as keyword spotting, ImageNet classification, playing Atari games, controlling robots, many of which are usually out of reach for neuromorphic models. We test these in settings of online adaptation, supervised, unsupervised, self-supervised or reinforcement learning, meta-learning, and non-backprop-based deep learning. This is achieved by exploiting also other biological mechanisms than spikes. The biological mechanisms that we employ include spikes, Hebbian plasticity, short-term plasticity, and efference copies. The algorithms remain suitable for efficient neuromorphic hardware, and include a new method for decreasing the power consumption of weighting operations in analog synapses. Based on these results, we propose that exploiting the breadth of neuromorphic mechanisms, besides spikes, in suitable applications, is a path towards making neuromorphic the mainstream type of AI computing.
1.3.-I1
In this presentation, we will present the recent progresses on the compute-in-memory (CIM) prototype chips using the resistive random access memory (RRAM) technology. Mixed-signal RRAM based CIM can process the multiply-accumulate (MAC) functions in deep neural networks efficiently using the integrated analog-to-digital converter (ADC), thus it is regarded as a competitive solution for AI hardware design for edge intelligence. In collaboration with TSMC Corporate Research, we taped out two generations of RRAM CIM macros in TSMC 40 nm process. The following features are supported in these prototype chips: 1) Adaptive input sparsity control; 2) Reconfigurable weight precision; 3) Integrated digital compute units; 4) Input-aware on-chip ADC reference; 5) On-chip write-verify controller; 6) Input encoding for embedded security; 7) ADC-less communication between sub-arrays with pulse-width-modulation; 8) In-situ error correction code that preserves the MAC parallelism. Finally, the prospects and challenges of CIM chip design will be summarized.
1.3.-I2
Artificial intelligence applications have demonstrated their enormous potential for computation and processing over the last decade. However, they are mainly based on digital operating principles while being part of an analogue world. They also still lack the efficiency and computing capacity of biological systems. Neuromorphic electronics emulate the analogue information processing of biological nervous systems. Neuromorphic electronics based on organic materials have the ability to emulate efficiently and with fidelity a wide range of bio-inspired functions. A prominent example of a neuromorphic device is based on organic mixed conductors (ionic-electronic). Neuromorphic devices based on organic mixed conductors show volatile, non-volatile and tunable dynamics suitable for the emulation of synaptic plasticity and neuronal functions, and for the mapping of artificial neural networks in physical circuits. Finally, organic neuromorphic circuits enable the local sensorimotor control/learning in robotics as well as more efficient and realistic neuroelectronic interfaces.
1.4.-I1
My group is developing a new paradigm of sensing, computing, storage, and hardware security inspired by the neurobiological architectures and neural algorithms found inside various animal brains that allow evolutionary success in resource constrained environments. Towards the realization of our vision, we exploit unique electronic and optoelectronic properties of layered two dimensional (2D) materials such as graphene, MoS2, WSe2, black phosphorous etc., to design high performance, ultra-low-power, artificially intelligent, and inherently secure solid state devices inspired by natural intelligence. For example, we have mimicked auditory information processing in barn owl (Nature Communications, 10, 3450, 2019), collision avoidance by locust (Nature Electronics, 3, 646–655, 2020), and subthreshold signal detection by paddlefish and cricket using stochastic resonance (Nature Communications, 2020). We have also mimicked probabilistic computing in animal brains using low-power Gaussian synapses (Nature Communications, 10, 4199, 2019), and memristive graphene synapses (Nature Communications, 11, 5474, 2020) and realized biomimetic devices that can emulate neurotransmitter release in chemical synapses (ACS Nano, 11, 3, 2017) and neural encoding in afferent neurons (Nature Communications, 12, 2143, 2021).
1.4.-T1
Although neuromorphic cameras were causally inspired by the retina’s neuromorphology, there is potential to go backwards and try to understand the brain’s processing of visual saliency using event-based data. Visual saliency is the distinct subjective perceptual quality that distinguishes an object from its neighbors and surroundings [1]. Since scientists know that movement, which is captured by photometric silicon retinae, has a huge impact on saliency. Thus, using event-data might be sufficient in tracking a salient object – therefore curbing the need for conventional cameras.
In this talk, we will discuss unsupervised learning methods that exploit the combination of spatial and temporal channels to track salient objects [2]. By using different kernels to induce temporality of spikes, we find that objects can be tracked with accuracy rivaling algorithms deployed on conventional camera data by using several inexpensive distance metrics (e.g. determinant comparison). By then taking the spatiotemporal representation of spikes in pixel neighborhoods, we use three different decision trees to modify tracking templates for object tracking in real-time applications (e.g. drone’s or teletourism.): a relevance tree measuring the difference between the filters, a high-variance tree ensuring generalizability in the filter, and a recency tree measuring how similar the filter is with filters several time-stamps in the past. We show that our results can easily integrate new features without losing old features – representing stability-plasticity, which is necessary for online learning [3].
However, validating these learning models on event-data can be difficult since there is no established ground-truth for saliency. Therefore, we will also present an ongoing experiment to collect eye-tracking data from human participants. The participants are presented event-data captured from imagers like the ATIS and DAVIS cameras. This dataset is to be used as a metric for future visual saliency algorithms written for event-data. If event-based cameras continue to prove their sufficiency in identifying salient objects, this dataset will lay the foundation for future algorithm development.
1.4.-T2
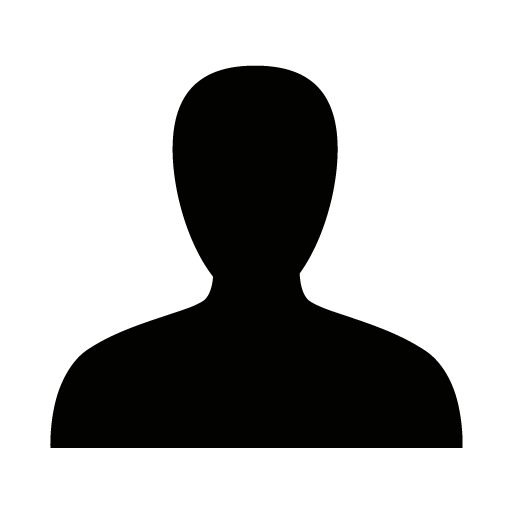
Synapses play a critical role in the computation of the nervous system. The primary function of synapses includes integrating pre-synaptic circuits and converting them into post-synaptic currents, which are scaled by the synaptic weight [1]. Overwhelmingly, bio-inspired neuromorphic architectures are almost exclusively implemented using physically rigid silicon technology [2]. As such, they are limited to a small area, hard to interface with biological tissues, require expensive fabrication equipment, and need high-temperature fabrication processes. Organic electronics are an alternative to conventional materials and electronics [3]. They can be integrated with low-temperature processes with relatively low-priced fabrication equipment over a large area. Organic electronics and materials also offer flexibility, stretchability, and biocompatibility [4].
Here, we report two biologically realistic synaptic models implemented using flexible complimentary organic materials, including organic log-domain integrator [5] and organic differential-pair integrator synaptic circuits [6]. The synapses are shown to convert input voltage spikes into output current traces with biologically realistic time scales. We characterize the circuits’ response based on the synaptic circuit parameters, including gain and weighting voltages, synaptic capacitance, and circuit response due to inputs of different frequencies. The time constant is estimated using the circuit response and compared to the theoretically calculated one. The results show that the theory confirms the experimental measurements. The effect of flexibility is studied over the organic log-domain integrator. The strain shifts the threshold voltage of p and n-type organic field effect transistors and increases the time constant, a parameter critical in learning neural codes and encoding spatiotemporal patterns. Time constants comparable to those of biological synapses, in excess of tens to hundreds of milliseconds, are necessary for future integration with biological neurons and in processing real-world sensory signals such as speech or biosignals. The estimated time constant for the organic log-domain integrator and organic differential-pair integrator reaches 126 and 2000 ms, respectively. The synaptic capacitors are at the level of nano-Farad, while biologically plausible time constants are achieved by deploying smaller synaptic capacitors. Integration of such organic synaptic with organic somatic circuits [7-9] paves the way for future networks of biocompatible artificial organic neurons.
1.4.-T3
While silicon-based neuromorphic circuits (Si-NCs) offer the advantages of small-scale and real-time system-on-chip computing, the complex manufacturing process of Si-NCs often requires expensive high-precision equipment operating in ultra-high temperature and ultra-clean facilities.1 Besides, declining performance of Si-NCs has been reported in applications requiring biocompatibility, physical flexibility, and large area coverage of biological tissues.2–4 As organic counterpart of Si-NCs, the organic neuromorphic circuits (ONCs) consisting of Organic Field Effect Transistors (OFETs) are fast becoming popular due to their unparalleled processing conditions and low manufacturing cost.2,5,6 However, the low reproducibility, low state retention, low temperature stability, high write/read speed and high switching noise of hardware implementations have made the design and fabrication of ONCs unpredictable and inefficient.
We report on a novel modeling approach for analog organic circuits using very simple to customize circuit topology and parameters of individual p- and n-type OFETs.7 Aided with the combination of primitive elements (OFETs, capacitors, resistors), the convoluted behavior of analog ONCs and other general analog organic circuits, can be predicted. The organic log-domain integrator (oLDI8) synaptic circuit, the organic differential-pair integrator (oDPI9) synaptic circuit, and the organic Axon-Hillock (oAH10) somatic circuit are designed and serve as the modular circuit primitives of more complicated ONCs. We first validate our modeling approach by comparing the simulated oDPI and oAH circuit responses to their experimental measurements. Thereafter, the summation effects of the excitatory and inhibitory oDPI circuits in prototyped ONCs are investigated. We also predict the dynamic power dissipation of modular ONCs and show an average power consumption of 2.1 μJ per spike for the oAH soma at a ~1 Hz spiking frequency. Furthermore, we compare our modeling approach with other two representative organic circuit models and prove that our approach outperforms the other two in terms of accuracy and convergence speed. This work pioneers the study of ONC and organic analog circuit modeling and will help improve the design efficiency of larger-scale hardware-level SNNs.
1.4.-T4
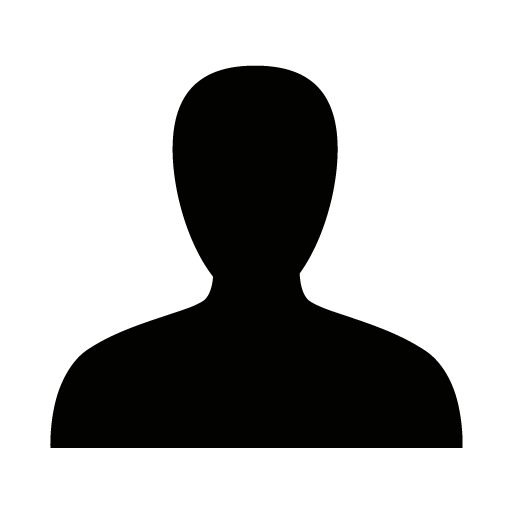
The rate coefficient of an inelastic collision, a.k.a. chemical reaction, can be determined by a non-linear Arrhenius-like mathematical relation. This is mathematically similar to the weights in an artificial neural network (ANN) determined by the activation functions. Therefore, a chemical pathway network (CPN) that massive chemical species are connected by the cause-effect chemical reaction pathways and share a similar mathematical ability with ANN: a functional mapping between the input space and output space. One can thus consider the CPN of material with enough complication of chemical reactions as an ANN and apply the machine learning (ML) technique on it to train, in other words, program the material for specific purposes. This approaches to a new type of intelligent material that is programmable and is different from others on the market that is merely basic logic embedded in the design of material for specific and fixed purposes. The programmability can make the intelligent material hardware that can switch to one of its software to complete a mission alone. In this work, we derived the theoretical general equations to show how a CPN can be a functional of mapping and how to practically program the CPN by finding the optimal parameters such as the temperature and pressure as the weights of the CPN. As an example, to demonstrate this theory, we propose a programmable material intelligence by training a He-air plasma, considering the CPN as an ANN, to play the board game Tic-Tac-Toe. In each turn, the board information is sent to the plasma discharge units by feeding a gas combination, and the plasma returns spectra to show its next move. After training, the plasma shows a significantly high winning rate when playing against a random-move player in a 500-game test. This means that the plasma learned to make logical decisions with strategy. As fewer and fewer move number is required for the plasma to finish its opponent, the decisions of intelligent plasma are more and more aggressive. The plasma finally even concludes that the best defense is a good offense by itself. This work thus reveals the potential of any matter that has a complicated chemical reaction system that can be used as a carrier of artificial intelligence. In other words, a material can be programmed and process data through its own molecular collisions, and thus can be considered an analog computer at the molecular level.
1.4.-T5
Juan Bisquert (pHD Universitat de València, 1991) is a Professor of applied physics at Universitat Jaume I de Castelló, Spain. He is the director of the Institute of Advanced Materials at UJI. He authored 360 peer reviewed papers, and a series of books including . Physics of Solar Cells: Perovskites, Organics, and Photovoltaics Fundamentals (CRC Press). His h-index 95, and is currently a Senior Editor of the Journal of Physical Chemistry Letters. He conducts experimental and theoretical research on materials and devices for production and storage of clean energies. His main topics of interest are materials and processes in perovskite solar cells and solar fuel production. He has developed the application of measurement techniques and physical modeling of nanostructured energy devices, that relate the device operation with the elementary steps that take place at the nanoscale dimension: charge transfer, carrier transport, chemical reaction, etc., especially in the field of impedance spectroscopy, as well as general device models. He has been distinguished in the 2014-2019 list of ISI Highly Cited Researchers.
The dynamic response of metal halide perovskite devices contains a combination of ionic and electronic phenomena and is an excellent model system for the understanding of artificial biomimetic computational elements as neurons and synapses. We present a physical model in terms of delayed recombination current that explains the evolution of impedance spectra and the evolution of current-voltage curves.1-3 We apply the dynamic picture based on a few neuron-like equations to the characterization of halide perovskite solar cells and memristors. A multitude of chemical, biological, and material systems present an inductive behavior that is not electromagnetic in origin. Here, it is termed a chemical inductor.4 We show that the structure of the chemical inductor consists of a two-dimensional system that couples a fast conduction mode and a slowing down element. The impedance spectra announce the type of hysteresis, either regular for capacitive response or inverted hysteresis for inductive response, and provides a new degree of control time domain response of artificial synapses.
(1) Bisquert, J. Hopf bifurcations in electrochemical, neuronal, and semiconductor systems analysis by impedance spectroscopy, Appl. Phys. Rev. 2022, 9, 011318.
(2) Bisquert, J.; Guerrero, A. Dynamic Instability and Time Domain Response of a Model Halide Perovskite Memristor for Artificial Neurons, J. Phys. Chem. Lett. 2022, 13, 3789-3795.
(3) Bisquert, J. Negative inductor effects in nonlinear two-dimensional systems. Oscillatory neurons and memristors, Chemical Physics Reviews 2023, to be published.
(4) Bisquert, J.; Guerrero, A. Chemical Inductor, J. Am. Chem. Soc. 2022, 144, 5996–6009.
1.4.-T6
Corey Lammie is currently employed at IBM Research - Zurich. He completed a PhD in Computer Engineering at James Cook University (JCU) (to be conferred in March, 2023), where he completed his undergraduate degrees in Electrical Engineering (Honours) and Information Technology in 2018.
His main research interests include brain-inspired computing, and the simulation and hardware implementation of Spiking Neural Networks (SNNs) and Artificial Neural Networks (ANNs) using RRAM devices and FPGAs. He has received several awards and fellowships including the intensely competitive 2020-2021 IBM international PhD Fellowship, a Domestic Prestige Research Training Program Scholarship (the highest paid PhD scholarship in Australia), the 2020 Circuits and Systems (CAS) Society Pre-Doctoral Grant, and the 2017 Engineers Australia CN Barton Medal awarded to the best undergraduate engineering thesis at JCU. Corey has served as a reviewer for several IEEE journals and conferences including IEEE Transactions on Circuits and Systems I and II, and the IEEE International Symposium on Circuits and Systems (ISCAS).
During the past two decades, epileptic seizure detection and prediction algorithms have evolved rapidly. However, despite significant performance improvements, their hardware implementation using conventional technologies, such as Complementary Metal-Oxide-Semiconductor (CMOS), in power and area-constrained settings remains a challenging task; espe- cially when many recording channels are used. In this paper, we propose a novel low-latency parallel Convolutional Neural Network (CNN) architecture that has between 2-2,800x fewer network parameters compared to State-Of-The-Art (SOTA) CNN architectures and achieves 5-fold cross validation accuracy of 99.84% for epileptic seizure detection, and 99.01% and 97.54% for epileptic seizure prediction, when evaluated using the Uni- versity of Bonn Electroencephalogram (EEG), CHB-MIT and SWEC-ETHZ seizure datasets, respectively. We subsequently implement our network onto analog crossbar arrays comprising Resistive Random-Access Memory (RRAM) devices, and provide a comprehensive benchmark by simulating, laying out, and determining hardware requirements of the CNN component of our system. To the best of our knowledge, we are the first to parallelize the execution of convolution layer kernels on separate analog crossbars to enable 2 orders of magnitude reduction in latency compared to SOTA hybrid Memristive-CMOS Deep Learning (DL) accelerators. Furthermore, we investigate the ef- fects of non-idealities on our system and investigate Quantization Aware Training (QAT) to mitigate the performance degradation due to low Analog-to-Digital Converter (ADC)/Digital-to-Analog Converter (DAC) resolution. Finally, we propose a stuck weight offsetting methodology to mitigate performance degradation due to stuck RON/ROFF memristor weights, recovering up to 32% accuracy, without requiring retraining. The CNN component of our platform is estimated to consume approximately 2.791W of power while occupying an area of 31.255mm2 in a 22nm FDSOI CMOS process.
1.4.-T7
Dr. Kaoutar El Maghraoui is a principal research scientist at the IBM T.J Watson Research Center and Adjunct Professor of Computer Science at Columbia University. Kaoutar’s work lies at the intersection of systems and artificial intelligence (AI). She leads the AI testbed of IBM Research AI Hardware Center, a global research hub focusing on enabling efficient next-generation accelerators and systems for AI workloads. She is responsible for the open-source development and cloud end user experience for IBM’s emerging Digital and Analog AI accelerators. In 2017, she co-led IBM’s Global Technology Outlook, a key internal strategy effort, where she contributed to creating IBM’s AI vision for the future of IT across global labs and business units. Kaoutar has co-authored several patents, conference, and journal publications in the areas of systems research, distributed systems, high performance computing, and AI. She holds a PhD. degree from Rensselaer Polytechnic Institute, USA, and a bachelor’s and master’s Degrees from Al Akhawayn University, Morocco. She was recognized with several awards including the Robert McNaughton Award for best thesis in computer science, Best of IBM award in 2021, IBM’s Eminence and Excellence award for leadership in increasing Women’s presence in science and technology, several IBM outstanding technical accomplishments, 2021 IEEE TCSVC Women in Service Computing award, and 2022 IBM Technical Corporate award. Kaoutar is the global vice-chair of the Arab Women in Computing organization and active member in many women in science and technology initiatives. She is an ACM Distinguished Member, Senior IEEE Member, and member of Society of Women Engineers, TinyML foundation, and AnitaB.org.
The next step in the evolution of specialized hardware for AI is rooted in addressing the performance efficiency loss from data movement between computational units and memory. This can be achieved through analog in-memory computing which eliminates the Von Neuman bottleneck and allows highly parallel computations directly in memory using memristive crossbar arrays. Although memristive crossbar arrays are a promising future Analog technology for accelerating AI workloads, their inherent noise, and non-idealities demand for improved algorithmic solutions.In this talk, we introduce the IBM Analog Hardware Acceleration Kit [1], a first of a kind open-source toolkit to simulate crossbar arrays from within PyTorch, to conveniently estimate the impact of material properties and non-idealities on the accuracy for arbitrary ANNs (freely available at https://github.com/IBM/aihwkit). This platform allows understanding, evaluating, and experimenting with emerging analog AI accelerators. Our roadmap and capabilities include algorithmic innovations from IBM Research around hardware-aware training, mixed-precision training, advanced analog training optimizers using parallel rank-update in analog, and allowing inference on real research Phase-change memory (PCM)-based analog AI chip prototypes, as well as allowing the research community to extend the toolkit with new devices, analog presets, algorithms, etc. We will show an interactive demo of how the toolkit can be used online though our web front-end cloud composer. The composer provides a set of templates and a no-code experience to introduce the concepts of analog AI, configure experiments, and launch training and inference experiments.
1.4.-K1
J. Joshua Yang is a professor of the Department of Electrical and Computer Engineering at the University of Southern California. He was a professor of the ECE department at the University of Massachusetts Amherst between 2015 and 2020. His current research interest is Post-CMOS hardware for neuromorphic computing, machine learning and artificial intelligence, where he published several pioneering papers and holds 120 granted and about 60 pending US Patents. He is the Founding Chair of the IEEE Neuromorphic Computing Technical Committee, a recipient of the Powell Faculty Research Award and a recipient of UMass distinguished faculty lecturer and UMass Chancellor's Medal. He serves on the Advisory Boards of a number of prime international journals and conferences, including serving as an associate editor of Science Advances. Dr. Yang is a Clarivate™ Highly Cited Researcher in the field of Cross-Field and an IEEE fellow for his contributions to resistive switching materials and devices for nonvolatile memory and neuromorphic computing.
Neural networks based on memristive devices have shown potential in substantially improving throughput and energy efficiency for machine learning and artificial intelligence, especially in edge applications. Because training a neural network model from scratch is very costly, it is impractical to do it individually on billions of memristive neural networks distributed at the edge. A practical approach would be to download the synaptic weights obtained from the cloud training and program them directly into memristors for the commercialization of edge applications. Some post-tuning in memristor conductance may follow afterward or during applications. Therefore, a critical requirement on memristors for neural network applications is a high-precision programming ability to guarantee uniform and accurate performance across a massive number of memristive networks. This translates into the requirement of many distinguishable conductance levels on each memristive device, not just lab-made devices but more importantly, devices fabricated in foundries. High precision memristors also benefit other neural network applications, such as training and scientific computing. Here we report over 2048 conductance levels, the largest number among all types of memories ever reported, achieved with memristors in fully integrated chips with 256x256 memristor arrays monolithically integrated on CMOS circuits in a standard foundry. We have unearthed the underlying physics that previously limited the number of achievable conductance levels in memristors and developed electrical operation protocols to circumvent such limitations. These results reveal insights into the fundamental understanding of the microscopic picture of memristive switching and provide approaches to enabling high-precision memristors for various applications.
2.1.-K1
In-memory computing is emerging as a promising new platform for implementing machine learning, data analytics and artificial intelligence inspired by the human brain. Emerging resistive/memristive memory devices can enable brain-inspired computing primitives, thanks to their tunable volatile/nonvolatile characteristics, their excellent scaling in both 2D and 3D, their ability to run in-memory compute algorithms, and their unique physical properties that can mimic the individual building blocks in the brain, such as neurons, synapses and dendrites. This talk will present the status and challenges about resistive switching devices for in-memory computing. A broad scope of emerging devices will be illustrated, ranging from resistive switching memory (RRAM) and memtransistors based on 2D semiconductors such as MoS2. The prospects for hybrid memristive-CMOS circuits for neuromorphic computing will be discussed in terms of scaling and energy efficiency.
2.1.-I1
Halide perovskites have been under the focus for photovoltaic applications where their power conversion efficiencies have soared to efficiencies exceeding 25%. They have also garnered tremendous research interest over recent years for the development of next-generation light-emitting diodes (PeLED) in optical displays and light sources. The spotlight on this class of semiconducting material stems from several of its enviable traits such as long carrier diffusion length, defect tolerance, high colour purity, and spectral bandgap tunability which spans across the visible and infrared spectrum.
This talk will focus on our efforts on utilising the ionic characteristics of halide perovskites to enable memristive devices that can be employed for neuromorphic applications. The influence of the ionic compositions and interfacial layers in the switching behaviour of the devices would be explained. Going beyond two terminal devices, our recent efforts on three terminal devices would also be covered.
2.1.-I2
Resistive switching technologies like information storage and neuromorphic computation require a high integration density. Hence, studying ultra-small devices with few nanometres in length is important to extract accurate conclusions. However, sometimes patterning small devices is challenging and one good option is to study the electrical properties of the materials at the nanoscale using conductive atomic force microscopy (CAFM). In this seminar, I will explain multiple types of experiments that are useful to characterize the electronic properties of different materials and devices at the nanoscale using CAFM, explaining the different setups that we have developed. I will describe some of the properties I have analysed in metal-oxides, graphene, molybdenum disulphide, hexagonal boron nitride, and nanoparticles.
2.2-T1
Precise and reliable measurement of wind power uncertainty is crucial for the efficient and effective operation of the smart grid. This is because it allows for better economic planning and real-time control of the grid, ensuring a stable and reliable supply of energy. In this paper, a novel spiking neural network (SNN) architecture is proposed as a solution to this problem. SNNs are an alternative to artificial neural networks (ANNs) that encode interneuron communication into temporally-distributed spikes. This allows for a reduction in memory access frequency and data communication, leading to lower computational power requirements for deep learning workloads.
The proposed SNN architecture uses a multi-objective gradient descent (MOGD) algorithm to generate high-quality wind power prediction intervals (PIs). In this algorithm, the SNN is trained to optimize multiple, potentially conflicting objectives simultaneously. The use of MOGD in the proposed SNN architecture makes it well-suited for tasks with multiple, potentially conflicting objectives, such as multimodal data analysis or optimization of multiple objectives.
The use of SNNs is especially important in remote locations where many wind power farms are located. These locations often lack access to reliable cloud services and power supplies, making the reduced computational power requirements of SNNs particularly advantageous. Because SNNs encode interneuron communication into temporally-distributed spikes, they require less memory access and data communication, leading to lower computational power requirements for deep learning workloads. This makes them well-suited for use in remote locations where access to computational resources may be limited.
The proposed SNN architecture is able to achieve comparable performance to its ANN counterpart on complex regression tasks, such as wind power interval prediction. In these tasks, the resulting multi-objective SNN demonstrates superior performance compared to state-of-the-art ANNs. This makes it a promising solution for improving the precision and reliability of wind power uncertainty measurement in the smart grid.
2.2-T2
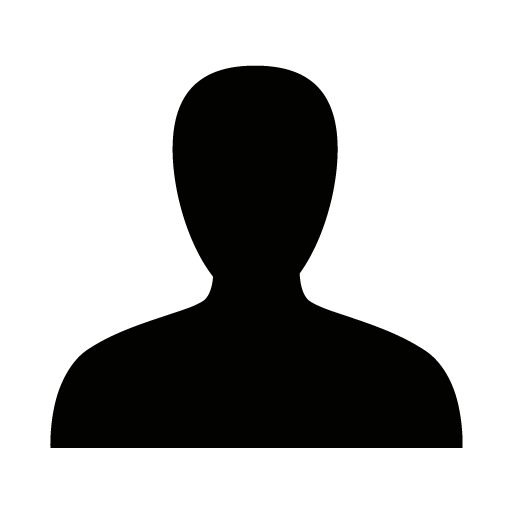
Memory, parallel processing and efficient interconnectivity are key requirements in neuromorphic computing. To emulate the highly distributed nature of biological neural networks, neuromorphic computing utilizes billions of nodes(neurons) and tunable weighted connections(synapses) to perform data-centric tasks such as classification and projection. However, the efficiency of electrical connections relying on physical wires is severely limited by its interconnectivity issues. In contrast, optoelectronically connected systems make use of the fan-out or diffuse nature of light to transmit spikes to multiple receivers in a parallel fashion. While an optoelectronic connection involves both transmitter and receiver nodes, recent optoelectronic approaches only utilize photosensitive memory devices known as the photomemristors to encode the temporal characteristics of the input spikes at the receiver nodes. Light-emitting devices with temporal memory as the transmitter nodes are yet to be explored.
Here, we fabricated a tandem memory LED device comprising of organic and perovskite emitters. Firstly, the memory LED device exhibits temporal memory behaviour with a dynamic gain of 400%. With repeated electrical stimulation, the luminosity of the device increases over time and shown to be highly frequency-dependent. Secondly, the device has dual-color emissions with distinct memory characteristics. Initially, the red organic emitter is first activated due to the small recombination zone spatially located at the organic emitter layer. However, with repeated electrical pulsing, ionic drift in the halide perovskite layer results in the widening of the recombination zone, enabling the green perovskite layer to emit. We first studied the widening of the recombination zone; both the electroluminescence and photoluminescence spectra of the bilayer stack are compared. Interestingly, the photoluminescence spectra did not exhibit any emission from the red organic emitter. This highly suggests that the color change is not due to reabsorption but a spatial shift in recombination zone. Finally, we showcase two use cases in optoelectronic neuromorphic computing. An array of tandem memory LEDs was experimentally utilized as a neuromorphic pre-processor to enhance contrast of noisy digits by 3.81x and maintain the recognition accuracy (from -30% to only -1.46% drop). Additionally, we also demonstrated experimentally the inhibition of return mechanism by emulating heterogeneous synaptic connections in a Winner-takes-all circuit. The inhibition of return mechanism is a pivotal functionality in regulating visual attention via thorough scanning of visual field for multiple salient cues. Through these demonstrations, we highlight the essence of utilizing the dual-color tandem memory LED as the dynamic node for optoelectronic neuromorphic pre-processing.
2.2-T3
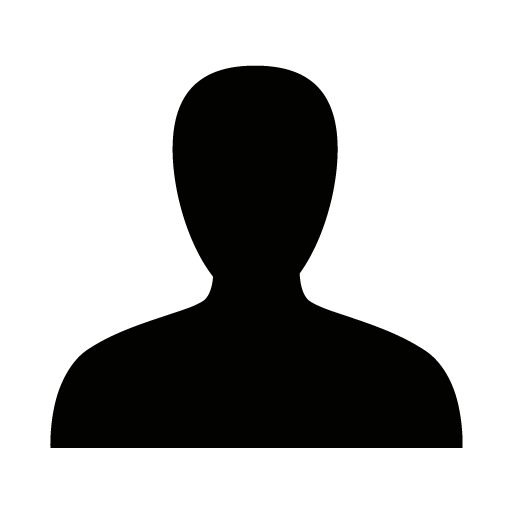
Reservoir computing is noted for its low-training-cost capability in temporal signals processing [1,2]. Thus, hardware implementations tailored to requirements in reservoir computing would profoundly improve data processing in edge computing where the energy budget and bandwidth is strictly limited. In this work, we report a Hf0.5Zr0.5O2-based three-terminal ferroelectric memcapacitor (FMC) with nonideal depolarization dynamics for multi-sensory information processing physical reservoir computing (RC) system. Our RC system is verified by standard benchmarking tasks with high accuracy, and the power consumption (~2.4 nW per reservoir state) outperform most hardware and software-based RC systems. Furthermore, such physical RCs are capable of recognizing sensory information including graphic, acoustic, electrophysiological, and mechanic modalities. As proof-of-concept, a touchless user interface (TUI) for virtual shopping is demonstrated using FMC-based RC systems which can identify purchasing operations based on acoustic and electrophysiological commands. Our design would shed light on the development of high energy-efficiency edge computing apparatus.
2.2-T4
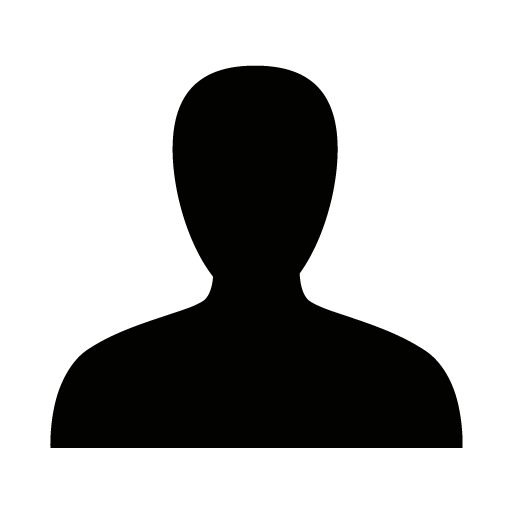
Advancing the memristor concepts is one of the primary topics in neuromorphic computing. Among the main routes in this direction is development of bio-realistic dynamical memristors, i.e. those that mimic multiple modes of biological behavior. This is expected to boost the performance in such tasks as spatio-temporal pattern recognition due to encapsulated transient operation, which is close to the behavior of the biological counterparts in visual cortex. The concept of second order memristor is a successor of the classical memristor concepts that allows to realize the short-term synaptic dynamics and its influence on the long-term synaptic modification. Here we present the second order memristor based on La0.7Sr0.3MnO3 / BaTiO3 / La0.7Sr0.3MnO3 – an all-oxide multiferroic tunnel junction [1]. We show the ability of analog resistance modulation with voltage pulses, together with an inherent feature of short-term memory. Based on this, we demonstrate the emulation of multiple synaptic learning behaviors: short and long-term potentiation / depression, paired-pulse facilitation / depression, spike rate- and experience-dependent plasticity with the sliding threshold frequency effect, in accordance with the Bienenstock-Cooper-Munro theory. The long-term memory effect is explained on the basis of reversible redox reaction at La0.7Sr0.3MnO3 / BaTiO3 interfaces, which modifies the ratio of Mn3+ / Mn4+ ions, responsible for the conduction in these regions and in the overall device. And the short-term behavior is attributed to the voltage-induced transient dynamics of oxygen vacancy distribution and dielectric polarization. We believe that the demonstrated second order memristor concept deepens the understanding of dynamical behavior in neuromorphic devices and may be of use in development of hardware artificial neural networks.
2.2-T5
With the unprecedented rise of halide perovskites in the field of photovoltaic, ion migration comes out as a bane that results in unfavorable I-V characteristics in perovskite solar cells and has been a major drawback towards their physical implementation. Recently this unwelcomed trait is being exploited to unlock new opportunities, especially in the field of memristors for information storage and computation. This report introduces lead-free all-inorganic Cs3Sb2I9 for realizing multifunctional memristive behavior using its two polymorphs (dimer and layered). The dimer-based devices show an initial low-resistive state along with a self-compiling nature that brings it to the high resistive state under suitable bias due to the self-formed metallic bridges. The devices based on the layered phase, in contrast, are initially in a high state and thereby require a voltage to switch to a low-resistive one and are found to obtain better performance reliability and environmental stability. A closed-loop pulsed switching (CLPS) measurement protocol has been employed to further increase the devices' endurance by passively controlling the make and break of the conducting bridges. Finally, interface-modified devices have exhibited multiple functionalities of the human brain, such as excitatory/inhibitory postsynaptic currents and spike voltage/duration/timing dependent plasticity, the transition from short-term memory to long-term memory, and potentiation/depression behavior with extremely low energy consumption per synaptic event. The device characteristics have also followed the Ebbinghaus curve of forgetting and the Atkinson–Shiffrin memory model applicable to human brains. This study hence concludes that the introduction of lead-free all inorganic Cs3Sb2I9 as a memory device shows better promise for multifunctional memory elements using different polymorphs and devices structure and will pave the way towards realizing energy-efficient artificial synaptic functions for next-generation memory applications.
2.2-T6
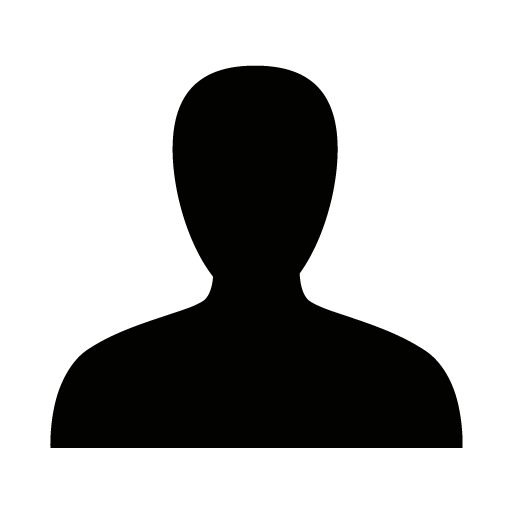
Novel computing systems are currently being explored to overcome the memory bottleneck of computational systems based on the von Neumann architecture. In this context, brain-inspired paradigms implemented with memristive devices are promising candidates demonstrating low energy consumption, high scalability, large parallelism, and intrinsic high error tolerance. However, these systems are mainly limited to the emulation of relatively simple biological primitives due to the first-order dynamics of the memristive element. Typical solutions to implement higher-order neuromorphic functions require increasingly complex circuits that simulate the brain functioning, thus requiring additional computation outside the memristor device and losing the advantages in terms of area and complexity.
Here we present second-order dynamics in halide perovskite memristors which are intrinsically enabled by mixed ionic-electronic conduction. The second-order dynamics arises from the interplay between ion migration, back-diffusion, and modulable Schottky barriers. Second-order memistor dynamics allows for both rate and timing-dependent plasticity without the need for overlapping pulses, thus paving the way for a general methodology to develop neuromorphic memristive circuits with high spiking parallelism and low power consumption. By using a triplet-spike-timing-dependent-plasticity scheme, we demonstrate the implementation of the Bienenstock-Cooper-Munro learning rule. By taking inspiration from the visual processing in cortical neurons network, we implement unsupervised orientation-selective learning for binocular inputs in halide perovskite memdiodes network. these results support halide perovskite as key enabling material for neuromorphic engineering able to mimic the bioneurological phenomena of the brain by device physics.
2.2-T7
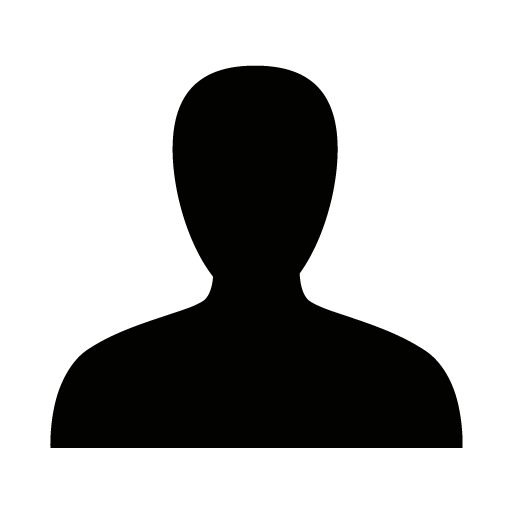
Simulation frameworks such MemTorch [1] [2], DNN+NeuroSim [3] [4], and aihwkit [5] are commonly used to facilitate the end-to-end co-design of memristive machine learning (ML) accelerators. These simulators can take device nonidealities into account and are integrated with modern ML frameworks. However, memristors in these simulators are modeled with either lookup tables or simple analytic models with basic nonlinearities. These simple models are unable to capture certain performance critical aspects of device nonidealities. For example, they ignore the physical cause of switching, which induces errors in switching timings and thus incorrect estimations of conductance states. This work aims at bringing physical dynamics into consideration to model nonidealities while being compatible with GPU accelerators. We focus on Valence Change Memory (VCM) cells, where the switching nonlinearity and SET/RESET asymmetry relate tightly with the thermal resistance, ion mobility, Schottky barrier height, parasitic resistance, and other effects [6]. The resulting dynamics require solving an ODE that captures changes in oxygen vacancies. We modified a physics-derived SPICE-level VCM model [7] [8], integrated it with the aihwkit [5] simulator and tested the performance with the MNIST dataset. Results show that noise that disrupts the SET/RESET matching affects network performance the most. This work serves as a tool for evaluating how physical dynamics in memristive devices affect neural network accuracy and can be used to guide the development of future integrated devices.
2.3-T1
Spiking neural networks (SNNs) have the potential to greatly reduce memory and energy consumption compared to traditional neural networks. Inspired by the efficiency of the human brain, SNNs incorporate temporal and neuronal sparsity, which can be exploited by next-generation neuromorphic hardware.
To explore the potential of SNNs in engineering applications, where regression tasks are common, we introduce a framework for regression using SNNs in the context of continuum mechanics. However, the nature of SNNs presents a challenge for regression tasks, which are frequently encountered in engineering sciences. To address this challenge, we propose a network topology that uses the membrane potential of spiking neurons to decode binary spike trains into real numbers.
We apply our framework to history-dependent material models, fitting SNNs to data that describes the behavior of materials over time. This type of model can be used to model materials that are subjected to complex loading histories, such as materials that are stressed beyond their reversibility point. We use nonlinear regression to fit the model to data that describes the time-dependent behavior of these materials, allowing for accurate predictions about the material's response to different loading conditions.
We derive several different spiking neural architectures, ranging from simple spiking feed-forward networks to complex spiking long short-term memory networks. We conduct numerical experiments to evaluate our framework's ability to perform regression on linear and nonlinear, history-dependent material models. SNNs are a natural fit for modeling history-dependent materials, and we demonstrate their ability to accurately model materials that are stressed beyond reversibility. A comparison with traditional neural networks shows that our proposed framework is more efficient while maintaining precision and generalizability. All code has been made publicly available to support reproducibility and encourage further development in this field.
2.3-T2
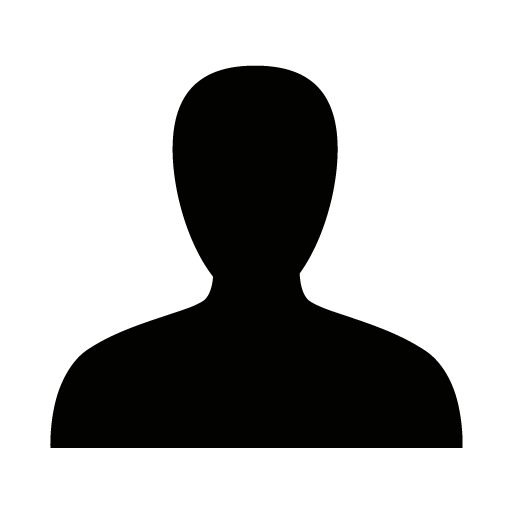
In the last twenty years, a broad range of emerging memory technologies have been proposed as storage class memory (SCM) toward bridging the gap between high-performance memory and low-cost storage devices. Novel memory devices are also essential for developing low power, fast and accurate in-memory computing and neuromorphic engineering concepts that can compete with the conventional CMOS digital processors. 2D semiconductors, such as transition metal dichalcogenides (TMDs), provide a novel platform for advanced semiconductors, thanks to their atomic-scale thickness and their strong potential for 3D integration [1]. We present a three-terminal device called memtransistor, based on multilayer MoS2 with ultra-short channel length. Depending on the channel length (Lchannel) and the physics mechanism exploited, the device can be used as a memory [2] or as a powerful computing device [3,4]. When the distance between the two drain and source terminals is below 50 nm, the device exhibits a switching characteristic thanks to the Ag cation migration between the two electrodes. This device is called ion-based memtransistor to distinguish it from the electron-based memtransistor in which charge trapping is exploited to obtain an analog synaptic characteristic. The last device has a longer channel to avoid the ion migration between source and drain terminals. Thanks to the switching characteristic with independent control of the two resistive states, and the three-terminal structure, the ion-based memtransistor has been used in a chain-type memory array architecture where the individual memory devices along the chain can be selected for write and read [2]. This experiment paves the way for high-density, 3D memories based on 2D semiconductors. The charge trapping is instead exploited to obtain the synaptic characteristics with the electron-based memtransistor [4]. Synaptic potentiation/depression are obtained moving the device threshold and analogically changing the channel conductance. The linearity of the characteristic, the low power operation and the scalability offered by the 2D semiconductor makes the device extremely promising for the implementation of hardware neural network accelerators [3]. In addition, the dynamic characteristic of the device is exploited for the realization of the reservoir in a reservoir computing (RC) system for image recognition. The results make the memtransistors a promising technology for future high-density neuromorphic computing concepts.
2.3-T3
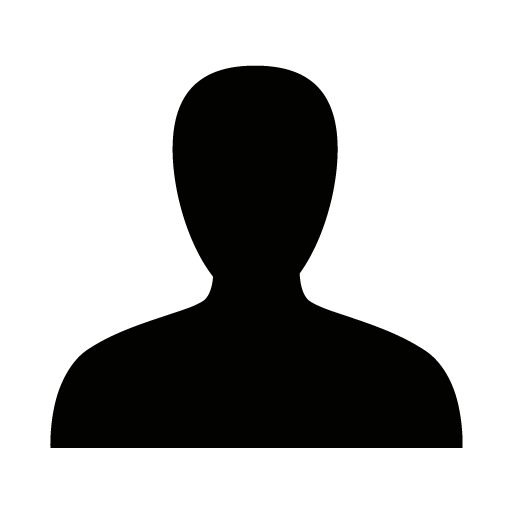
Research advancement in brain-machine interfaces, implantable/wearable devices, prosthetics, and intelligent soft robotics calls for close interaction between technology and nature. Since the fundamental building elements of life differ significantly from those utilized in electronic devices, the ability to link an artificial device with a biological system is crucial to the success of these domains. Neuromorphic systems that borrow design concepts from biological systems promise to bridge this gap. Although several software-based neuromorphic algorithms have been integrated into biomedical systems, hardware-based systems that can directly interface with living tissue, adapt based on biological feedback, and utilise event-based sensing and processing capabilities of biological systems are ultimately necessary. However, circuits and devices made of silicon (Si), commonly used in hardware neural networks and neural interfaces, suffer from several drawbacks such as rigidity, lack of biocompatibility, the requirement for numerous circuit elements, and operation mechanisms that are fundamentally different from biological systems, making bio-integration difficult. Hence in this work, I will present Organic Electrochemical transistor-based artificial neurons which can be modulated by ions and exhibit capability for bio integration and feedback. Two diffferent neuron models will be discussed – a simple leaky integrate and fire (LIF) neuron and a conductance-based biorealistic neuron. While the LIF neuon is based on a complementary organic electrochemical transistor-based circuit, the biorealistic neuron utilises antiambipolar behaviour in ladder-type poly(benzimidazobenzophenanthroline) (BBL). The biorealistic neuron can spike at frequencies nearing 100 Hz, emulate most critical neural features, demostrate stochastic spiking, and enable neurotransmitter-/amino acid-/ion-based spiking modulation, which is then used to stimulate biological nerves. These combined features are impossible to achieve using previous technologies.
2.3-I1
Neuromorphic engineering is inspired by the efficiency of the brain and focusses on how to emulate and utilise its functionality in hardware. Organic electronic materials have the potential to operate at the interface with biology and offer promising solutions for the manipulation and the processing of biological signals with potential applications ranging from efficient artificial intelligence systems and bioinformatics to brain-computer-interfaces and smart robotics.
This talk describes state-of-the-art organic neuromorphic devices and provides an overview of the current challenges in the field and attempts to address them. I demonstrate two device concepts based on novel organic mixed-ionic electronic materials and show how we can use these devices in trainable biosensors and smart autonomous robotics.
Next to that, organic electronic materials have the potential to operate at the interface with biology. This can pave the way for novel architectures with bio-inspired features, offering promising solutions for the manipulation and the processing of biological signals and potential applications ranging from brain-computer-interfaces to bioinformatics and neurotransmitter-mediated adaptive sensing. I will highlight our recent efforts for such hybrid biological memory devices.
2.3-I2
Neuromorphic computing is seeing a resurgence as Si scaling strategies offer diminished returns. Software leads the way in implementing intelligence noted in animal brains into artificial neural network algorithms and learning rules. Simultaneously, there is interest in examining how such features can be emulated in hardware and whether this can offer some practical advantages in realizing low power computers in general or computers that can perform certain tasks more efficiently. The focus of my presentation will be on metal-insulator transitions in quantum materials such as correlated perovskite semiconductors that can be used to emulate the characteristics of neurons and synapses in the brain and serve as building blocks for AI hardware. We will then discuss how features of intelligence noted in various insects, mammals and birds by biologists can be implemented in electronic devices and circuits for learning and decision-making. Fundamental microscopic mechanisms essential to understanding the operational principles of such devices will be discussed using combination of synchrotron scattering studies and first principles modeling. Connections to the early research on spin glasses for neural networks will be discussed. Collaborations and funding will be acknowledged in the presentation.
2.4-I1
Prof. T. Venkatesan is currently the Director of the Center for Quantum Research and Technology (Professor of Physics and ECE) at University of Oklahoma, and Scientific affiliate at NIST Gaithersburg. He is also the founding Director of the Center of Optimal Materials for Emerging Technologies (COMET) at OU. Prior to this he was Director of the Nano Institute at the National University of Singapore (NUSNNI) where he was a Professor of ECE, Physics, MSE and NGS. He wore various hats at Bell Labs and Bellcore before becoming a Professor at University of Maryland. As the inventor of the pulsed laser deposition (PLD) process, he has over 800 papers and 34 patents and is globally among the top one hundred physicists (ranked at 66 in 2000) in terms of his citations (Over 51,186 with a hirsch Index of 115-Google Scholar). He has graduated over 56 PhDs, 35 Post Docs and over 35 undergraduates. He is also the founder and Chairman of Neocera, and Neocera Magma, companies specializing in PLD and magnetic field imaging systems and co-founder of Blue Wave Semiconductors. He recently helped launch two healthcare companies in Singapore, Cellivate and Breathonix. Close to 12 of the researchers (PhD students and Post Docs) under him have become entrepreneurs starting over 25 different commercial enterprises.
He is a Fellow of the Royal Society (FRS), National Academy of Inventors (USA), Singapore National Academy of Science, Asia-Pacific Artificial Intelligence Academy, World Innovation Foundation, American Physical Society (APS), Materials Research Society (MRS), Academician of the Asia Pacific Academy of Materials, Fellow of the winner of the Bellcore Award of excellence, George E. Pake Prize awarded by APS (2012), Distinguished Lectureship on the Applications of Physics Award- APS (2020), President’s gold medal of the Institute of Physics, Singapore, Guest Professor at Tsinghua University, past member of the Physics Policy Committee (Washington DC), the Board of Visitors at UMD and the Chairman, Forum of Industry and Applications of Physics at APS. He was awarded the outstanding alumnus award from two Indian Institute of Technologies- Kanpur (2015) and Kharagpur (2016), India.
Artificial intelligence (AI) has been heralded as the flagbearer of the fourth industrial revolution but comes with a cost and that is computing power. It is projected that by 2040, we will need more computing energy than the total energy we can produce now. So, we need devices that can offer higher computing/ storage density with low energy consumption like neurons. We are addressing these challenges using oxide and molecular-electronic based memristors, which enable us to overcome the von Neuman bottleneck by co-locating the memory and computing functions on the same device, as in neuromorphic computing.
I will discuss a variety of strategies for forming oxide based memristors using different phenomena- band filling and creating a quasi-conduction band, using oxygen vacancies to create conductive percolation, using metal-insulator transitions, or using asymmetric tunneling at a ferroelectric barrier. The relative merits of the various approaches will be detailed.
In addition, I will touch upon memristive devices and circuits made from an azo-aromatic complex with extra-ordinary reproducibility, robustness, and scalability. These devices have been shown to switch with energies approaching atto-joules with measured switching times shorter than 5 ns. By using a simple cross bar array, we have shown that these devices perform with an energy-speed product 5000 times that of a state-of-the-art CMOS circuit.
1. Sreetosh Goswami*, Rajib Pramanick, Abhijeet Patra, Santi Prasad Rath, Ariando, Damien Thomson, T. Venkatesan*, Sreebrata Goswami* and R Stanley Williams*, Decision Trees within a Molecular Memristor, Nature, Sep. 3, 2021
2. Sreetosh Goswami, Adam J. Matula, Santi P. Rath, Svante Hedstrom, Surajit Saha Meenakshi Annamalai, Debabrata Sengupta, Abhijeet Patra, Siddhartha Ghosh, Hariom Jani, Soumya Sarkar, Mallikarjuna Rao Motapothula, Christian A. Nijhuis, Jens Martin, Sreebrata Goswami*, Victor S. Batista*, T. Venkatesan*, Robust resistive memory devices using solution-processable metal-coordinated azo-aromatics, Nature Materials, 16, 1216 (2017). News and views on this article: I. Valev and M. Kozicki, Non-volatile Memories: Organic Memristors come of age, Nature Materials, 16, 1170 (2017)
2.4-T3
Antonio Guerrero is Associate Professor in Applied Physics at the Institute of Advanced Materials (Spain). His background includes synthesis of organic and inorganic materials (PhD in Chemistry). He worked 4 years at Cambridge Dispaly Technology fabricating materiales for organic light emitting diodes and joined University Jaume I in 2010 to lead the fabrication laboratory of electronic devices. His expertise includes chemical and electrical characterization of several types of electronic devices. In the last years he has focused in solar cells, memristors, electrochemical cells and batteries.
Halide perovskite materials are mixed electronic and ionic conductors that find use in several applications. The ionic conductivity is responsible for a the memory effect that leads to hysteresis in the solar cells configuration.1 Here we explain how this ion migration can be used to our advantage to promote formation of conductive and insulating states making them useful as resistive memories (memristors). We show that the working mechanism and performance of the memory devices can be tuned and improved by a careful selection of each structural layer. Several configurations are evaluated in which structural layers are modified systematically: formulation of the perovskite2, the nature of the buffer layer3 and the nature of the metal contact4. In addition, we develop an electrical model to account for the observed j-V response.5 Overall, we provide solid understanding on the operational mechanism of halide perovskite memristors that unveils the connection between electronic and ionic conduction.
2.4-T4
I studied Computer Science (ETH Zürich) and Neural Systems and Computation (Univ. of Zürich and ETH Zürich). I used to work with spiking neural networks until joining the in-memory computing group of Abu Sebastian at IBM Research in Zurich. My main interests are the robustness of networks to various sources of noise, adversarial attacks deployed on hardware, and bridging the performance gap between GPUs and analog in-memory computing w.r.t. inference accurcay.
The precise programming of crossbar arrays of unit cells is crucial for obtaining high matrix-vector-multiplication (MVM) accuracy in analog in-memory computing (AIMC) cores. We propose a radically different approach based on directly minimizing the MVM error using gradient descent with synthetic random input data. Our method significantly reduces the MVM error compared with conventional unit cell by unit cell iterative programming. It also eliminates the need for high-resolution analog-to-digital converters (ADCs) to read the small unit-cell conductance during programming. Our method improves the experimental inference accuracy of ResNet-9 implemented on two phase-change memory (PCM)-based AIMC cores by 1.26%.
2.4-T5
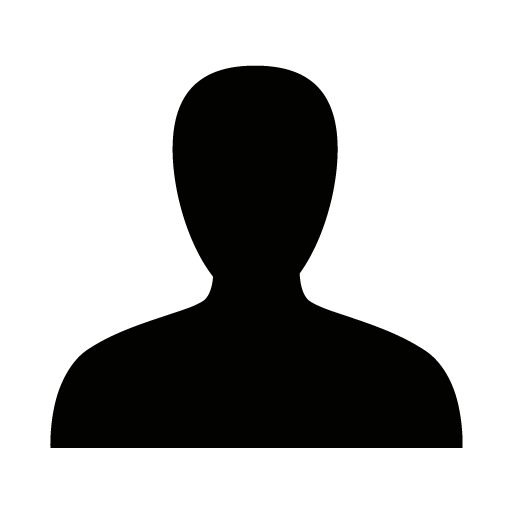
Synaptic plasticity is at the base of learning and memory capabilities of the human brain and lately recent studies reported a strong correlation between synaptic dysfunction and neurodegenerative diseases. Unfortunately, the complexity of the brain and the nervous system prevents the investigation of mechanisms underlying cognitive impairment, and for this reason the possibility to inhibit neurodegeneration at the early stage of disease is still far from being concrete1. In this scenario, in vitro platforms have attracted significant interest as they could provide simplified biomimetic models of neuronal systems and allow for the investigation of synaptic dysfunctions in neurodegeneration. In this context, organic electrochemical transistors (OECTs) have recently emerged as neuromorphic devices that exhibit synaptic plasticity2 and dopamine-mediated adaptive behavior as biological neuronal cells3. Furthermore, the recent implementation of supported lipid bilayers (SLBs) on conductive polymers and OECTs lead to the development of a new class of biosensors able to mimic cells native environment4. Here, we present a biomimetic in vitro platform which displays a neuronal membrane outer architecture and is able to recapitulate the same functionalities of neurons (i.e. neurotransmitter-mediated synaptic plasticity). In detail, we investigated the role of SLB ionic barrier behavior on the neuromorphic functionalities of the OECT: here, we evaluated the role of a full homogeneous covering of OECT gate and channel (SLB-full configuration) vs the specific confinement of the lipid bilayer on the OECT channel only (SLB-one side configuration)5. Furthermore, combining the oxidation of dopamine at the gate electrode with the SLB-one side neuromorphic device, we accomplished an in vitro platform which mimic pre and post-synaptic neuronal architecture as well as synaptic functioning. We expect that such biomembrane-based organic neuromorphic transistor could represent a first step towards the implementation of fully biomimetic in vitro systems, which resemble composition and functionalities of neuronal networks and as such, could contribute to unwind the complex mechanisms underlying neurodegeneration and synaptic plasticity loss.
2.4-I2
Although artificial deep neural networks promise revolutionary performance advantages on data-heavy and intractable workloads, their pre-deployment training on present digital hardware is already energy-limited and economics-limited. Prevailing backpropagation training algorithms are generally incompatible with emerging post-digital hardware because of mismatches between the requirement for exact calculations and the inherent imprecision of analog hardware, thereby needing innovations and co-design at all layers of a computing stack. Here, we introduce a biologically plausible activity-difference-based training demonstrated on co-designed integrated analog memristor Hopfield networks - memristor activity-difference energy minimization (MADEM). MADEM treats the network parameters as a constrained optimization problem, and numerically calculates local gradients using behavioral differences in the same hardware targeted by the training. Thus, MADEM is highly suited for and exploits analog hardware, and promises over four orders of magnitude energy advantages compared to digital approaches for scaled up problem sizes.
2.4-I3
Analog non-volatile memory (NVM)-based accelerators for Deep Neural Networks (DNNs) can achieve high-throughput and energy-efficient multiply-accumulate (MAC) operations by taking advantage of massively parallelized analog compute, implemented with Ohm’s law and Kirchhoff’s current law on arrays of resistive memory devices. Competitive end-to-end DNN accuracies can be obtained if weights are accurately programmed onto NVM devices and MAC operations are sufficiently linear.
This presentation discusses the origin of this opportunity as well as the challenges inherent in delivering on it. We briefly discuss materials and devices for analog volatile and non-volatile memory, and circuit and architecture choices and challenges, before describing a 14-nm inference chip consisting of multiple 512x512 tiles (crossbar arrays) of Phase Change Memory (PCM) devices. Multiple DNN benchmarks are implemented using a circuit-switched 2D mesh for massively-parallel data-transport between the tiles.
2.4-T1
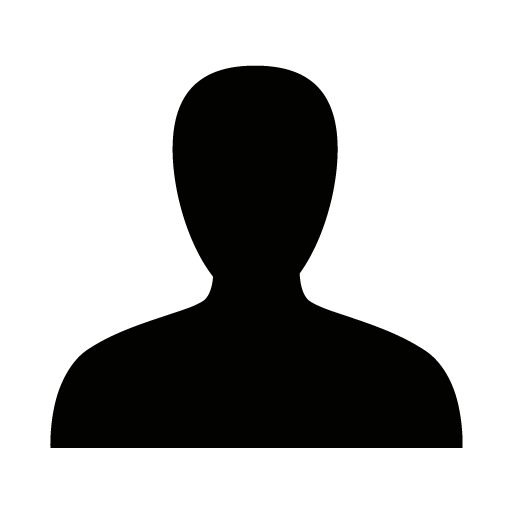
Light and its interaction with physical matter plays a crucial role in several processes that are at the base of the life on Earth[1]. Such interaction was deeply studied and exploited in bioelectronics allowing for several application like biosensing[2] and visual-pathway emulation[3]. In humans, light stimulus is transduced in the retina and directly sent to the brain as spiking signals[4].
In this context, light-driven neuromorphic devices were demonstrated, offering advantages of optical systems, such as negligible signal delay, no power loss and wide bandwidth[5]. On the other hand, such devices rely on metal oxides and photovoltaic materials, crucially impeding a their usage in biointerfaces and preventing from their application[6] in case of loss of vision or other sight-related pathologies .
In light of this, we demonstrate a fully organic photo electrochemical transistor (OPECT) able to conjugate light-induced and voltage-controlled neuromorphic features, while also exploiting key features of conducting polymers (CPs), as biocompatibility and stability in aqueous environment[6]. In addition, the presented platform allows for multimodal signal integration, combining different stimuli independently.
A photoresponsive PEDOT:PSS covalently bonded to azobenzenes moieties (azo-tz-PEDOT:PSS) is synthesized though an ad hoc “click” reaction and used as gate electrode in planar-gated depletion-mode OPECT (azo-OECT). Here, by shining UV light on the gate a charge transfer/trapping mechanisms is induced, light-modulating the conductance of the transistor. Firstly, the conductance modulation is exploited to prove the biomimetic potential of the azo-OECT, by implementing a fully organic OFF vertical pathway of the human retina.
Subsequently, by exploiting the multimodal signal processing capability of the azo-OECT, neuromorphic features are investigated. By applying positive voltage pulses at the gate terminal, it is in fact possible to elicit a transient conductance modulation of the azo-OECT, that is reversed after few seconds, mirroring the short-term synaptic plasticity (STP). On the other hand, when the gate electrode is lit by light pulses, a stable conductance modulation is observed, mimicking long-term synaptic plasticity (LTP) and proving learning capabilities. The opposite reaction (forgetting) is then induced by applying negative voltage pulses at the gate terminal.
Ultimately, the combination of all the input signals allows the azo-OECT to learn sensory information following Atkinson-Shiffrin memory model[7].
The neuromorphic features demonstrated in azo-OECTs, along with intrinsic properties of CPs, are what makes the proposed architecture an ideal candidate for a neurohybrid interface, in which the artificial neuromorphic device could cooperate with biological circuitry, independently computing biological, electrical and optical signals.
2.4-T2
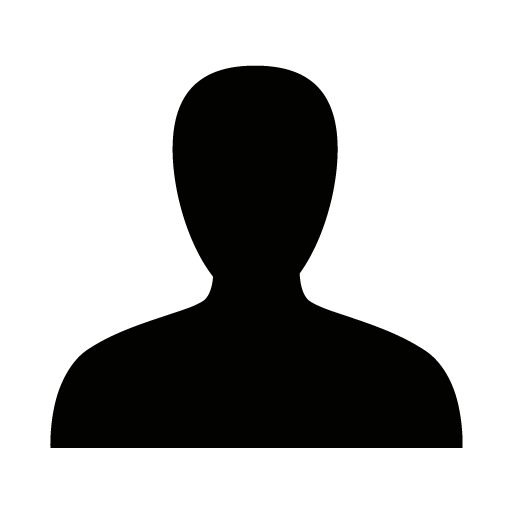
Next generation body-machine interfaces are attractive for both healthcare applications and consumer products. However, interfacing with biology requires electronic hardware with 1) excellent mechanical compliance, 2) operational stability in physiological environments, 3) reliable harnessing, discerning, amplification, and transduction of physiological information, and 4) ability to adapt or learn from physiological surroundings and execute tasks accordingly. For this highly demanding task, iono-electronics, where a semiconductor responds to incoming ions and undergoes a property change (electronic, optical, mechanical) which is in turn detected or utilized for a specific application are promising candidates. Though conjugated polymers have shown to be ideal conductors for such applications, only a few mixed conductors are studied to date and a one-material-fits-all bottleneck continues to hamper rapid advancements. The challenge lies in the lack of a cross-disciplinary design approach of high-performance materials. Establishing the needed balance between the two antagonistic modes of transport (ion permeability and electronic charge transport) to yield high performance devices has not only become an exciting field of fundamental research, but also a conduit towards advanced electronics. My lab at MIT (the laboratory of organic materials for smart electronics, OMSE Lab) employs molecular design to meet performance requirements in polymer-based iono-electronics. In this talk, I will share our ongoing efforts in designing novel semiconductors with varying degrees of ion-responsiveness and the scope of applications enabled by molecular tuning. We utilize copolymerization as a facile route to yield a library of mixed conductors enabling a variety of applications ranging from rapidly switching electrochemical transistors to high-fidelity artificial synapses. We have shown that by systematically tethering polar side groups onto known excellent electron conductors, we can probe the contribution from both ionic and electronic conduction to the resulting device performance and determine suitable applications. Lastly, my talk will introduce how we are utilizing these novel conductors to design redox-active nanocomposites combining biocompatibility, electrochemical sensing, and neuromorphic signal processing towards high performance bio-probes.
3.1.-K1
Wulfram Gerstner is Director of the Laboratory of Computational Neuroscience LCN at the EPFL. He studied physics at the universities of Tubingen and Munich and received a PhD from the Technical University of Munich. His research in computational neuroscience concentrates on models of spiking neurons, the dynamics of spiking neural networks and spike-timing dependent plasticity. More recently, he got interested in generalizations of Hebbian learning in the form of multi-factor learning rules and in the role of surprise for learning. He currently has a joint appointment at the School of Life Sciences and the School of Computer and Communications Sciences at the EPFL. He teaches courses for Physicists, Computer Scientists, Mathematicians, and Life Scientists. He is the recipient of the Valentino Braitenberg Award for Computational Neuroscience 2018 and a member of the Academy of Sciences and Literature Mainz (Germany).
The success of Artificial Neural Networks relies on End-to-End training with BackProp, but Biological Neural Networks use local learning rules - and understanding biological rules should also give insight for designing neuromorphic materials and devices. I will review Hebbian two-factor rules as well as their generalization to three-factor rules for action learning [1]. I will then present our recent work on multi-factor learning rules that enabled us to successfully learn representations in networks of up to six layers [2].
The slogan of my biological modeling work is 'No BackProp, Please!' - and we can discuss after the talk whether this should also be slogan for Neuromorphic Materials and Devices.
[2] B. Illing, J. Ventura, G. Bellec, and W. Gerstner (2021)
Local plasticity rules can learn deep representations using self-supervised contrastive predictions
35th Conference on Neural Information Processing Systems (NeurIPS 2021)
3.1.-I1
Neuromorphic systems achieve high energy efficiency by computing with spikes, in a brain-inspired way. However, finding spike-based learning algorithms that can be implemented within the local constraints of neuromorphic systems, while achieving high accuracy, remains a formidable challenge. Equilibrium Propagation is a hardware-friendly counterpart of backpropagation which only involves spatially local computations and applies to recurrent neural networks with static inputs. So far, hardware-oriented studies of Equilibrium Propagation focused on rate-based networks. In this work, we develop a spiking neural network algorithm called EqSpike, compatible with neuromorphic systems, which learns by Equilibrium Propagation. Through simulations, we obtain a test recognition accuracy of 96.9% on MNIST, similar to rate-based Equilibrium Propagation, and comparing favourably to alternative learning techniques for spiking neural networks. We show that EqSpike implemented in silicon neuromorphic technology could reduce the energy consumption of inference by up to three orders of magnitude and training by up to two orders of magnitude compared to GPUs. Finally, we also show that during learning, EqSpike weight updates exhibit a form of Spike Timing Dependent Plasticity, highlighting a possible connection with biology.
3.1.-I2
Dr Brett Kagan is the Chief Scientific Officer at Cortical Labs, a Melbourne based start-up committed to building a new generation of Synthetic Biological Intelligence by integrating living brain cells with silicon and leveraging their computational power. Dr Kagan has previously completed a PhD at the Florey Institute through the University of Melbourne in stem cell therapy for neonatal brain injury. Prior to this, he has conducted research with a medical education consortium focusing on primary health care objectives and received numerous grants and awards supporting this work. Before joining Cortical Labs, Dr Kagan worked as a postdoctoral research fellow at the Australian Regenerative Medicine Institute and engaged in scientific and regulatory affairs consulting work in the biotechnology and nutraceutical industries. Dr Kagan has a strong interest in the fundamentals of intelligence along with the philosophical and scientific interface which drives investigations in this area. He is a strong advocate for improving general scientific literacy and the involvement of empirical evidence in policy development.
The dream of building a neural inspired platform that offers the adaptability of biology, coupled with the reproducibility of traditional hardware, has made considerable progress in the neuromorphic field[1]. Yet, substantial barriers remain that will require new perspectives and approaches to overcome. Revisiting the basics of how biological neurons behave may offer a window to achieve these next steps. Framing modern neuroscientific understandings in the light of recent breakthroughs in developing Synthetic Biological Intelligence (SBI) offers one pathway to progress. Recent work[2] by our group has enabled a monolayer of neurons to dynamically adapt behavior in a goal-directed manner in simulated game-worlds - such as a simplified version of pong. It was found that neural cells from both human and mouse sources could be embodied using electrophysiological recording and stimulation to form a real-time closed loop environment. Implications from the Free Energy Principle were tested to determine whether these neurons would display a rudimentary form of intelligence to show learning by improving at the game. Extending beyond basic performance, the underlying electrophysiological network activity changes that occurred provides insight into the fundamental features that drive intelligence in neural systems. Coupled with the high sample efficiency and network wide adaptability of these living neurons, this data provides compelling implications that may be useful in future neuromorphic engineering efforts.
3.2.-T1
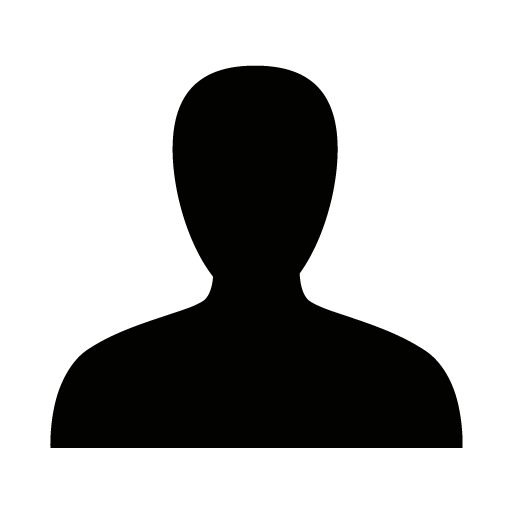
Nanowire networks (NWNs) mimic the brain’s neuro-synaptic connectivity and emergent dynamics. Consequently, NWNs may also emulate the synaptic processes that enable higher-order cognitive functions such as learning and memory. Conductive filaments in NWNs form and decay at a different rate, enabling memory for previously activated junctions and pathways within the network. Here, we exploit this behaviour to train selective network pathways via a learning mechanism inspired by supervised learning in the brain. Using gradient descent to adjust voltages and current outputs of output drain electrodes, we train NWNs to associate inputs with a target drain electrode. Capitalizing on filament decay, we then test the network’s working memory performance on a simple cognitive task. Findings demonstrate the ability of NWNs to recall previously trained pathways, even after a series of interference patterns are presented to the network.
3.2.-T2
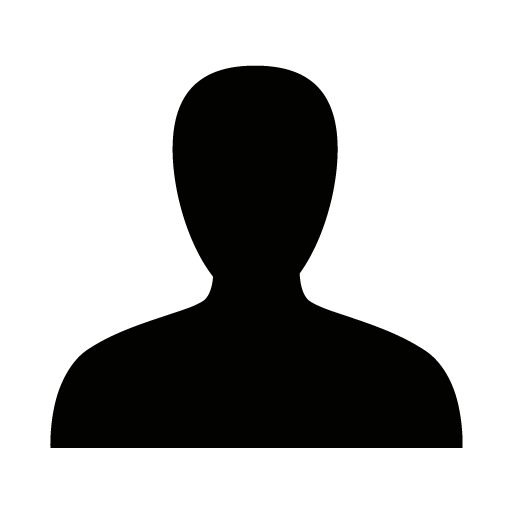
Metal halide perovskites, with their superior electronic properties, solution processibility and scalability, are promising candidates for optoelectronic applications such as solar cells and LEDs. Although of relevance to thin film transistor applications, for perovskite systems, TFT operation at room temperature is primarily achieved via precise compositional, microstructural control or via surface/substrate/electrode treatments. Here, tin doped indium oxide is utilized as source/drain contacts to fabricate a room temperature operational n-type solution-processed Cs(MAFA)Pb(BrI) transistor. The inherent photo response of the perovskite channel and the gate bias modulation capability of the transistor configuration is utilized to demonstrate optical learning in these TFTs. By modulating the gate bias polarity, to boost or suppress the channel conductance, gradual or immediate forgetting of optical learning is achieved. Such TFT configuration and opto/electrical learning/forgetting schemes opens an avenue to employ perovskites as optical learning pixels
3.2.-T3
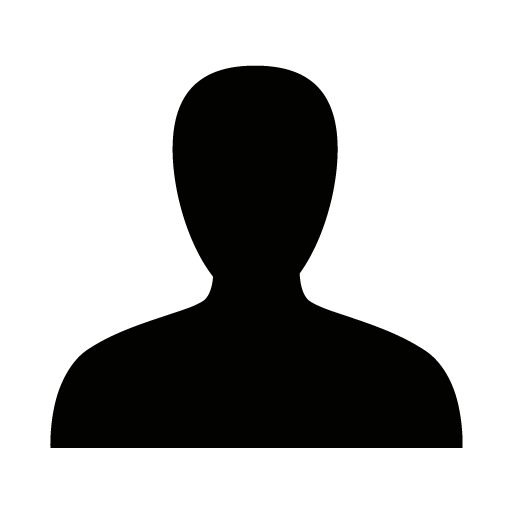
The switching kinetics and electrical behavior of halide perovskite memristive devices, which are based on ion migration with low activation energy, are similar to those of biological synapses, making these devices one potential candidates for brain-inspired computing. Here we report lead free zero dimensional Cs3Bi2I9 perovskite thin films as an active switching material in flexible crossbar memory devices, which exhibits typical bipolar resistive switching (RS) characteristics with high on/off ratio (106), high endurance (104 cycles), long retention (105s) and device yield of more than 93%. In addition, electrical pulse engineering on devices exhibits several synaptic characteristics along with paired pulse facilitation (PPF), long-term potentiation (LTP) / long-term depression (LTD) characteristics with non-linearity of 0.7 /1.2 for 50 conducting states was obtained. In particular, extremely low cycle-to-cycle variations (2.3%) during long-term potentiation and depression processes over 55000 pulses, low devices- device variation are achieved. Thus, a high classification accuracy of 92% is demonstrated in the Modified National Institute of Standards and Technology (MNIST) simulations. This work emphasizes the prospect of zero dimensional Cs3Bi2I9 perovskite thin-films toward designing of next-generation high-performance analog neural networks (ANN).
3.2.-T4
This presentation will present a spiking neural network accelerator made using fully open-source EDA tools, process design kit (PDK), and memory macros synthesized using OpenRAM. The chip is taped out in the 130 nm SkyWater process and integrates over 1 million synaptic weights, and offers a reprogrammable architecture.
It operates at a clock speed of 40 MHz, a supply of 1.8 V, uses a PicoRV32 core for control, and occupies an area of 33.3 mm2. The throughput of the accelerator is 48,262 images per second with a wallclock time of 20.72 µs. The spiking neurons use hysteresis to provide an adaptive threshold (i.e., a Schmitt trigger) which can reduce state instability.
This results in high performing SNNs across a range of benchmarks that remain competitive with state-of-the-art, full precision SNNs.
3.2.-T5
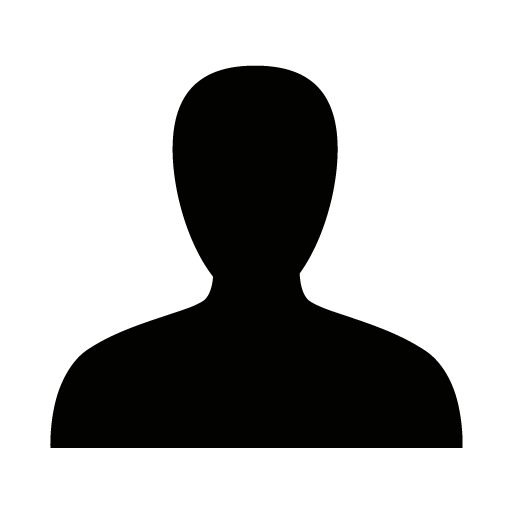
The resistance state of filamentary memristors is tuned by relocating only a few atoms at interatomic distances in the active region of the conducting filament. Thereby the technology holds great promises not only in its ultimate downscaling potential and energy efficiency but also in unprecedented operation speed. In spite of an ongoing interest and recent achievements demonstrating sub-100 ps resistive switching, the breakthrough toward telecommunication frequency applications still lacks the clarification of the dominant mechanisms and inherent limitations of ultra-fast resistive switching.
By utilizing current state of the art electronics, we present the current world record resistive switching speed and multilevel programming in tantalum-pentoxide based memristors due to 20 ps long set and reset voltage pulses, confirmed by the evaluation of the steady states before and after the programming pulses. More importantly, we move beyond the common approach of verifying the effect of the programming pulses in the established steady state only: We have developed an optimized sample design which enabled us, for the first time, to monitor the dynamics of the resistive switching also during the ultra-short programming voltage pulses. Thereby set times well below 20 ps pulse durations could be measured at picosecond resolution. A thermal delay of the reset transition, which exceeds the reset voltage pulse duration, was discovered and the electric field induced and diffusion driven resistive switching mechanisms were experimentally separated [1].
Our results underline the importance of the thermal optimization of atomic scale resistive switches for applications requiring fast cyclic operation. This enables the integration of valence change based filamentary resistive switches as fast and low-power components into state-of-the-art telecommunication technologies.
3.2.-I1
Memristive crossbar arrays are promising non-von Neumann computing technologies to enable real-world, online
learning in neural networks. However, their deployment to real-world learning problems is hindered by their non-linearities
in conductance updates, variation during operation, fabrication mismatch and the realities of gradient descent training. In this
work, we show that, with a phenomenological model of the device and bi-level optimization, it is possible to pre-train the neural
network to be largely insensitive to such non-idealities on learning tasks. We demonstrate this effect using Model Agnostic Meta Learning (MAML) and a differentiable model of the conductance update on the Omniglot few-shot learning task. Since pre-training is a necessary procedure for any on-line learning scenario at the edge, our results may pave the way towards real-world applications of memristive devices without significant adaption overhead.
3.3.-T1
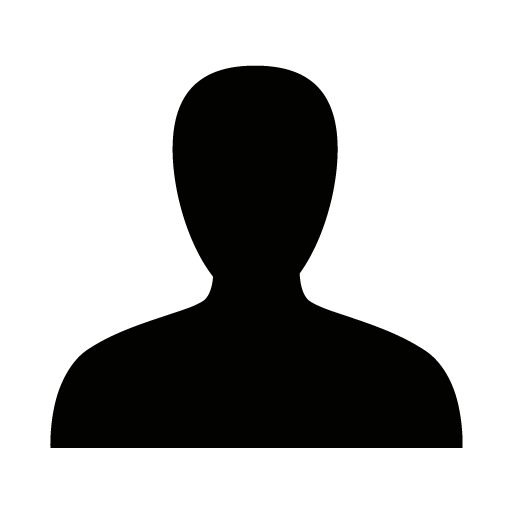
Memristive crossbar arrays are built using scalable in-memory computing technologies. As such, these arrays can solve the von-Neumann bottleneck, which is the dominant factor of the energy consumption of neural network computations. They enable the deployment of large network models on compact embedded, mobile, edge devices for ultra-low power real-time inference applications [1].
However, in many scenarios, the post-deployment conditions might require a further adaptation in the network weights to compensate for domain data shifts due to sensory or environmental noise, gradual device degradation or simply fine-tuning to a new task that has not been seen during the offline training. The analog hardware non-idealities such as programming variability and non-linearity of conductance updates, the high power consumption of programming pulses, and the limited device endurance hinders the possibility of having power-efficient on-chip edge adaptation. This becomes further complicated with the data and memory- intensive requirements of gradient-based learning, such as training in batches and the lack of online and local credit assignment solutions.
In this work, we demonstrate that bi-level optimization offers an offline training solution to overcome the impact of such non-idealities for online learning on edge. We use Model-Agnostic Meta-Learning (MAML) [3] to pre-train a feedforward spiking neural network with accurate memristive synapse models to perform few-shot learning on standard regression benchmarks. Through the differentiable stochastic device model, the outer optimization loop learns a good initialization of the memristive conductances for the deployement that the negative impact of device programming during inner loop updates on the edge is suppressed. To achieve this, we use an empirical model of phase-change material (PCM) [2], which comprises all major forms of analog hardware non-idealities based on the measurements from 10,000 devices. The model includes the programming stochasticity and non-linearity as a function of device programming history, 1/f read noise, and temporal conductance drift. We improved the offline outer loop optimization performance by carefully selecting twice- differentiable surrogate spiking function [4] and additional hardware-aware training methods, such as noise-injection with straight-through estimators [5] and update thresholding [6].
Our results showed that a network trained with bi-level optimization can be finetuned to adapt new tasks on the edge by applying single blind programming pulses to only the output layer memristors of the neural network. We report competitive accuracies thanks to learning to mitigate write noise, read noise and drift of phase-change memory devices.
3.3.-T2
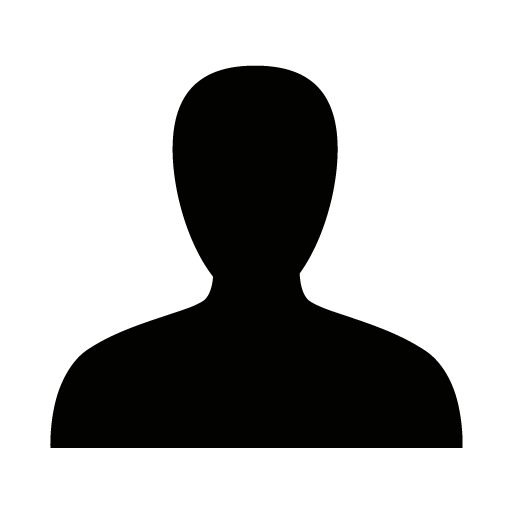
In-memory computing (IMC) has gained traction as the leading candidate for the next computational architecture, overcoming the limitations of the classical von-Neumann computing machines by performing computation directly within the memory unit. Coupled with crosspoint arrays of resistive memories, IMC has potential for unprecedentedly high throughput and energy efficiency [1], by exploiting Kirchhoff and Ohm’s law in an open-loop fashion to perform matrix-vector multiplication (MVM). To alleviate the dependence of open-loop IMC on external digital signal processors, closed-loop, analogue feedback-based structures have been proposed, enabling one-step linear system solution [2] and linear regression [3]. Thanks to its intrinsic parallelism, closed-loop IMC (CL-IMC) can ultimately achieve O(1) complexity [4], vastly improving upon the O(n3) complexity of conventional digital systems. CL-IMC is particularly suited to accelerate next-generation communication networks such as the predicted 6G massive multiple-input multiple-output (MIMO) framework. In MIMO, base stations (BS) equipped with antenna arrays communicate with many users at once, performing heavy matrix-based pre- and post-processing operations both in the uplink, where distortion and noise must be removed from received signals, and in the downlink, where transmitted data must be suitably precoded to maximize throughput [5]. We present a novel CL-IMC circuit for massive MIMO acceleration, suitable for both uplink and downlink processing by reconfiguring input/output pairs [6]. The circuit consists of two memory arrays, each mapping the channel matrix H, feedback-connected by means of two arrays of transimpedance amplifiers (TIAs). By proper tuning of the TIAs transconductances, the circuit can be adapted to work in both low and high signal-to-noise ratio (SNR) regimes. Simulation results show floating-point-comparable accuracy with as low as 5-bit memory elements and 60 dB, 100 MHz amplifiers [6]. Benchmark simulations on large-scale systems show energy and area efficiency improvements by 3 orders of magnitude with the same throughput of a digital system [6], making CL-IMC a suitable candidate for data processing in next-generation broadband cellular networks.
3.3.-I1
The hardware implementation of neural networks is vital to realize power-efficient and fast artificial intelligence (AI) systems for a plethora of applications, e.g., bio-signal classification or real-time monitoring of industrial processes. Following the traditional paradigms of machine learning, efficient hardware implementation of AI systems requires versatile artificial synapses with well-adjustable synaptic strength, also referred to as synaptic plasticity.
However, besides these traditional approaches, it is worth taking a look at the function of biological neural networks as they possess superior properties in terms of power efficiency, fault tolerance, and flexibility. In particular, biological neural networks attain their excellent efficiency from employing specific non-linear properties of the synapses and neurons as well as of the entire system.
In this contribution, I discuss the non-linear properties of organic electrochemical transistors (OECTs), which are in the spotlight of research as they enable the hardware implementation of versatile, low-voltage artificial synapses. The non-linear properties are based on the complex interaction between polarons and ions, which can be manipulated, e.g., by chemical treatment of the semiconducting polymer. I will show how the non-linearity can be quantified and I will demonstrate three approaches to employ it for efficient computations. More specifically, I will discuss how the non-linear response of OECTs can be used to mimic the functions of biological neuron models (spiking neuron), help in decision making using Bayesian inference (stochastic computing), and classify complex bio-signals using reservoir or echo-state computing.
3.3.-I2
Donhee Ham, Ph.D., is Gordon McKay Professor of Harvard University and Samsung Fellow. https://www.donheehamlab.org
Reverse engineering the brain by mimicking the structure and function of biological neuronal networks on a silicon chip was the original goal of neuromorphic engineering, but remains a distant dream. The focus of neuromorphic engineering has thus been eased from the rigorous brain mimicry to brain-inspired design. In this talk, I would like to share an advance in neuroelectronic interface, whose ability to map the biological functional synaptic connectivity may return neuromorphic electronics to its original goal of reverse engineering the brain and may one day create what better approximates the unique computing traits of the brain. Reverse engineering the
3.3.-I3
Ferroelectrics were one of the first materials classes to be proposed as non-volatile semiconducting memories, already 70 years ago. However, until recently, they have struggled to compete in the race towards miniaturization. Luckily, this gloomy prospect has now fully changed thanks to the advent of hafnia-based ferroelectrics, a novel highly polar phase of hafnia that is able to retain the polarization (memory) state down to the nanoscale. The mechanisms that help hafnia to counteract depolarization, which other ferroelectrics are not able to achieve, are not yet fully understood but it is clear that this material has brought ferroelectrics back into the race. In this talk I will present some of our work on ferroelectrics materials for brain-inspired devices, including synaptic behavior of hafnia-based ferroelectrics devices. In addition, I will show our progress towards the use of ferroelectric domain walls as nanoscale memristive networks.
Details of this work can be found at Y. Wei et al. Nature Mat. 17, 1095 (2018), Physical Review Applied 12, 031001 (2019), npj Quantum Materials 4, 62 (2019), Neuromorph. Comput. Eng. 2 044007 (2022), J. L. Rieck et al. Adv. Intellig. Systems, https://doi.org/10.1002/aisy.202200292
3.4.-I1
Machine Learning applications, especially Deep Neural Networks (DNNs) have seen ubiquitous use in computer vision, speech recognition, and robotics. However, the growing complexity of DNN models have necessitated efficient hardware implementations. The key compute primitives of DNNs are matrix vector multiplications which leads to significant data movement between memory and processing units in today’s von Neumann systems. A promising alternative would be co-locating memory and processing elements, which can be further extended to performing computations inside the memory itself. We believe in-memory computing is a propitious candidate for future DNN accelerators since it mitigates the memory wall bottleneck. In this talk, I will discuss various in-memory computing primitives in both CMOS and emerging non-volatile memory (NVM) technologies. Subsequently, I will describe how such primitives can be incorporated in stand-alone machine learning accelerator architectures. Finally, I will focus on the challenges associated with designing such in-memory computing accelerators, and explore future opportunities.
3.4.-K1
Challenges to meet today’s computing needs include the slowing down of technology scaling and the exponential growth of data that need to be processed. A promising alternative approach consists in performing computing based on intrinsic device dynamics, such that each device functionally replaces expensive circuits, leading to adaptive ‘complex computing’. In this talk, I will present a few examples of how material properties can enable device dynamics, and how internal device dynamics can lead to interesting network dynamics and potential applications. These native dynamics at the device and material levels can enable new computing architectures, such as brain- inspired neuromorphic systems, which offer both high energy efficiency and high computing capacity.
3.4.-K2
Stochastic neural networks have become the state-of-the-art approach for solving problems in machine learning, information theory, and statistics. The critical operation in such networks is a stochastic dot product. While there have been many demonstrations of dot-product circuits and, separately, stochastic neurons, the efficient hardware implementation combining both functionalities is still missing. In my talk, I will discuss our recent work [1], [2], [3] addressing this need. I will start with a discussion of compact, fast, energy-efficient, and scalable stochastic dot-product circuits based on passively integrated metal-oxide memristors. The high performance of such circuits is due to mixed-signal implementation enabling energy-saving in-memory computing. The stochastic functionality is achieved by operating the circuit in a low signal-to-noise ratio regime by utilizing the circuit’s intrinsic and/or extrinsic noise to the memory cell array. I will then discuss several application demonstrations based on passively integrated TiO2-x memristors, including Hopfield networks for solving optimization problems and a Boltzmann machine. In the proposed circuits, the neuron outputs can be selectively scaled, which allows adjusting coupling between the neurons and/or controlling the signal-to-noise ratio at runtime without the need to rewrite the memory weights. This feature enables, for example, efficient implementation of different annealing approaches that improve the solution quality for the solved optimization problems. I will conclude my talk with a comparison of the proposed approach to other solutions based on conventional and emerging technologies and a discussion of the important future work.